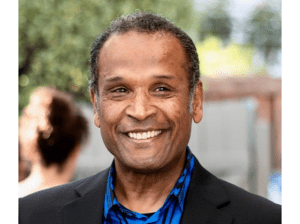
In May, OpenAI announced a partnership with Reddit to train its language models using the forum’s extensive collection of user-generated content.
OpenAI’s goal of enhancing its models’ ability to respond to real-world conversations and diverse linguistic patterns seemed straightforward. But the decision quickly sparked concerns – namely, the potential inclusion of misinformation and biased content in the training data, based on the unregulated nature of Reddit’s online discussions.
This scenario highlights a complex but increasingly common innovation trade-off: the benefits of rich, dynamic, copyright-free data versus the risks of unreliable AI-generated inputs.
The takeaway? To build AI systems that can thrive in real-world environments, IT teams must understand how to balance risks with effective strategies that ensure AI model accuracy, fairness, and resilience.
User-Generated Data Will Shape the Future of AI
The use of unreliable data for AI training may seem counterintuitive, but it’s a key component in helping AI systems become more resilient and adaptable.
By nature, user-generated data (UGD) contains inconsistencies, biases, and inaccuracies, which mirror the real-world complexities AI models must learn to navigate. Training on a diverse range of clean and noisy data means AI models can grow more effective at generalizing across various languages, cultures, and scenarios, making their outputs more relevant and effective.
Incorporating unreliable data from sources like Reddit also helps AI systems build resilience. Exposing models to noise trains them to filter and prioritize high-quality information over weaker inputs, improving their ability to operate in environments where data quality is inconsistent. This capability is especially important for AI systems deployed in dynamic settings like real-time interactions or fast-changing data streams, where clean, structured data isn’t always available.
Moreover, using potentially biased or noisy data can help AI models recognize and reduce bias in their outputs. It may seem counterintuitive at first, but when combined with human feedback loops, models can learn to safely detect bias in real-world interactions. That type of validation process helps AI insights continuously improve.
Human input remains critical for identifying where AI models perform well and where they fall short. Fine-tuning based on human insight also leads to improved accuracy and fairness in outputs over time. But this learning process can be accelerated when large-scale sets of UGD are included.
5 Strategies to Safely Incorporate UGD Into AI Models
The successful integration of UGD into AI models requires a strategic balance between leveraging UGD’s benefits and mitigating its known risks. As with any emerging solution, IT teams play a vital role in ensuring AI systems remain accurate and adaptable despite the challenges posed by beneficial, yet noisy or unreliable data sources.
Consider the following strategies to help harness the power of UGD in your own AI applications:
- Conduct data preprocessing and cleaning
Before incorporating UGD into your AI models, ensure the data is thoroughly preprocessed and cleaned. Automated tools can help you detect, filter, and remove irrelevant, toxic, or inaccurate data, which protects your models from being influenced by poor-quality information – boosting reliability while decreasing the threat of misinformation.
This prep work offers the additional benefit of protecting data privacy. Anonymizing or removing personally identifiable information (PII) before feeding data into your AI system can help ensure compliance with relevant regulations and safeguard the privacy of end users.
- Implement bias monitoring and detection
UGD often introduces bias into AI models, so you need a system to handle ongoing bias monitoring. Regular audits of your AI outputs can reveal emerging biases and identify performance drift over time.
With these insights, you can make real-time corrections like rebalancing datasets or applying algorithms within your database to address and minimize bias, ensuring your models remain as fair and accurate as possible.
- Integrate human-in-the-loop oversight
Human oversight is necessary to validate your AI’s data and model performance. The involvement of domain experts or subject matter specialists during data labeling and validation processes can provide critical insights and catch issues that might otherwise go overlooked. This human involvement helps ensure your AI’s outputs align with real-world expectations, particularly in high-stakes scenarios where inaccuracy poses a greater threat.
Adversarial testing can also help uncover vulnerabilities by challenging your AI model with deceptive or complex inputs. This step strengthens the model’s resilience, ensuring it can handle tough scenarios and deliver robust solutions.
- Prioritize model explainability and interpretability
For your AI models to be trusted, they must be explainable and interpretable – your models must clearly outline how they arrive at their decisions.
AI transparency not only creates accountability and strengthens confidence in the system, but also ensures traceability from input data to model outputs. This means your organization can more easily maintain trust and clarity around how AI decisions are made, and intervene to take appropriate action and provide feedback when outcomes are poor.
- Establish ethical AI guidelines
Developing and enforcing ethical AI guidelines is important with all data inputs, but especially when working with UGD. Collaborate with relevant stakeholders to create policies that govern the use of UGD, particularly concerning privacy and fairness.
These guidelines can provide a foundation for responsible AI development, helping your organization maximize the benefits of UGD without sacrificing ethical standards or user trust.
More Reliable AI, Powered by User Data
UGD offers immense potential to quickly enhance and scale AI models by providing diverse, real-world insights that help refine performance and boost intelligence.
However, as with many innovations, the use of UGD introduces inherent risks, and it’s vital to be aware of those risks. Mitigating any potential vulnerabilities requires starting small, experimenting and scaling gradually as you refine your AI approach and layering in ample IT oversight focused on explainable AI outputs and integrated human feedback loops.
By applying strategies like data preprocessing, bias monitoring, and adversarial testing, you can more safely tap into the power of UGD while still ensuring your AI models remain reliable and resilient.