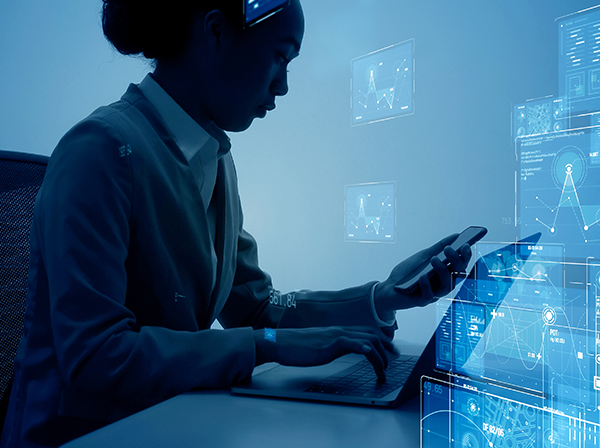
The multifaceted role of a data scientist goes far beyond crunching numbers and analyzing datasets. So, what does a data scientist do? In today’s data-driven world, these professionals are required to possess a diverse range of skills and knowledge to truly excel in their field. While technical expertise in statistics, programming languages, and machine learning algorithms is undoubtedly crucial, it is equally important for data scientists to embrace a broader skill set.
They should be able to translate technical jargon into easily understandable terms while presenting their findings through visualizations or reports. In summary, the essential skills for a data scientist encompass more than just statistical analysis; proficiency in coding and communication are equally vital.
Daily Regimen of Data Scientists: From Statistical Analysis to Coding and Communication
While statistical analysis forms the foundation of a data scientist’s work, proficiency in coding and effective communication are equally important to thrive in this multifaceted role. Data scientists must have a solid understanding of statistical concepts and methodologies to extract meaningful insights from complex datasets.
First and foremost, they must possess the ability to identify relevant questions, design appropriate experiments or analyses, and derive actionable insights from the results. They should be adept at applying statistical models, conducting hypothesis testing, and interpreting results accurately.
Additionally, they need to possess strong programming skills to manipulate and analyze data efficiently. Proficiency in languages like Python or R enables them to clean datasets, build predictive models, and automate tasks.
Another essential aspect of the data scientist’s role is effective communication because these data geeks have to communicate their technical insights and findings to a broad range of business users. Thus, it is imperative that they have convincing storytelling skills to transform raw data into meaningful narratives to guide decision-making.
All the above qualities require critical thinking, creativity, and adaptability. Moreover, data scientists also need a solid understanding of business acumen. They must align their analytical work with organizational goals and strategies, identifying opportunities for growth or improvement.
Mastering the Tools of the Trade: Python, R, SQL, and Hadoop for Data Scientists
Python, with its extensive libraries of powerful tools for data manipulation, data analysis, and machine learning, has established itself as a go-to language for Data Science. Similarly, R programming offers a wide range of statistical techniques and packages specifically designed for data analysis.
Knowledge of structured query language (SQL) is crucial for working with relational databases. Data scientists use SQL to efficiently extract relevant information from vast datasets. They can perform complex queries and aggregations to uncover valuable insights.
As big data continues to grow exponentially, Hadoop remains an integral part of a data scientist’s toolkit. With its distributed processing framework, Hadoop enables handling large-scale datasets across clusters of computers.
Knowledge of these tools helps data scientists clean and preprocess raw data effectively. They can then apply advanced analytics techniques like ML algorithms or predictive modeling to extract valuable insights from the available information in order to make informed decisions that drive business growth.
Data Science Applications: From Predictive Modeling to Social Network Analysis
The field of Data Science encompasses a diverse range of applications that extend far beyond mere numbers and statistics. One key area where data scientists excel is in predictive modeling, which involves using historical data to make informed predictions about future outcomes.
By employing advanced algorithms and machine learning techniques, data scientists can uncover correlations, causations, trends, and patterns within datasets, enabling them to forecast everything from consumer behavior to stock market fluctuations.
Another fascinating domain within the realm of Data Science is social network analysis. This area focuses on understanding the intricate relationships between individuals within a network, be it online or offline. Data scientists employ sophisticated graph theory algorithms to examine how information flows through networks, identify influential nodes or communities, and predict the spread of ideas or diseases.
Data scientists play key roles in healthcare, finance, marketing, and cybersecurity.
The Evolving Role of a Data Scientist
It is evident that data scientists will continue to face new challenges and take on additional responsibilities. One prominent trend is the increasing need for data scientists to be proficient in artificial intelligence (AI) and machine learning (ML). With advancements in technology, organizations are relying on these tools to gain insights from vast amounts of complex data.
Data scientists will need the skills necessary to develop and deploy AI models, as well as accurately interpret their results. Additionally, there is a growing emphasis on ethical considerations within the field. As concerns surrounding privacy and bias continue to rise, data scientists will be expected to navigate these issues responsibly. This includes ensuring that algorithms are fair and unbiased, as well as protecting sensitive information.
Furthermore, collaboration will become an integral part of a data scientist’s role. Working closely with domain experts across different departments within an organization will enable more effective problem-solving and decision-making processes. In conclusion, the role of a data scientist in 2023 will encompass not only technical expertise but also ethical considerations and collaborative skills.
Essential Skills for Data Scientists
Data scientists will continue to be in high demand. To excel in this rapidly evolving field, they must acquire a diverse range of skills that go beyond traditional data analysis.
First, a strong foundation in statistics and mathematics remains essential for understanding the fundamentals of Data Science. Second, proficiency in Python and R is crucial for manipulating and analyzing large datasets efficiently. Third, expertise in ML algorithms is becoming increasingly important for building predictive models.
In addition to these core skills, data scientists should also familiarize themselves with emerging technologies such as deep learning. As organizations collect vast amounts of unstructured data, like images, videos, and text, the ability to extract meaningful patterns and insights from these complex datasets using deep learning techniques will be highly valued.
Finally, effective communication skills are vital for data scientists to convey their findings to non-technical stakeholders clearly. The ability to translate complex analytical results into actionable business recommendations will set apart exceptional data scientists.
The Future: Moving from Data Mining to Data Visualization
As the field of Data Science continues to evolve, so too does its potential to transform industries and revolutionize decision-making processes. While descriptive and predictive analytics have long been utilized to gain insights from historical data and make informed predictions about future outcomes, the current emphasis is on prescriptive analytics.
Prescriptive analytics takes data analysis to a whole new level by not only providing insights into what might happen but also offering recommendations on how to make it happen.
By leveraging advanced algorithms, machine learning techniques, and artificial intelligence, data scientists can now go beyond simply understanding patterns and trends. They can provide actionable suggestions that optimize decision-making processes.
The impact of prescriptive analytics is far-reaching across numerous sectors. For example, in healthcare, it can help physicians determine personalized treatment plans based on patient data. In supply chain management, it can optimize inventory levels and streamline logistics operations. In finance, it can assist with risk management strategies.
In 2023, the role of a data scientist has evolved from merely mining large datasets to effectively visualizing (interactive dashboards, charts, and graphs) and interpreting complex data to enhance decision-making processes.
With the advent of advanced techniques, data scientists are now equipped with powerful tools and methodologies that enable them to extract valuable insights from vast amounts of information. Data mining remains a crucial aspect of a data scientist’s job; however, it is no longer the sole focus.
Image used under license from Shutterstock.com