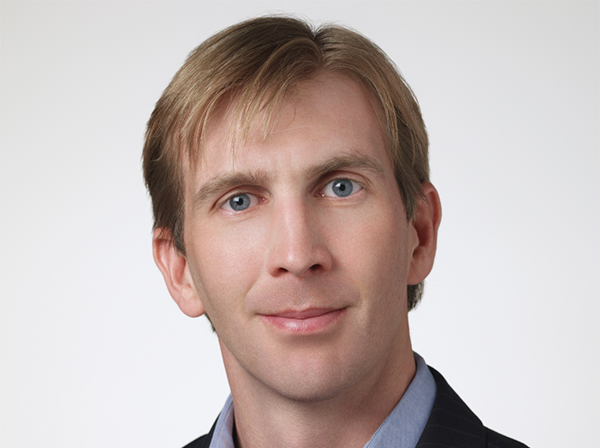
Organizations have long struggled with the “eternal data problem” – that is, how to collect, store, and manage the massive amount of data their businesses generate. This problem will become more complex as organizations adopt new resource-intensive technologies like AI and generate even more data. By 2025, the IDC expects worldwide data to reach 175 zettabytes, more than double our current threshold.
In all this noise, AI stands out as a viable option for automating pattern and trend identification. However, despite the hubbub surrounding innovative AI applications like ChatGPT and Bard, businesses must proceed cautiously. Data is not inherently decipherable, even by the most advanced AI algorithms, and hiccups can cause disaster. For example, imagine a bank using AI to match and merge names without deterministic rules outlined and audited – an error in this instance could have severe ramifications for the bank’s reputation and compliance status.
When organizations deploy AI at an enterprise scale, they take enterprise-level risks. As such, before taking drastic action, leaders must conduct due diligence on their systems to ensure their advanced algorithms will operate on the most current and high-quality information. The secret to doing so? A comprehensive data synthesis and management strategy, which can be realized through master data management (MDM).
Data Deluge Is an Enterprise-Wide Problem
We live in a digital era wherein modern enterprises generate more data than ever. Various commonplace interactions – including customer transactions and social media activity – create sprawling data reserves. Additionally, mid-size and large businesses must aggregate data generated across multiple locations. Even small organizations must heed the data sprawl created by their remote or hybrid workforce.
But it’s not enough to generate this data – systems must also understand it.
However, many businesses don’t use their data effectively because their tools, skills, or resources are limited. Leaders can identify inadequately managed data by conducting an audit that targets the following common issues:
- Unsustainable storage practices: As data generation grows exponentially, IT and data leaders will struggle to store and process it.
- Too many sources and tools: Many organizations address data disarray by adopting new technologies. However, many of these tools generate more data instead of analyzing and storing data, leading to further chaos and informational silos.
- Low trust: When data is not validated, it’s difficult to trust its integrity.
Unfortunately, leaders without a proper data management strategy are missing out. Big data provides critical insights. For example, consumer-facing data can communicate information about customer behavior, while logistics data can help improve operational efficiency and develop new products and services.
AI Cannot Solve Data Disarray
Many experts herald generative AI (GenAI) as the bridge into a new era of work. Certainly, GenAI will change how employees interact with their systems and accomplish tasks. According to a National Bureau of Economic Research (NBER) study, GenAI integrations contribute to a 14% productivity boost among customer support agents, with low-skilled employees receiving the most significant productivity gains. Other professionals in the information economy have seen similar boosts in productivity thanks to GenAI.
Yet Data Quality severely limits the potential of GenAI. AI cannot properly interpret, categorize, or understand data reserves if the hosting organization has no management strategy. Why? Because leading AI tools, including GenAI, subsist on data to contextualize their environment; without the right information to pull from, these advanced technologies are useless. In fact, depending on the use case, AI tools relying on disorganized data can even be detrimental to business outcomes.
To illustrate, let’s return to our earlier example about a bank using GenAI to synthesize its customers’ records. This mid-size bank has one million customers and relies on a manual data management system. An IT leader tasks a new GenAI integration to consolidate duplicative customer data as they look to streamline their organization’s cloud blueprint.
During this process, the GenAI integration identifies several customers named Robert Smith and suggests a way to combine all Robert Smiths without an active address on file. Because the bank implements this integration rapidly and without proper training, these erroneous merges are approved by human moderators. Now, the GenAI has absorbed misleading guidance about when and why to merge customer information – meaning this error will be committed in perpetuity, at least, until flagged by a system moderator and addressed.
This simplified example demonstrates the problem leaders will face if they use GenAI to drive down data disorganization: AI cannot create context. AI systems require human oversight and robust guidelines on which to operate. Without data in its correct place and format, AI systems will generate inaccurate outputs, creating headaches for human moderators and hampering progress.
MDM Is a Long-Term Solution for a Long-Term Problem
MDM systems are the perfect partner for GenAI because these systems restore data integrity, providing AI with the prior knowledge it needs to generate correct outputs. In our banking example, MDM could have cleansed, analyzed, and sorted the bank’s data before GenAI integration. With a comprehensive view of customer data, GenAI could focus on creating more useful outputs, such as providing insights into customer behavior or identifying candidates for special rates and offers.
Understandably, leaders across all industries are excited by the possibilities of GenAI. However, becoming a data-driven organization is not a one-and-done process, and there are no fast lanes toward proper data organization and governance. Perhaps the only expedited way to adopt GenAI effectively is to review MDM solutions and adopt a tool with an immense time-to-value proposition. Otherwise, leaders will likely take a shortcut that will cost them – and their organization – big.