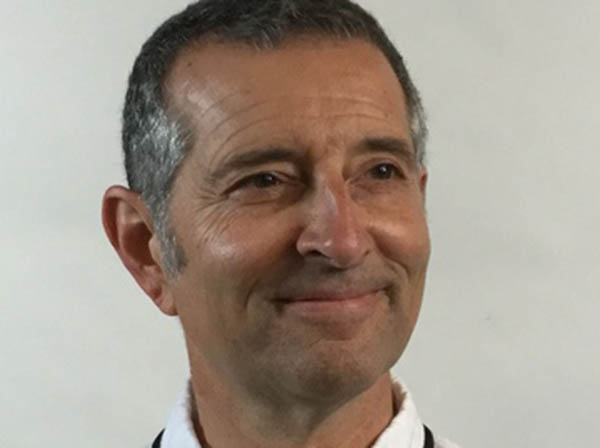
Click to learn more about author Roger Magoulas.
Artificial intelligence has no doubt had a huge impact on business and society as a whole. From healthcare to the military to autonomous cars, AI is paving the way for new applications – all while making our lives easier by reducing human effort and providing accurate intelligence.
As 2020 begins, here’s a quick snapshot of the new developments in automation, hardware, tools, model development, and more that will help shape and accelerate AI as we enter a new decade.
Acceleration of AI Adoption
First and foremost, AI is poised for an acceleration in adoption in 2020 and beyond. This will be driven by more sophisticated AI models being put in production, specialized hardware that increases AI’s capacity to provide quicker results based on larger datasets, simplified tools that democratize access to the entire AI stack, small tools that enable AI on nearly any device, and cloud access to AI tools that allow access to AI resources from anywhere.
Complex business and logic challenges, along with the ability to integrate data from multiple sources and competitive incentives to make data more useful, will combine to elevate AI and automation technologies from optional to a requirement for businesses who want to remain ahead of the competition. For example, as AI processes have enhanced over the years, so too have their unique capabilities to address an increasingly diverse array of automation tasks that defy what traditional procedural logic and programming can handle. This includes image recognition, summarization, labeling, complex monitoring, and response.
In fact, according to a 2019 survey, over half of the respondents say AI (deep learning, specifically) will be part of their future projects and products – and a majority of companies are starting to adopt machine learning.
Blurred Lines Between Data and AI
As every AI practitioner knows, any application of AI is only as good as the quality of data collected. Thankfully, access to the amount of data needed for successful applications, proven use cases for both consumer and enterprise AI, and more-accessible tools for building applications have grown dramatically, which has spurred new AI projects and pilots.
To stay competitive, data scientists will need to at least dabble in machine and deep learning. At the same time, current AI systems rely on data-hungry models, so AI experts will require high-quality data and a secure and efficient data pipeline. As these disciplines merge, data professionals will need a basic understanding of AI, while AI experts will need a foundation in solid data practices and, likely, a more formal commitment to data governance.
New (and Simpler) Tools, Infrastructures, and Hardware
We’re in a highly empirical era for machine learning, meaning that today’s tools for machine learning development need to account for the growing importance of data, experimentation, model search, model deployment, and monitoring. At the same time, managing the various stages of AI development is getting easier with the growing ecosystem of open source frameworks and libraries, cloud platforms, proprietary software tools, and SaaS. Even for companies who are looking to use AI for the first time, options such as drag-and-drop interfaces allow amateurs an easy way to get in on the AI action without typing a single line of code.
The Emergence of New Models and Methods
While deep learning continues to drive a lot of interesting research, most end-to-end solutions are hybrid systems. For example, AlphaGo wasn’t a pure deep learning engine; it incorporated Monte Carlo Tree Search and at least two deep neural networks.
In 2020, we‘ll hear more about the essential role of other components and methods – including Bayesian and other model-based methods, tree search, evolution, knowledge graphs, simulation platforms, and others. We also expect to see new use cases for reinforcement learning emerge. Finally, we might even begin to see exciting developments in machine learning methods that aren’t based on neural networks.
New Applications Driven by New Developments
As Alexa and Echo have shown, technology giants are recognizing interest and demand in voice recognition technologies. Developments in computer vision and speech/voice (“eyes and ears”) technology are helping to drive the creation of new products and services that can make personalized, custom-sized clothing, drive autonomous harvesting robots, or provide the logic for proficient chatbots. Furthermore, work on robotics (“arms and legs”) and autonomous vehicles is coming closer to market.
There’s also a new wave of startups targeting “traditional data” with new AI and automation technologies. This includes text (new NLP and NLU solutions; chatbots), time series and temporal data, transactional data, and logs.
Finally, both traditional enterprise software vendors and startups are rushing to build AI applications that target specific industries or domains. This is in line with findings in a recent McKinsey survey: enterprises are using AI in areas where they’ve already invested in basic analytics.
Fairness and Built-in Biases
Software engineers know to assume that bugs exist in software they develop. Taking a cue from the software quality assurance world, those working on AI models need to assume their data has built-in or systemic bias and other issues related to fairness – and that formal processes are needed to detect, correct, and address those issues.
That said, detecting bias and ensuring fairness doesn’t come easy and is most effective when subject to review and validation from a diverse set of perspectives. AI practitioners will need to build in intentional diversity to the processes used to detect unfairness and bias – cognitive diversity, socioeconomic diversity, cultural diversity, physical diversity – to help improve the process and mitigate the risk of missing something critical.
Retraining the Workforce
As AI tools become easier to use, use cases proliferate and projects are deployed, cross-functional teams are being pulled into AI projects. This means data literacy will be required from employees outside traditional data teams. In fact, Gartner expects that 80% of organizations will start to roll out internal data literacy initiatives to upskill their workforce by 2020.
But training is an ongoing, to succeed in implementing AI and ML, companies will need to take a more holistic approach toward retraining their entire workforce. This may be the most difficult, but most rewarding, process for many organizations to undertake. The opportunity for teams to plug into a broader community on a regular basis to see a wide cross-section of successful AI implementations and solutions is also critical.
Retraining also means rethinking diversity. Reinforcing and expanding on how important diversity is to detecting fairness and bias issues, diversity becomes even more critical for organizations looking to successfully implement truly useful AI models and related technologies. Most AI projects are expected to augment human tasks, meaning that incorporating the human element in a broad, inclusive manner becomes a key factor for widespread acceptance and success.
It’s clear that we’ve made astounding progress in AI over the past decade, which has laid the foundation for some exciting new developments. As we start the new year, only time will tell what new heights we’ll reach with AI at the end of the next ten years.