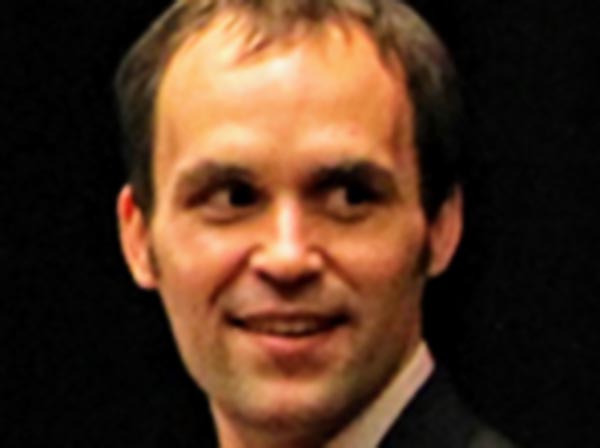
Click to learn more about author Jeff Kinard.
The global predictive maintenance market is anticipated to grow from $3 billion in 2019 to $10.7 billion by 2024, according to Markets and Markets. However, in order for the philosophy to become more pervasive, organizations need to have high-quality data. Businesses are drowning in data, and it must be scrubbed to ensure that decisions, especially predictive maintenance ones, are driven by accurate information. To achieve a single, complete view of data requires aggregation from multiple sources and systems, which has put data quality back in the spotlight.
Trustworthy data is critical for predictive maintenance, especially in heavy industries, where failure can be both costly and potentially catastrophic. For example, if a gas exploration business makes maintenance decisions based on poor data, it could result in a pipeline failing leading to an unplanned outage; the business could incur significant financial losses as a result. Or, depending on the failure, it could impact the safety of workers.
The data deluge is only increasing with automation, machine learning, and AI, with digital transformation fueling the growth. Companies are amassing more and more types of data and the need to quickly and accurately analyze this data has become even more of a priority. IDC predicts that by 2025, nearly 60 percent of the 175 zettabytes of existing data will be created and managed by enterprises. Accurate, reliable, and timely information is vital to effective decision-making. However, the quality of data remains a stumbling block.
So how do organizations deliver an accurate, single view of data?
If companies want to reap the cost, efficiency, and operational gains from predictive maintenance, then it’s essential to go back to basics and make data quality the focus.
There are critical steps to improving data quality:
1. Master Data Governance: Prioritizing good Data Governance is key to helping gather data from multiple systems, including EAM/ERP/CMMS/IIoT, and ensuring that your single source of truth is accurate.
2. Data Silo Elimination: Breaking down silos to gain insights is another critical component to improving data quality. Organizations have access to vast volumes of data; however, they must be able to bring data together from lots of different sources to get a single view of the data. In the case of predictive maintenance, this is mission-critical, and the goal should be to remove all silos.
3. Data Cleansing: Organizations must invest time in data cleansing to maximize the accuracy of data in a system. This requires ensuring that the data is correct, consistent, and usable by identifying any errors and correcting them so they don’t reoccur. This cleansed data then can be used to drive actionable insights.
By following these three steps, organizations will have an accurate, single view of the data necessary to drive predictive maintenance decisions. Quality data drives better decision-making, improved business processes, and greater competitiveness. An organization that makes decisions based on poor quality data is opening the door up to financial and, in the case of many predictive maintenance situations, grave safety consequences.