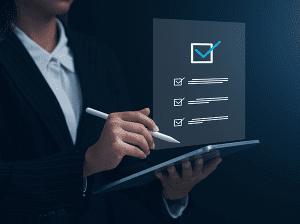
In the rapidly evolving landscape of data management, the concept of data governance has gained prominence as organizations strive to manage, protect, and leverage their data effectively. While developing and implementing a data governance program, it is important to consider how to measure the organization’s current state of data management and develop a plan for regular improvement. This process involves assessing data management and data governance maturity.
Data governance maturity frameworks help organizations assess their data governance capabilities and guide their evolution toward optimal data management. To implement a data governance or data management maturity framework (a “model”) it is important to learn what data governance maturity is, how and why it should be assessed, discover various maturity models and their features, and understand the common challenges associated with using maturity models.
What Is Data Governance Maturity?
Data governance maturity refers to the level of sophistication and effectiveness with which an organization manages its data governance processes. It encompasses the extent to which an organization has implemented, institutionalized, and optimized its data governance practices. A mature data governance framework ensures that the organization can support its business objectives with accurate, trusted, and accessible data.
Maturity in data governance is typically assessed through various models that measure different aspects of data management such as data quality and compliance and examines processes for managing data’s context (metadata) and its security. Maturity models provide a structured way to evaluate where an organization stands and how it can improve for a given function.
Why Assess Data Governance Maturity?
Assessing data governance maturity is crucial for several reasons:
- Benchmarking and Improvement: By evaluating maturity, organizations can benchmark their current state of data governance and data management against industry standards and best practices. This helps identify strengths, gaps, and areas for improvement.
- Strategic Alignment: Understanding maturity levels can support the organization’s plans for improving their data governance practices so they align with organizational goals and regulatory requirements, facilitating better decision-making and confidence with data used for compliance and regulatory reporting.
- Resource Allocation: The results of an assessment can help to prioritize resources and investments in data governance and related data management functions. Organizations can allocate resources more effectively, identify areas where training would be useful, and support investment in areas to provide sustained improvements and benefits.
- Risk Management: Mature data governance practices mitigate risks related to data breaches, inaccuracies, and compliance failures. Regular assessments help in identifying and addressing potential vulnerabilities and offer improvement goals based on best practices and data management industry standards.
- Performance Measurement: Maturity assessments provide an opportunity to develop and refine metrics and benchmarks that an organization can use to measure the performance of their data governance and related data management initiatives over time.
How to Assess Data Governance Maturity
Assessing data governance maturity involves several steps, and effective assessments include all of them:
- Identify a Data Governance Assessment Team: Identify a small group that will be responsible for all aspects of the data governance maturity assessment process. The team should include some data governance and data management senior professionals and at least one person with appropriate experience in assessment and evaluation using maturity models. This requirement may lead to using an external resource, which is often considered a best practice, offering objective viewpoints as well as assessment expertise.
- Define Objectives: Determine what the organization wants to achieve with the assessment and its plans for data governance. Objectives may include enhancing the current data governance practices, improving data quality, enhancing compliance with more transparent data access and usability, or streamlining data management processes such as creating organizationally accepted data definitions or formalizing business participation in data operations. Often, the assessment is part of the organization’s development of a data strategy.
- Select a Maturity Model: Choose an appropriate maturity model based on the organization’s specific needs and goals. Different models focus on various aspects of data governance. Some models are focused on data governance only, while others include related data management functions in their frameworks.
- Gather Data: The team should collect information through surveys, interviews, and documentation reviews. Engaging stakeholders across the organization to get a comprehensive view of current practices is essential, but the number of stakeholders selected should be balanced (neither too few nor too many).
- Evaluate Current State: Compare the collected data against the maturity model’s criteria to assess the current state of data governance and related data management functions such as metadata management and data security.
- Identify Gaps and Opportunities: Analyze the assessment results to identify gaps in data governance practices, identify related data management functions that contribute to the strengths and gaps, and determine opportunities for improvement.
- Develop a Roadmap: Create an action plan that outlines steps to sustain strengths, address gaps, and enhance the organization’s data governance maturity. This plan should include realistic timelines, responsibilities, and resource requirements. Most maturity experts recommend using a phased approach for the roadmap, to reduce the effects of many, sometimes competing, improvement opportunities.
- Develop Reports and Presentations: Write one or more reports to identified audiences (e.g., leadership, stakeholders, relevant departments/teams, etc.). Each report should outline the assessment’s processes and clearly state the results and plans for achieving the assessment’s objectives. Deliver appropriate presentations that summarize the reports and communicate next steps to all stakeholders.
- Implement Changes: Execute the roadmap’s action plans, monitor progress, and adjust as needed to ensure that improvements are effectively implemented. The assessment team may lead some of the implementation activities or the plans may be executed by different teams as appropriate for the organization.
- Review and Reassess: Regularly review and reassess data governance and data management maturity to ensure continuous improvement and alignment with organizational objectives. Most organizations that engage in data governance maturity assessments rely on six-month evaluation intervals for the first two years, and then annually thereafter.
Data Governance and Data Management Maturity Models to Know
Several data governance maturity models provide frameworks for assessing and improving data governance and related data management practices. Some prominent models include:
- The Data Management Association (DAMA) DMBoK Model: The DAMA Data Management Body of Knowledge (DMBoK) offers a comprehensive framework that covers various aspects of data management and governance. It provides a maturity model with five levels, from initial (ad hoc) to optimized (predictive and proactive) practices. The DAMA model emphasizes the importance of establishing data management processes, data quality, and data stewardship as foundational elements of mature data governance (DAMA International, 2017).
- The Gartner Data Governance Maturity Model: Gartner’s model includes five stages of maturity: Initial, Developing, Defined, Managed, and Optimized. It focuses on key aspects such as data ownership, data quality, and compliance. The model highlights the need for organizations to progress from reactive and fragmented practices to a proactive, integrated approach to data governance (Gartner, 2021).
- The IBM Data Governance Maturity Model: IBM’s model comprises four stages: Initial, Repeatable, Defined, and Managed. It emphasizes the need for clear data policies, standardized processes, and metrics to measure governance effectiveness. IBM’s model is particularly useful for organizations looking to implement structured data governance practices and achieve consistency across data management functions (IBM, 2020).
- The CMMI Institute Data Management Maturity Model: The Capability Maturity Model Integration (CMMI) Institute provides a maturity model that integrates data management practices with overall process improvement. It includes five levels: Initial, Managed, Defined, Quantitatively Managed, and Optimizing. This model focuses on continuous improvement and the integration of data management processes with organizational goals (CMMI Institute, 2018).
- DCAM: DCAM – the Data Management Capability Assessment Model – is developed and maintained via the collaboration of EDM Council members. It enables organizations to have trust and confidence that the data and analytics programs are built on industry best practices and have been proven across industries. It provides a structured framework across the data lifecycle and supply chain – from establishing a data management foundation through the application of analytics. (DCAM, 2020)
Features of Data Governance Maturity Models
Each data governance maturity model has distinct features that cater to different organizational needs:
- Assessment Criteria: Models define specific criteria and metrics for evaluating data governance practices. These criteria typically cover aspects such as data governance policies, data quality, data security, metadata management, and compliance.
- Stage Descriptions: Maturity models outline various stages or levels of maturity, providing a roadmap for organizations to follow as they improve their data governance practices.
- Best Practices: Models often include best practices and recommendations for advancing through maturity stages. These practices guide organizations in implementing effective data governance processes.
- Benchmarking: Many models offer benchmarking capabilities, allowing organizations to compare their maturity levels with industry standards or peers.
- Continuous Improvement: Mature models emphasize the importance of continuous improvement and adaptation to changing business needs and regulatory requirements.
Challenges of Using a Maturity Model
While data governance maturity models provide valuable frameworks for assessing and improving governance practices, they come with challenges:
- Model Complexity: Many maturity models are complex and may require significant time and resources to implement. Organizations need to ensure they have the capacity to effectively handle the complexity involved in using these models. Additionally, some data governance maturity models do not address the relevant related data management functions, such as metadata management, data quality management, or data security to a sufficient level of detail for some organizations. Using any of the standard data governance maturity models requires the organization adapt some content or approach to support the organization’s culture.
- Subjectivity: Assessing maturity can be subjective, as it often relies on self-assessments and qualitative data. Organizations need to ensure that assessments are objective and based on accurate information. This is one reason that best practices recommend the use of an assessment expert as part of any assessment team.
- Dynamic Nature of Data: Data governance practices and requirements evolve over time. Maturity models may need frequent updates to remain relevant and effective in addressing emerging challenges and technologies.
- Cultural Resistance: Implementing changes based on maturity model assessments can face resistance; organizational culture may not accept the views discovered in an assessment. Adopting and sustaining effective change management strategies and choosing a maturity model carefully can help overcome resistance and ensure successful implementation.
- Integration with Existing Processes: Integrating maturity model recommendations with existing data management processes can be challenging. Organizations need to align new practices with current workflows and systems. Most data management experts recommend the use of a phased approach with integrated teams for all implementation initiatives.
- Resource Constraints: Implementing and advancing through maturity stages may require significant resources, including time, money, and expertise. Organizations must carefully plan and allocate resources to achieve desired outcomes. Developing a phased and detailed set of roadmaps can help manage resources accordingly.
Conclusion
Data governance maturity identification and improvement can help organizations to optimize their data management practices and achieve strategic goals. Assessing data governance maturity helps organizations benchmark their current practices, identify gaps, and implement improvements.
Various maturity models offer frameworks for evaluating and advancing data governance capabilities, each with its unique features and focus areas. However, each framework should be adapted to the organization while retaining its focus on best practices and objective measurement.
Organizations using these models can discover challenges such as complexity, subjectivity, and resistance to change. By understanding and addressing these challenges, organizations can leverage data governance maturity models to enhance their data management practices and continue to understand and improve management of their data resources.