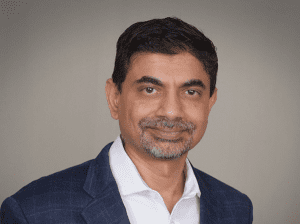
AI is the new technology darling of the business world, and rightfully so. IDC found that companies that invest in AI realize an average return of $3.50 for every dollar spent. Generative AI alone could add the equivalent of $2.6 to $4.4 trillion annually to the global economy across the 63 use cases McKinsey and Company analyzed.
The excitement is warranted, but companies often lack the high-quality data needed to initiate a successful AI project. So, they need to lay a proper data foundation before taking on AI. Here are the three main factors to consider in order to determine your AI project readiness:
- Data Location: Understand where your data resides within your company’s landscape. This involves mapping out data sources and data flows to ensure accessibility and integration capabilities.
- Data Documentation and Harmonization: Establish best practices for documenting and harmonizing data. This includes creating metadata, data dictionaries, and standardized schemas to ensure consistency and clarity across disparate data sets.
- Data Quality: Prioritize the integrity of your data. Evaluate your data’s accuracy, completeness, and relevance to ensure it meets the stringent requirements of your company and compliance needs.
AI projects can’t produce quality results based on bad data. Irrelevant or inaccurate data leads to irrelevant or inaccurate output. AI initiatives are simply too complex and too costly to get them wrong from the start because your data was bad.
Data Is Foundational
Data is the foundation for AI; that’s how it gets trained, and then the trained model processes data according to the developer’s intention. Is your goal to use AI to help solve a business problem, including if you’re using a generative AI tool based on a large language model (LLM)? Then you’ll need to provide it with the proper business context – good data – so it can give you answers specific to that business context. In other words, you don’t just dump whatever data you have into the model.
Are you creating a new model? If so, you must know which data from your trove is appropriate for training and validating it. You need to segregate that data so you can train the model based on one set of data. Then you will validate the model against another set of data to make sure it’s working as intended.
Barriers to a Solid Data Foundation
It’s a significant challenge for many enterprises to determine where their data is stored and how available it is. Do you know what kinds of data exist in your business? Do you know where it is and what rules govern that data? That’s a great place to start. Frankly, many organizations don’t know these things, but they are essential.
Having data doesn’t always mean having ready access to it. Data may exist in multiple systems and silos. Enterprises are especially known for having rather complex data landscapes. They tend to lack one curated database that features all that the model needs, laid out in rows and columns, waiting to be retrieved.
In addition to data being spread across many systems, it’s also in multiple formats: data lakes, graph databases, SQL databases, NoSQL databases. In some instances, you can only access data through proprietary application APIs. Some data is structured, and some isn’t. Some data is coming in near real time from an IoT sensor, some is stored in files and so on. Gathering all this data is a challenge, as most companies don’t have systems or tools that can do it.
Let’s say you find all your data and translate it into one common format that your business can understand. That’s the canonical model. The next step is to consider the quality of that data. It may look great from afar, but close up, you find the duplications and errors that are unavoidable when data comes from many sources. Data in this shape is not fit for purpose.
Three Steps for a Solid Data Foundation
Understanding your data is the cornerstone of your AI initiative. You must be able to describe what data is being captured by your business, where it dwells, what business rules have been assigned to it and so on.
The second step is data evaluation. You need to answer the question of what good data looks like for your business needs. You must determine what constitutes high-quality data in your organization. You need to establish rules for how to validate and cleanse the data, as well as a plan for how you will maintain the desired quality across the data’s lifecycle.
Should you succeed at moving data from disparate systems into a canonical model and improve its quality, you must still make it scalable. This is step three in establishing your data foundation. Most AI models need massive amounts of training data. And then you’ll need a great deal of data for retrieval-augmented generation – a method of augmenting generative AI models with data taken from external sources. All of this data changes constantly.
This requires you to have a plan for the appropriate scalable data pipeline that can manage the quantity of data that might flow through it. At first, it’s so overwhelming just to determine where to get the data you need, how to cleanse it and so forth that scalability doesn’t occur to you. But you must think through which platform you’ll use to create this initiative – one that can scale along with the volume of your additional data as time goes on.
Quality Data, Quality Outcomes
If you’re serious about creating and maintaining a competitive advantage with AI, you’ve got to start with the data. Gathering data and preparing it to achieve business goals is complex and difficult. But your competitors aren’t waiting for you to get it right, so there’s no time to waste in getting it wrong. You must start with the firm foundation of a platform and a process that will enable you to sustain high-quality data. Follow the best practices discussed above and you will have an AI model that can help you achieve your business goals.