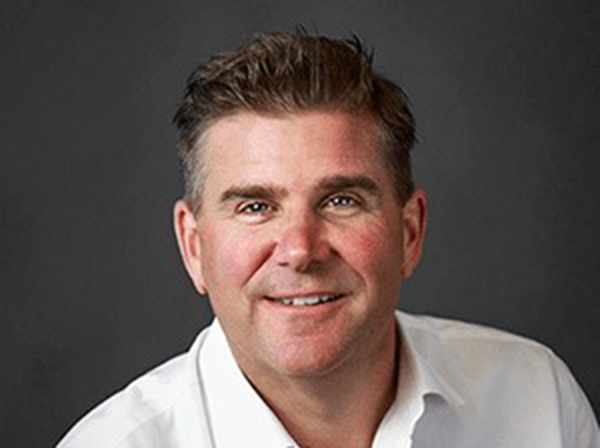
There’s been a lot of talk about semantic layers lately. I’ve seen dozens of companies using a semantic layer to drive self-service analytics at scale. But even with all these success stories, I still get this question: “Is a semantic layer worth the effort?” In other words, is the juice worth the squeeze?
So, how do you know that you need a semantic layer? DBP Institute, a data and analytics research firm, conducted a survey of data leaders and asked them the same question. In the report The Business Impact of Using a Semantic Layer for AI and BI, DBP Institute quantifies the business impact of using a semantic layer for a company’s business intelligence (BI) and artificial intelligence (AI) initiatives.
The results of the study are impressive, as you can see in the chart below:
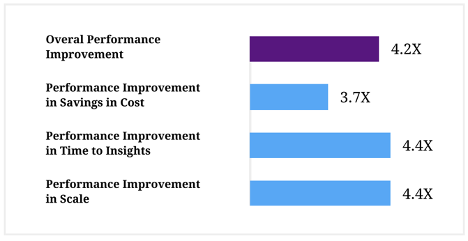
In this article, we will dive into some key takeaways that show how a semantic layer can dramatically improve the speed and scale of analytics delivery while substantially reducing the costs.
Speed
Since the beginning of the data age, it seems that technology and data organizations can never keep up with the data demands of the business. A semantic layer delivers analytics to the business faster, in the following ways:
1. Accelerating Time to Insights
A semantic layer reduces time to insights by connecting analytics consumers directly to business-friendly, analysis-ready data. By bringing “live” data to users, in their preferred tooling and without data extracts or imports, there’s no time wasted moving or reformatting data for analysis.
2. Accelerating Data Preparation Time
A semantic layer reduces or eliminates many data preparation tasks by leveraging data virtualization for transformations. By avoiding physical data transformation using ETL or ELT tools, a semantic layer can create new calculations and clean data without the need to physically persist those transformations.
3. Accelerating Data Modeling
Today, we require end users to model data in their consumption tools before they can create their charts, tables, predictive models or simply analyze their data. With a semantic layer, data stewards create the semantic model on behalf of data consumers so that end users can get right to the business at hand: creating data products.
Scale
Delivering analytics to the business faster is essential, but true data democratization requires scale. A semantic layer can make more data available to more people in the following ways.
1. Expanding self-service
The wide-scale adoption of “self-service” visualization tools delivered many positives by freeing users from the binds of IT. However, in doing so, modern visualization tools like Tableau and Power BI now require their users to become data experts and SQL jockeys – creating “haves” and “have nots” in analytics. A semantic layer makes data accessible to everyone by presenting users with a logical view of data rather than tables and columns in a database. Along with native support for tools like Excel, a semantic layer evens the playing field so anyone can make data-driven decisions.
2. Expanding access to data source
The era of the single, monolithic data warehouse is over. Data lives in many forms, including data lakes, cloud data warehouses, and SaaS applications. A semantic layer hides the complexity of data location and format from users with its logical, business-friendly interface, thereby making more data sources available for analysis.
3. Driving consistent metric definitions
The self-service analytics revolution greatly expanded the universe of analytics creators – a good thing. However, without guardrails, all those creators can destroy trust in data by presenting alternative definitions of common business terms and metrics – a bad thing. A semantic layer brings order to the universe by serving as a central repository for an organization’s business definitions. Now, analytics creators can build data products without reinventing the wheel, driving efficiency and trust in data.
Cost Savings
On the topic of efficiency, a semantic layer can deliver substantial cost savings in a number of ways:
1. Improving ROI
Organizations make substantial investments in building data lakes and warehouses and purchasing all sorts of tools for their business analysts, data scientists, and application developers. A semantic layer can justify these investments by enabling more users and access to more data – essentially, driving more people to make more data-driven decisions.
2. Reducing computing costs
If you look closely at the query patterns of most BI queries, you will find that they are highly correlated. Essentially, users ask the same questions over and over. These repetitive queries can drive up costs dramatically since most cloud data platforms charge customers on a consumption basis (amount of data read). A semantic layer can reduce these costs by orders of magnitude by redirecting queries away from the atomic data tables and to aggregate tables instead. Since the semantic layer “understands” the semantics of each query, it can rewrite queries to find the lowest cost approach at answering the user’s question.
3. Reducing manual labor
Building data pipelines and tuning query performance is a time-consuming and expensive task. Even worse, in a dynamic environment with changing query patterns and data-hungry consumers, it’s a job that’s never quite “done”. A semantic layer drastically reduces or eliminates these manual tasks by replacing physical ETL/ELT tasks with virtualized transformations and by automating query tuning using AI and machine learning.
Summary
As you can see above, a semantic layer can remove friction and make data available to everyone in your organization. By reducing manual labor, a semantic layer can improve an organization’s ability to deliver new data to the business faster and at scale. Not surprisingly, a semantic layer is quickly becoming a critical component in a modern data and analytics stack.