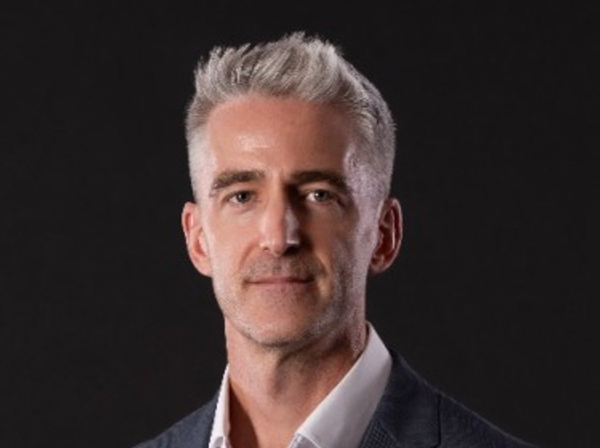
AI has the power to transform industries by analyzing massive datasets and automating complex processes. However, AI’s effectiveness is directly tied to the integrity of the data fueling it. Data governance is required to drive accountability around privacy and ethics, while poor quality data results in inaccurate AI outcomes, leading to customer dissatisfaction, delayed decisions, and increased costs. Despite AI’s potential, only 12% of organizations report that their data is truly AI-ready.
Organizations are enthusiastic about AI’s future, but tangible returns are yet to be realized by many. Ensuring optimal data quality and strong data governance practices as part of a robust data integrity strategy is critical to fully harness AI’s capabilities and avoid costly missteps. Establishing a strong quality and governance mindset across the organization is also crucial as there needs to be clarity around policies, roles, and overall business goals.
As an example, a leading e-commerce retailer noticed its product recommendation engine was performing poorly, causing a drop in customer satisfaction and sales. Investigations revealed that inconsistent product attributes from multiple data sources were distorting the AI models. To fix this, the company introduced a formal data governance framework to standardize data definitions, set up robust data quality checks for anomaly detection, and implemented data observability tools to monitor real-time pipeline health. Within a few months, recommendation accuracy increased significantly, leading to a 20% spike in click-through rates and a marked improvement in overall sales – demonstrating how strong data governance, quality, and observability can transform AI outcomes.
Data Governance: The Backbone of AI-Driven Success
Effective data governance lays the groundwork for successful AI adoption. It helps businesses understand their data, where it is stored, who owns it, and how it is being used. Additionally, comprehensive regulatory frameworks like the EU AI Act are being introduced to manage the risks associated with AI. Integrated systems that pull data from multiple sources need strong data governance and quality practices to ensure that workflows run smoothly and deliver reliable results. This establishes confidence in your data by ensuring AI models have access to the necessary information and is used responsibly in compliance with privacy, security, and other relevant policies.
AI has created clear requirements for data governance, but 62% of organizations report it as the most-cited challenge, inhibiting organizational progress of AI initiatives. Data governance answers the specific questions needed to understand and ensure source data is correct for its intended AI model while maintaining compliance with privacy and security regulations. It also allows for data quality standards to be managed to establish and monitor the data’s trustworthiness. By implementing a robust data governance framework, businesses can easily find, understand, and leverage critical data – leading to more accurate and informed AI insights, decisions, and reporting. In fact, organizations that invest in data governance programs report a wide variety of benefits, with 58% highlighting improved data quality, alongside improved quality of data analytics and insights.
Data Quality: Essential for Trustworthy AI Outcomes
Inaccurate, incomplete, or outdated data can sabotage AI initiatives. Ensuring data accuracy, timeliness, and reliability is paramount, particularly for AI systems designed to provide personalized recommendations or customer experiences. For example, AI-driven movie streaming services depend on precise customer data – such as age, location, and preferences – to generate relevant recommendations. Inaccuracies in that data lead to skewed suggestions, which diminish user experience and trust.
To avoid this, businesses must invest in continuous data quality improvement. Without accurate, well-maintained data, AI models cannot produce the reliable insights needed for innovation and growth. Unfortunately, many companies treat data quality as an afterthought. However, with AI, unlocking data’s potential requires a strong data quality strategy.
While every business faces unique data challenges, establishing effective data quality management helps pinpoint and address common issues. Data might be accurate but still not useful, or it could come from multiple sources, leading to duplication. By implementing a solid data quality strategy, automated controls can validate new and updated data against real-world criteria. This approach ensures data is accurate, complete, and reliable to encourage data-driven decisions, reduce costs, and boost revenue and compliance, but needs to be proactively maintained for continued results.
Data Observability: The Key to Real-Time Data Management
As a part of data quality management, ongoing monitoring is key especially as data volumes increase and data formats become more complex. Data observability addresses this challenge by proactively identifying data anomalies and ensuring AI models consume only high-quality data. These tools leverage AI/ML techniques to monitor data pipelines in real time, flagging unexpected changes or outliers that could distort AI model outputs.
Consider a business that typically sees 10,000 new customer sign-ups per day. If that figure suddenly drops to 1,000 or spikes to 100,000, a data issue likely exists. Data observability tools can detect these anomalies early, allowing organizations to address issues before they impact AI model accuracy. Moreover, observability ensures that data transformations – like normalizations or enrichments – are executed correctly, preserving data quality throughout the pipeline.
Ultimately, AI’s effectiveness is only as strong as the quality of the data powering it. Poor-quality data not only leads to flawed AI outcomes but also raises privacy and security risks, which can be costly to rectify. In contrast, high-quality data has the power to transform how businesses operate, offering more personalized customer experiences, smarter decision-making, and greater operational efficiency.
Each journey to unlocking AI success is unique. Having the right technology to support data governance, data quality, and data observability is just a piece of the puzzle. Implementing a comprehensive strategic methodology that is reinforced by a cultural commitment to data integrity will help organizations achieve desired business goals. This data strategy will guide organizations toward a more effective AI implementation, enabling them to compete in an increasingly AI-driven world.