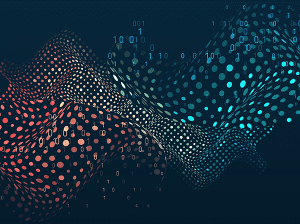
A data mesh challenges the traditional centralized Data Architecture by advocating a distributed and domain-oriented architecture. Data mesh promotes the idea of treating “data as a product,” where each domain or business unit becomes responsible for its own data products. By doing so, individual domains gain autonomy over their data needs and can make faster decisions based on real-time insights.
To facilitate this decentralized approach, data mesh relies on four key principles: domain-oriented decentralized ownership and control of data products, self-serve infrastructure as a platform (IaaP), federated Data Governance model, and discoverability through a self-serve data catalog.
The Domain-Oriented Decentralized Governance Model
The domain-oriented, decentralized governance model is a core aspect of a data mesh. In this model, each domain within an organization takes ownership of its data and becomes responsible for its governance. This means that domain teams have the autonomy to make decisions regarding their specific data needs, such as defining schemas, access controls, and quality standards.
To achieve a decentralized governance model, organizations need to establish clear guidelines and frameworks that enable collaboration and coordination across domains. This includes defining common principles and standards for data interoperability and integration. Additionally, tools and platforms should be provided to support domain teams in managing their data effectively.
It necessitates fostering a culture of trust, transparency, and collaboration between domains. Leadership plays a crucial role in supporting these changes by empowering domain teams to take ownership of their data while providing guidance on overarching organizational goals.
Scalable Data Infrastructure and Autonomous Data Teams
Building a scalable data infrastructure and autonomous data teams remains a critical task while implementing the data mesh. In this decentralized model, the responsibility for data products is distributed among various domain-oriented teams. Each team takes ownership of its own domain’s data and is empowered to make decisions about its storage, processing, and accessibility.
To achieve scalability, organizations need to establish a robust foundation of infrastructure components that support the seamless flow of data across domains. This involves implementing reliable pipelines for ingesting and transforming raw data into consumable formats, as well as building efficient storage solutions that cater to specific domain needs.
Furthermore, enabling autonomous data teams requires establishing clear boundaries between domains and providing them with self-service tools that allow for independent development and deployment of their respective data products.
Cloud-Native Platforms and Advanced Analytics Techniques
Data mesh promotes the idea of leveraging cloud-native platforms and advanced analytics techniques in a federated data ecosystem. In this approach, instead of relying on a centralized data team to manage all aspects of the data pipeline, individual domain teams are empowered to own and manage their own data products.
This means that each team is responsible for collecting, processing, analyzing, and serving their own domain-specific data. Leveraging cloud-native platforms allows teams to take advantage of scalable and elastic resources provided by cloud providers. This enables them to efficiently store and process large volumes of data.
Additionally, advanced analytics techniques such as machine learning algorithms can be utilized to extract valuable insights from the vast amount of collected data. By adopting a federated approach, organizations can break down silos and enable cross-functional collaboration.
6 Key Features of a Data Mesh for Enterprises
Traditional approaches to Data Management have often hindered business agility, resulting in data silos, slow decision-making processes, and missed opportunities. To overcome these challenges, organizations are increasingly turning to a data mesh.
The key features of a data mesh are:
Decentralized and domain-oriented approach: This emerging paradigm recognizes that data is not a centralized asset owned by a single team or department, but rather a distributed resource that belongs to the entire organization.
Data organized around specific business domains rather than technical functions: Each domain team becomes responsible for defining the schema, quality standards, and access policies for their respective datasets. This allows for greater agility as teams can independently make changes without impacting other domains.
Distributed systems and self-serve capabilities: One of the key features of a data mesh for enterprises is its ability to ensure scalability and agility through the use of distributed systems and self-serve capabilities. By adopting a distributed system approach, organizations can leverage the power of multiple interconnected nodes to handle large volumes of data efficiently. Distributed systems allow for parallel processing, enabling organizations to scale their operations as needed without compromising performance.
Moreover, self-serve capabilities empower teams across the organization to access and analyze relevant data independently, reducing dependencies on centralized IT teams. This enables faster decision-making processes as teams can directly access the information they need when they need it.
Collaborative approach to Data Governance and ownership: In traditional centralized Data Architectures, Data Governance is often enforced through strict control mechanisms and hierarchical decision-making processes. However, in a collaborative approach to Data Governance and ownership, the focus shifts toward empowering individual domain teams to take responsibility for their own data. Within a data mesh, each domain team becomes the owner of their respective data products.
This ownership entails not only the technical implementation but also the end-to-end accountability for the quality, reliability, and security of their data. This collaborative approach fosters a sense of shared responsibility among domain teams and promotes transparency and accountability across the organization.
Data accessibility, quality, and real-time insights: By decentralizing data ownership and democratizing access across teams and departments, a data mesh empowers individuals to access and utilize relevant information in real time. This fosters collaboration and agility within an organization, as employees can make informed decisions based on accurate and up-to-date insights. Moreover, a well-implemented data mesh ensures high-quality data throughout the entire organization.
Security, privacy, risk management, and cloud computing in a data mesh environment: Implementing a data mesh framework within an enterprise requires careful consideration of various factors to ensure security, privacy, risk management, and efficient utilization of cloud computing resources. To address security concerns in a data mesh environment, enterprises should implement access controls and encryption mechanisms at multiple layers.
Risk management strategies play a vital role in identifying potential vulnerabilities and mitigating any potential threats.
In Summary
Data mesh fosters cross-functional collaboration and data democratization. By shifting from a centralized model to a decentralized one, the responsibility for data is distributed across different teams or domains, empowering them to have ownership over their own data. This approach eliminates the bottlenecks created by a centralized data team and allows domain experts to make decisions based on their specific business needs.
Self-serve data infrastructure promotes agility, scalability, and improved productivity. By empowering business teams with direct access to data, the need for centralized data teams to act as gatekeepers is eliminated. This self-service model allows teams to retrieve and analyze data independently, reducing reliance on specialized technical resources and streamlining decision-making processes.
The data product thinking approach drives enhanced decision-making and improved customer experience. By treating data as a product, companies shift their focus from simply collecting and storing data to creating valuable insights that can be used to make informed business decisions. This approach involves understanding the needs and preferences of internal stakeholders, such as business users and analysts, and delivering data products that address their specific requirements. Data product thinking also extends to external customers, where organizations can leverage data products to personalize experiences, offer tailored recommendations, and anticipate customer needs.
Real-time analytics capabilities help achieve performance optimization. With real-time analytics, organizations can achieve performance optimization by promptly identifying and addressing bottlenecks or inefficiencies in their operations. By leveraging up-to-the-minute insights, businesses can make data-driven decisions that drive growth and competitive advantage. Ultimately, the integration of real-time analytics capabilities within a data mesh framework enables organizations to harness the power of data for improved performance, innovation, and business success.