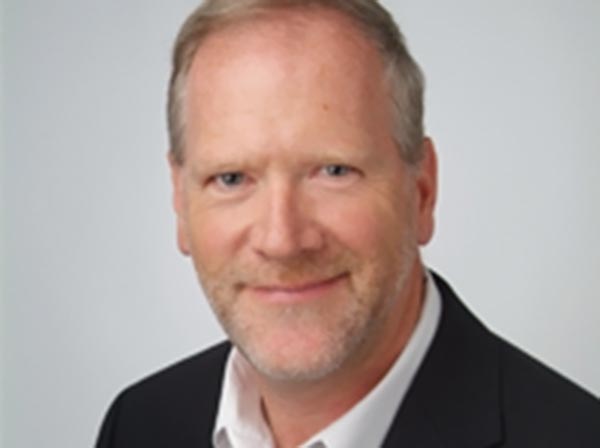
Click to learn more about author Neil McGovern.
Many enterprises are still finessing their data strategies, and more often than not organizations are found comparing data warehouses and data lakes as standalone solutions. To that I say, why not do both? According to PwC’s recent data optimization survey, the top data challenges in the enterprise include poor data reliability, inability to adequately protect and secure data, data siloing or lack of sharing, and inadequate IT systems to support AI. With business decisions becoming increasingly more data-driven there are large amounts of data that needs to be stored, but simultaneously accessed in real time to make those necessary decisions. The business incentive to find a solution to these data challenges includes serious cost savings and boosted annual revenue. According to PwC, securing your data, mending the silos and having proper information systems in place, and increasing the data usage can increase annual revenue by 10% and could save a company 33%.
To have the most effective data strategy across organizations and business processes and to solve today’s Big Data challenges, IT departments should begin to change their mindset from comparing data lakes and warehouses to combining their powers and combing those powers in the cloud.
Breaking It Down: Data Lake vs. Data Warehouse
To understand why a hybrid data warehousing strategy is highly effective, let’s first break down each solution. A data lake is a large quantity of structured and unstructured data from multiple sources that can be efficiently and cost effectively stored. The value of a data lake is that it can deliver insights from data that otherwise would be too large (e.g., 25 years of historical financial data) or data that does not currently have a pre-defined purpose. This allows organizations to get insights and value from previously siloed data that are not possible with the current Data Management practices.
A data warehouse is a store to hold large quantities of data that has been prepared to deliver insight into key business questions. Data warehouses aggregate data from multiple sources and the data and infrastructure are optimized for analytics. The key to future success with both data warehouses and data lakes is to ensure that they are part of a larger combined vision for analytics that can be deployed on-premises and/or in the cloud. They also need to be integrated into a common data integration, movement, and lifecycle infrastructure.
Data warehouses have proven to enhance and improve decision making in organizations by marshalling key data that is structured, cleansed, and complete. A data warehouse aims for efficiency by limiting the data stored to the minimum required to address pre-existing areas of analysis. Data warehouses are able to deliver responses on up-to-date data very quickly and are key to real-time organizations. A data warehouse is a key component of an Intelligent Enterprise and is vital to decision making.
So, What’s the Difference?
Data lakes are more focused on capturing any and all data, often in a raw state, even though the use and value of that data may not be apparent at the time of capture. A data lake allows an organization to investigate areas of analysis that are beyond the boundaries of the data set stored in a data warehouse. Data lakes tend to have slower response times than data warehouses because of the large, amorphous nature of their data sets. Data lakes are valuable to store large historical data sets and can be an archive for a data warehouse. Data lakes can also stage raw data that is in the process of being prepared for import into a data warehouse.
Both data warehouses and data lakes need to be able to increase the value of data to organizations by increasing Data Quality (and thus the trust users have in their data). They need to interact to ensure that they can connect to all data sources to deliver a comprehensive view of data across and even beyond an organization, and they need to bring advanced analytical tools to deliver intelligent insights from the data.
Putting the Data into Action
The best use cases for data warehouses are answering operational questions about an organization, such as revenue for the month to date, which customer bought most of a product in the last 30 days, etc. Data warehouses are also being integrated into business decision-making processes to optimize supply chains, improve customer response times, and increase the accuracy and speed of decision making. Data warehouses are key to delivering real-time analytics as they can continually load the latest, freshest data and respond to queries on that data in near real time.
Data lakes have operational uses, such as storing historical data, or acting as a staging area for data being prepared for a data warehouse. However, the unique value that data lakes can provide is insights from data that is not usually collected in a data warehouse. Data lakes can integrate those insights with more traditional data analytics to deliver answers not possible with traditional data warehouses. Data lakes are often the place for the large data sets that are processed by machine learning and artificial intelligence systems that look for hidden insights into an organization. It is with data lakes that we solve many of the data challenges around not having IT departments that are able to support AI initiatives. Data lakes give organizations the opportunity to examine how they want to utilize the data and to ensure it is being accessed in future scenarios to report on how well they are doing in a market or help to drive the best customer experiences by abiding by all data privacy regulations.
The Hybrid Approach
Of course, one of the better use cases for data lakes and data warehouses is when you can implement both, allowing the data to be in a harmonic and compatible state and deploying in the cloud. In the cloud, data warehouses and data lakes go hand in hand. It is here that an enterprise can harness both the powers of data lakes and data warehouses to assess data, determine its use in business processes, and then utilize it in operations to cut costs and increase revenue. Once these systems are in place it is also possible to automate these actions. No matter the cloud provider, this approach allows an organization to possess the unlimited low-cost storage and flexibility of a data lake, together with the high performance and analytical capabilities of a data warehouse. With the move of a company’s data infrastructure to the cloud, the comparison of data warehouse vs. data lake is dwindling. It is becoming natural for organizations to have both and move data flexibly from lakes to warehouses to enable efficient and real-time business analysis.
There is a lot of innovative work being done to logically bind the data warehouse and the data lake together into a single, fully integrated framework. This will provide the underlying Data Management engine with semantic understanding that can help blend the “understood” structured data in the data warehouse with the “unmined” unstructured data in the data lake. This will ultimately offer up an invaluable 360-degree view of the subject(s) being analyzed (e.g., customers), helping drive unforeseen business value and outcomes.