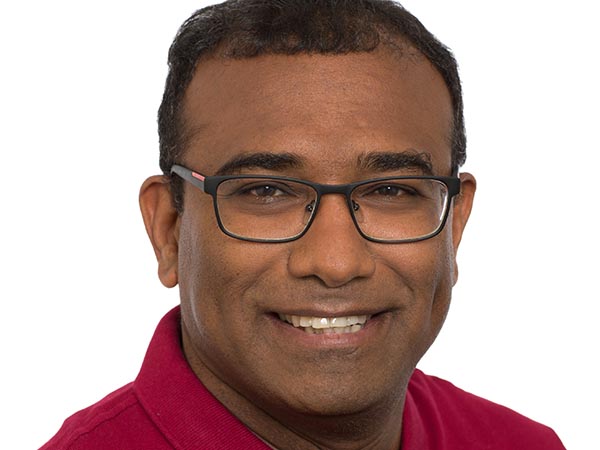
Click to learn more about author Karthik Ramasamy.
Traditional predictive analytics involve leveraging historical data to identify trends, forecast likely events and conditions at a specific time, and model the best action for the desired result. For example, a manufacturer might try to determine when a machine on the production line will fail, or a retailer might recommend a product based on previous purchases.
Essentially, this translates to trying to guess the future based on the past. Why do we do it? Because traditionally it’s all we’ve had the ability to do–with existing approaches, data is already historic by the time it is available for use. Companies attempt to mine value from that historical record in the best way they can: to predict future occurrences. Unfortunately, this creates a slippery slope as companies feel compelled to collect ever more data as the only way to improve the accuracy of their predictions. This not only creates complexity, it inevitably generates a negative feedback loop as the onslaught of data delays insight.
Past Results May Not Predict Future Performance
Any prediction has the potential to provide value, thus leading to heady initial assessments of the power of predictive analytics. The problem is, as organizations seek ever more data to continually refine predictions, they eventually reach an inflection point of decreasing marginal returns. As they handle an ever-growing amount of data, the cost and complexity become overwhelming, and can further lead to data not getting the cleansing and preparation it needs to be useful. In fact, a recent Bain survey found that industrial businesses are finding it increasingly challenging to extract valuable insights from their Big Data.
This chase for ever more data to make marginally better predictions misses a key point: it’s not just the amount of data but – just as or even more importantly – it’s recency that determines predictiveness. If I know what you’re doing right now, I’m in the best position to predict what you’ll do or need next. The faster I can gather and process that data (the “now”), the better my predictive analytics (the “next”).
For example, while a restaurant might rely on historical data to determine the supplies to purchase in advance, they don’t assume that if they can just get the records of what was ordered seven or eight or more years ago, they’ll be able to predict so accurately what customers will want that they can pre-make meals each day and then put them in front of customers without first asking them for their order. Instead, the restaurant optimizes its processes by reacting to data (a customer’s order) as quickly as possible. Not only do they optimize to prepare that order rapidly, they also use the order information to make suggestions about what else the customer might want, to predict when the main course needs to be ready, to know when they should check on the table, and more. For example, when a customer comes into the restaurant and orders a meal, the waiter or waitress can then make predictions about what else they would like based on the current conditions. Did they order pancakes? Maybe they would like a cup of coffee to go with their breakfast too. Predicting who is coming to the restaurant and what they are going to order, and then pre-making everything before the first diner shows up doesn’t make much business sense, and it certainly isn’t efficient.
Yet, across industries, that’s how many people are running their businesses – they build predictions based on what happened in the past and then hope that’s the right thing for the present. Instead of fixating on improving analytics by growing their pool of historical data, what if businesses could instead take advantage of low-hanging and high value immediate data to make better decisions?
Improve Customer Interactions with Recent Data
Relying on the most recent data to make analytics more accurate and predictive is generally less complex than historical-only approaches. Analyzing data as it arrives means focusing on a substantially smaller data set, making the analytics themselves more straightforward. It can also avoid the process and infrastructure involved in collecting, collating, storing and processing vast quantities of historical data for relevant use cases. The result? Improved business interactions based on timely information and faster response.
Take, for instance, Amazon’s approach to ‘fast fashion.’ The company designed an on-demand manufacturing system to quickly produce clothing (and other products) only after a customer’s order is placed. Imagine the implications of on-demand clothing production. Instead of the traditional approach of predictive analytics – guessing the trends that will resonate most with consumers, mass producing clothing to reflect those trends, shipping them to warehouses and stores in advance, and having them sit on store shelves hoping to be sold – retailers can provide exactly what customers want currently, and on-demand. This drives down a number of business costs while improving customer satisfaction and engagement.
Steps to Moving Away from Historical Data Analytics
Incorporating fast data into your analytics means more than just a change to your data process, it means fundamentally rethinking how you interact with users, partners (e.g. supply chains), systems and more. Take partners for example: fast data is a two-way stream, allowing partners to react more quickly to data generated by your company, while you can quickly adapt to the latest data from them.
How do you start down the path to fast data?
Step #1: Rethink How You Process Data
Implementing fast data requires a new perspective on analytics in general, shifting the mindset from “what can I do with even more historical data?” to “what can I do based on current information?” By the same token, applying traditional existing analytical approaches to fast data can often turn out to be counterproductive, as excessively sophisticated and time-consuming analytic model development can make even the fastest data processing slow. If the emphasis is on speed, make sure that carries through to the analytical models.
Step #2: Assess Existing Processes and Models
Fast data and predictions aren’t equally critical everywhere within an organization, as not every business interaction requires an immediate response. The goal should be to focus first on those areas that promise the biggest payoff. One approach might be to start with a broad assessment of existing analytical models within the organization with an eye toward identifying those where a keen understanding of current conditions can improve the accuracy and predictability of a next action.
Step #3: Bring in the Right Technology
Fast data infrastructure requires technology that can transform, process, analyze, and distribute data in motion, and is a lengthy topic of its own (and outside the scope of what we can cover here). But a key consideration toward identifying the right technology is to look for a modern cloud-native approach versus older legacy architectures. Moving to fast data often entails rapid iteration, rapid massive fluctuations in scale (both up and down), integration and reconfiguration without disruption, etc. The right solution will offer the performance, scalability, resilience and flexibility needed to deliver on these requirements.
Step #4: Consider New Opportunities to Benefit from Immediacy
A true fast data mindset can not only change existing predictive analytics, it can uncover totally new opportunities. Business systems and processes throughout an organization rely on data to make decisions every day, from sales and customer interaction to logistics, operational systems, and more. Enabling these systems to make decisions based on current information rather than relying on predetermined outcomes or historical guesswork can drastically improve accuracy and predictability.
Staying Competitive with Fast Data
Every business is setting out to improve customer experiences while at the same time reducing costs and inefficiency. A better understanding of what customers need “in the moment” can enable new offerings and new means of customer engagement.
Collecting and analyzing historical data to predict future events has been the go-to approach for businesses, for the fundamental reason that it’s the best we were able to do. But guessing customer needs based on past outcomes is no longer enough to meet customer expectations or beat the competition. In fact, customer demands are changing every day, every minute, and every second. If businesses aren’t turning to their most recent data first to keep up with these changes, they will quickly fall behind those that are.