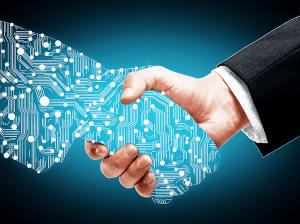
A batch processing system fails on the eve of a company’s deadline for monthly reports, threatening the accuracy of its financials. One of the two systems powering the dashboard of a global supply chain company crashes, and a manager overbooks a transport ship because it displays inaccurate data, causing a costly delay in a customer’s shipment. A simple change of a column name almost leads to more than 1,000 models powering an organization’s dashboards, metrics, and reporting tables to fail at once.
These are three examples of what can happen when data consumers place their trust in data that is not trustworthy. The people in your company who create and maintain the data products that others use in their work are rightly concerned about ensuring the quality of the data. Data consumers also need to be able to trust the data’s accuracy, reliability, timeliness, completeness, and relevance to the task at hand.
At some level, nearly every person in the organization plays some role in both the production and use of data products, but most are primarily data product producers or consumers. Data trust describes the relationship between these two groups: producers working to build trust into the tools they create and maintain, and consumers placing their faith in the trustworthiness of the information.
What Is Data Trust?
Data trust can be seen as data reliability in action. When you’re driving your car, you trust that its speedometer is reliable. A driver who believes his speedometer is inaccurate may alter the car’s speed to compensate unnecessarily. Similarly, analysts who lose faith in the accuracy of the data powering their models may attempt to tweak the models to adjust for anomalies that don’t exist.
Maximizing the value of a company’s data is possible only if the people consuming the data trust the work done by the people developing their data products. (Note that the term “data trust” is used to describe both the faith consumers have in the usefulness of data and a legal entity that serves as an independent third party charged with storing and managing data to facilitate collaboration between organizations.)
The rise of machine learning and other AI technologies heightens the importance of data trust for producers and consumers alike. The efficacy of machine learning models depends entirely on data that is not only accurate, complete, and error-free, but also free of bias, secure, and in compliance with applicable privacy regulations.
Bias-detection tools such as IBM’s AI Fairness 360 and Google’s What-If Tool assist companies in identifying and addressing bias in their machine learning models using such techniques as adversarial debiasing and reweighting, and the ability to evaluate fairness models across demographic groups.
Why Trust Matters
People are generally trusting souls – until they aren’t. Once a person loses their trust in a person, product, or company, regaining that trust is an uphill battle. Between data product producers and consumers is a black box that makes each invisible to the other. Trustworthiness depends on transparency, yet technologies such as self-driving cars and automated decision support ask data consumers to take a leap of faith despite widespread data breaches, failed personalization efforts, and steep declines in service quality.
Deloitte describes data trust as “bridging the gap between knowing and doing.” The company’s 2024 Global Human Capital Trends report found that 88% of the organizations surveyed acknowledge the importance of trust and transparency between data producers and consumers, yet only 52% have begun to act on the matter. The report found that a mere 13% of respondents are reaping the benefits of their efforts, averaging a two-fold increase in desired business outcomes and greater-than-two-fold jump in positive human outcomes.
How to Build Trust in Your Company’s Data
A new generation of products is intended to bridge the data trust gap by building visibility into modern data systems. Most operate by creating a layer of metadata that lets companies trace data elements back to their sources, providing a complete data lineage. Underlying these tools is the notion that since trust in data can be lost in many different ways, restoring that trust necessitates taking a variety of approaches. They include Fivetran’s Metadata API, dbt Lab’s Semantic Layer, and efforts by Soda and dbt, Monte Carlo Data, Metaplane, Astronomer, and OpenLineage.
- Fivetran Metadata API is designed to track data as it moves through pipelines managed by Fivetran. It reports on the source of the data, its impact, which users have access to it, and the effect of upstream schema changes on downstream processes. The tool is pre-integrated with data catalog providers such as Atlan, data.world, Collibra, and Alation.
- dbt Lab’s Semantic Layer allows teams to define data metrics centrally along with their dbt models. The metrics are referenced dynamically from one source to promote consistency in results, which improves self-service for non-technical business users. The system works with a range of data analytics tools, and its data queries can be exported to your data platform for use anywhere.
- Soda-dbt integration combines Soda’s data quality testing product with test results from dbt Labs to add the ability to visualize data quality over time, create an alert system for failed dbt results, and report and track data quality anomalies. The Soda Core open-source Python library and command-line interface (CLI) supports more than 18 data sources and features the Soda Library extension for connecting to Soda Cloud.
- Data observability tools include Monte Carlo Data’s end-to-end field-level data lineage that tracks column-level dependencies from initial ingestion from a data warehouse to dashboards and reports for business intelligence purposes. Similarly, Metaplane’s data observability products feature column-level lineage across the entire data stack that quickly identifies the products impacted by a data anomaly, the effect of raw data on your data infrastructure, and the data quality issues that each stakeholder must address.
- Astronomer and OpenLineage have integrated their products to allow Astronomer’s Astro platform based on Apache Airflow to work with OpenLineage’s open framework for data lineage and data observability. The system automatically gathers and correlates the creation, movement, and transformation of data sets across distributed environments. In addition to tracing the end-to-end transfers of data sets, the solution consolidates quality metrics and identifies potential operational problems.
Keys to Creating a Long-Term Data Trust Program
Understanding the importance of data trust is the first step in implementing a program to build trust between the producers and consumers of the data products your company relies on increasingly for its success.
Once you know the benefits and risks of making data trustworthy, the hard work of determining the best way to realize, measure, and maintain data trust begins. Among the goals of a data trust program are promoting the company’s privacy, security, and ethics policies, including consent management and assessing the risks of sharing data with third parties.
The most crucial aspect of a data trust program is convincing knowledge workers that they can trust AI-based tools. A study released recently by Salesforce found that more than half of the global knowledge workers it surveyed don’t trust the data that’s used to train AI systems, and 56% find it difficult to extract the information they need from AI systems. Of the workers who don’t trust AI training data, three out of four state that the systems don’t have the information they need to be of use.
Data management vendor Atlan presents a seven-step process for building trust in data among your organization’s various stakeholders.
- Start with a data governance framework that defines all roles, procedures, and responsibilities pertaining to data management in the organization. Among the roles are data stewards charged with confirming the quality and availability of data in their departments.
- Plan and implement your company’s data security policies for encrypting data at rest and in transit, controlling access to data based on roles and responsibilities, and protecting data systems with firewalls and intrusion detection systems.
- Prepare a schedule for regular compliance checks that include internal and external audits, documentation of data-handling practices, and remediation plans for items that are determined to be non-compliant.
- Combine automated data quality assurance measures with manual audits that provide a more detailed view of specific aspects of your company’s quality checks. Data cleaning becomes a component of quality checks by automatically updating incorrect or incomplete data.
- Engage stakeholders in data governance procedures by implementing feedback loops for internal and external data consumers. Training programs instruct managers and employees in the importance of data quality, and frequent, timely updates keep them aware of changes in data operations.
- Make sure all data use and storage guidelines are clear, accessible, and up-to-date. Follow all rules for securing employees’ informed consent before collecting or using their private data, and let stakeholders know how, when, and where their data is being applied.
- Continuously monitor your company’s data governance operations using tools that provide real-time reports of data changes, data accesses, and potential problems. Conduct surveys and collect other feedback on a regular basis, and apply iterative improvements based on that feedback.
Building trust in your company’s data from the outset is more effective and affordable than attempting to restore data trust once it has been lost. A proactive approach to instilling quality as the foundation of your data management frameworks promotes loyalty among staff members, facilitates governance, and makes your business function more efficiently. Data trust is ultimately a competitive advantage that benefits your customers and your bottom line.