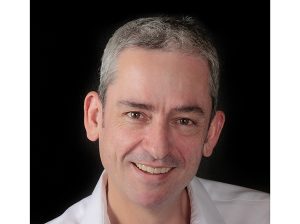
If you’ve been keeping an eye on the evolution of generative AI (GenAI) technology recently, you’re likely familiar with its core concepts: how GenAI models function, the art of crafting prompts, and the types of data GenAI models rely on.
While these fundamental components within GenAI remain constant, the way they’re applied is transforming. The approach to GenAI that captured the spotlight with ChatGPT’s rise in late 2022 is unlikely to be the same approach enterprises will embrace as they leverage GenAI to enable new business capabilities.
Let’s dive into how these concepts surrounding generative AI are evolving and what this change means for the future of GenAI in the business world.
Traditional Approaches to Generative AI
At its core, the fundamental elements of GenAI can be summarized as:
- Foundation Models: A foundation model is a GenAI model that can support a wide variety of uses. To date, a handful of GenAI models – such as the GPT models developed by OpenAI – have dominated this space.
- Data: Models rely on data for training purposes. Traditionally, that data took the form mostly of unstructured data, which the vendors of foundation models – such as, again, OpenAI – used to pretrain their models before making them available to the public.
- Prompt Engineering: Prompt engineering is the process of devising prompts that will produce the appropriate response from a GenAI model. Since the use of pretrained foundation models doesn’t provide much opportunity for customizing the data or context of those models, prompt engineering has been crucial for getting AI models to support certain use cases. You can’t control the data or the model itself, but you can control the prompts you feed into it.
In short, the GenAI ecosystem for the past year and a half or so has been dominated by third-party foundation models, which were pretrained on generic sets of unstructured data, to address use cases that relied heavily on custom prompt engineering. In this world, vendors who built foundation models were essentially the gatekeepers, since their decisions about how the models worked and which data they trained on set the constraints surrounding how models could be used.
Innovations in Enterprise Generative AI
Looking to the future, this approach is poised to change in several key ways.
1. Custom Foundation Models
One of the biggest changes is the increasing availability of foundation models beyond those supplied by companies that specialize in generative AI services.
In addition to open-source models that have been released by companies like Meta and Google, we’re now seeing vendors like SAP developing their own foundation models. Crucially, these models will provide greater opportunity for enterprises to custom-model operations by injecting their own parameters to control the context in which the model operates. In some cases, they can also train or retrain models on custom data.
The bottom line here is that a new generation of foundation models is giving enterprises much more finely tuned control over how they leverage generative AI. They no longer must settle for generic models that were not designed for their particular use cases. They can instead customize model behavior in extensive ways – provided they have the data engineering capabilities to do so.
2. The Use of Structured Data
Historically, GenAI models trained primarily on unstructured data – such as documents and Web pages – because the primary goal of the models’ designers was to allow users to search or summarize data within those documents. Essentially, GenAI models like those developed by OpenAI are alternative search interfaces for the Web.
This remains one important use case for GenAI within business. An additional, emerging use case is to leverage GenAI as an interface for querying structured data – such as information stored in databases – as well. Enterprises can already do this using solutions like Amazon Q.
This is significant because it indicates that GenAI can enhance businesses’ ability to interpret the vast volumes of structured data they possess. In the past, addressing questions based on this data required expert data analysts who wrote complex queries by hand and then generated reports. Now, GenAI can do that work at a rate much faster than even the most skilled data analyst could achieve.
3. The Emergence of Data Dispatchers
Integrating AI models with all the data that exists in a business is a complex task, not least because it is often unclear which dataset is most relevant for a specific use case. For instance, when querying sales data, should the model be prompted using data from the ERP system, the CRM, a manually prepared spreadsheet, or something else?
To tackle this issue, businesses are likely to adopt what I refer to as “data dispatchers.” A data dispatcher is an integration tool that efficiently exposes data to GenAI services in an efficient way, making it easy for enterprises to leverage their data for custom model training. Instead of forcing enterprises to determine which data they need for AI training, they’ll turn to data dispatchers to handle this work.
This places data dispatcher vendors in a position to become the new gatekeepers of the GenAI landscape. The power will shift from vendors who develop AI models to those who influence which data is available to support prompts.
Toward a Democratized, Data-Centric GenAI Landscape?
Ultimately, these shifts – which are already underway – promise to make GenAI more democratic, in the sense that enterprises will have more control over exactly how they use GenAI.
At the same time, they make data – especially proprietary data owned by particular businesses – more important than ever. Rather than being beholden to a handful of AI model vendors and the data they decided to train on, enterprises will get to decide – with help from data dispatchers – which information enables GenAI tools and services.
To thrive in this brave new world, the ability to manage and govern data effectively will be key. Data Management has long been important to enterprises, but if enterprises want to take advantage of emerging opportunities surrounding GenAI, they’ll need unprecedented levels of control over data so that businesses can use it to enable custom GenAI use cases.
That discussion is now being had and will be key to monitor in the months and years ahead.