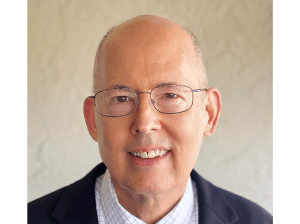
Data management professionals face growing challenges in handling the vast amounts of live data generated by live systems, such as transportation networks, power grids, and security infrastructures. IoT devices have proliferated widely and produce real-time data on an unprecedented scale, placing significant demands on traditional data processing methods. Legacy approaches – often reliant on batch processing and siloed databases – struggle to keep up, resulting in an inability to respond in the moment to dynamic issues. New technologies, including digital twins and generative AI, promise to address these challenges. Used in combination, they can deliver real-time analytics and breakthrough decision-making capabilities to assist in the management of today’s complex, live systems.
Digital Twins Offer Powerful Analytics and Prediction Capabilities
Digital twins are emerging as a powerful tool for managing the complexities of live data. A digital twin is a software-based, virtual representation of a component in a physical system. While originally created for product design and evaluation, it also can be used to track live behaviors and continuously monitor for emerging issues. A complex system, such as an airport or smart city, might have millions of digital twins representing many different types of components.
What gives digital twins their power is the contextual information they store about their corresponding physical components. This information assists them in analyzing IoT telemetry and making informed predictions and enables digital twins to offer a powerful, real-time view of an organization’s operations. Unlike traditional techniques that rely on a human in the loop, digital twins can continuously monitor live operations. They help ensure that businesses can respond quickly and effectively with better situational awareness.
Consider a security operations center monitoring potential cyber threats across a large-scale corporate network. Security teams must detect anomalies, such as unusual login attempts or network traffic spikes, before they escalate into full-blown security incidents and multi-step “kill chains.” Traditionally, anomaly detection relies on the time-consuming and error-prone process of manually inspecting static security logs and databases to find unusual patterns. In contrast, digital twins can continuously monitor telemetry from security endpoints and build up contextual information that more reliably identifies potential threats and helps eliminate false positives.
Using Generative AI to Enhance Digital Twin Decision-Making
In the process of analyzing incoming telemetry, digital twins maintain and continuously update information about the dynamic state of a live system. Operations managers can query and analyze this information for better situational awareness, which helps in decision-making when issues arise. The integration of generative AI enhances the ability of digital twins in performing these tasks. In addition to helping develop code that runs within digital twins, AI can assist managers in creating and interpreting queries to help them find and respond to dynamic issues.
For example, smart cities rely on IoT sensors embedded in systems such as traffic control, energy grids, building management, and water/sewer networks. These sensors continuously generate massive amounts of live data, which digital twins can monitor for anomalies. However, the overall complexity of systems like these can be challenging to manage. Generative AI can add the next level of analysis to help managers assess the scope of emerging incidents and react more effectively. It can look for anomalies that span many components and present real-time analytics that pinpoint problems. It can also determine when to issue real-time alerts to appropriate emergency responders, giving them vital time to address issues before they escalate.
Leveraging Digital Twins to Enhance Generative AI
In an interesting turnabout, digital twins can also enhance the power of AI in providing insights. While generative AI is powerful, it can sometimes produce incorrect or irrelevant information – known as hallucinations – when it relies on generalized models or low-quality data. Using a technique called “retrieval augmented generation,” or RAG, AI systems can access databases to help ground results and eliminate errors and hallucinations. When dealing with live systems, AI needs the latest information so that AI-driven insights are based on the most accurate data. By giving AI models access to real-time data, digital twins can help them generate precise responses instead of relying on outdated knowledge bases.
For example, generative AI can assist airlines in their daily operations to maximize on-time performance, efficiency, and customer satisfaction. To make this possible, AI needs the latest information about an airline’s vast array of components, including flights, passengers, bags, gates, maintenance, and much more. With the constant influx of data that airlines must handle every second, their databases may not reflect the latest information. By using RAG to access the state of digital twins, generative AI can tap the most up-to-date information to provide guidance on dynamic decisions, such as making schedule changes due to weather conditions.
Wrapping Up
As data continues to grow in both volume and complexity, data professionals are increasingly challenged in managing live operations across interconnected systems. The integration of digital twins and generative AI offers a powerful solution, providing in-the-moment insights that streamline workflows, enhance decision-making, and boost operational efficiency. Whether optimizing airline flight schedules or improving situational awareness in cybersecurity, AI-powered digital twins enable data teams to shift from a reactive to a proactive posture, ultimately leading to better outcomes and important competitive advantages.