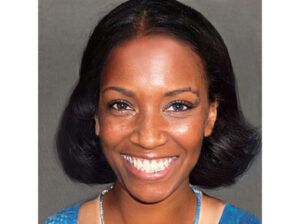
Businesses today are drowning in data. The sheer volume and complexity of information available have made it increasingly difficult for organizations to extract meaningful insights using traditional business intelligence (BI) tools and the expertise of specialized data scientists. This is where augmented analytics comes in. This game-changing technology combines the power of artificial intelligence (AI) with BI to empower non-technical users to uncover valuable insights and make informed decisions.
And everyone is already joining the party. Data shows that the demand for augmented analytics is expected to expand at a moderate CAGR of 21.8% from 2023 to 2033.
This article will explore the key components of augmented analytics, its transformative impact on business users, and the AI-driven functions that are redefining the data analysis landscape.
What Is Augmented Analytics?
Augmented analytics is the use of AI, machine learning (ML), and natural language processing (NLP) technologies to automate the process of analyzing data.
It helps prepare data, generate insights, and explain these insights in an easy-to-understand manner. This enables both technical and non-technical data scientists to make informed decisions without deep expertise in data science.
For instance, in addition to providing a wealth of data for marketing purposes, the integration of AI and BI could even prove to be relatively autonomous. Take the example of backlink management – identifying broken links, maintaining a healthy balance of dofollow and nofollow links, removing toxic backlinks, doing competitor analysis – all of these are relatively mundane (yet crucial) SEO practices that could be automated, saving your experts time for truly important endeavors.
The Evolution of Analytics
In the not-so-distant past, data analysis was the domain of a select few – highly trained data scientists and BI experts who navigated the intricate world of spreadsheets, statistical models, and complex visualizations. This specialized knowledge was often the key to unlocking valuable insights buried deep within the mountains of information that businesses collected.
However, as the digital revolution accelerated, the volume and variety of data grew exponentially, quickly outpacing the ability of even the most skilled analysts to keep up. Organizations found themselves drowning in information, unable to translate their data into meaningful, actionable insights that could drive strategic decision-making.
Today, augmented analytics has quickly democratized data analysis and empowered even non-technical business users to explore and derive insights from their data.
Augmented analytics automates many of the tedious tasks associated with data analysis, such as data preparation, anomaly detection, and natural language querying. This automation reduces the burden on specialized data teams and allows employees across departments to participate actively in the insight-generation process.
The combination of BI and AI also promises to level the playing field and introduce a more egalitarian approach to the data market. This powerful integration won’t just be in the hands of big business – in the near future, even a small business will be able to leverage this synergy to identify a unique, underserved niche, optimize invoice factoring for optimal cash flow at all times, or simply super-charge the biggest drivers of growth for the best possible return on investment.
The Components of Augmented Analytics
Augmented analytics combines the power of AI and BI tools to transform the landscape of data analysis, making it accessible and actionable for business users without deep technical expertise. Here are the core components of augmented analytics:
Machine Learning (ML)
This AI technology is the backbone of augmented analytics. It allows systems to learn from historical data, identifying patterns, deviations, and generating insights without human intervention.
Machine learning models are designed to thrive on big data, improving over time as they process new structured and unstructured data, making them indispensable for data analytics capabilities.
Natural Language Technologies
These technologies bridge the communication gap between humans and computers. Natural language processing (NLP) helps computers understand human language, while natural language generation (NLG) translates computer findings into easily understandable human language.
This interaction allows users to engage conversationally with the system, asking questions and receiving answers in terms familiar to their domain or industry, thereby making data analysis more intuitive.
Automation
Automation in augmented analytics refers to the use of machine learning technologies to automate routine manual tasks across the data analytics lifecycle. This significantly reduces the time needed for data preparation, analysis, and the generation of insights.
For instance, automation can help discover and prepare raw data more quickly and generate text-based reports, enhancing the efficiency of insight sharing. It also makes it easier to analyze the provided data, convert everything from PDF to Excel, and more or less have a completely autonomous way of handling different databases.
The Benefits of Augmented Analytics on Businesses
As the adoption of augmented analytics continues to grow, businesses are beginning to experience the transformative impact of this innovative technology.
Democratization of Data Analysis
Traditionally, data analysis has been the domain of highly skilled data scientists and BI experts. Augmented analytics changes this dynamic by empowering non-technical users to participate actively in the insight-generation process.
Augmented analytics automates complex tasks and presents information in a user-friendly format so that employees across departments can easily access, explore, and derive insights from their organization’s data.
This will both streamline and simplify business processes – no more worrying about how many business accounts you should have, whether or not your financing option offers optimal interest rates, or if adopting new standards of technologies is worth it in the long run. The relevant teams can easily use data to find the right information without waiting for an expert to analyze it.
Reduction of the Data Science Bottleneck
One of the most significant challenges faced by businesses is the shortage of data science expertise. Augmented analytics helps alleviate this bottleneck by reducing the reliance on specialized data professionals.
With the ability to automate data preparation, anomaly detection, and natural language querying, augmented analytics allows organizations to scale their data analysis capabilities without the need for a large team of data scientists.
Faster Decision-Making and Improved Business Outcomes
Augmented analytics boosts efficiency and makes data more accessible, leading to significant business advantages.
This technology simplifies the examination of complex data, helping organizations quickly discover insights and base their decisions on data. Such swift decision-making and enhanced insight quality greatly improve a company’s profitability, operational efficiency, and competitive edge.
The impact of these advancements on everyday business operations is profound. For example, companies might use these insights to improve their cash flow through strategies like accounts receivable financing or refine their business models for better predictions. Already, some businesses are leveraging AI to evaluate insurance risks more accurately.
Higher Accuracy and Efficiency
Augmented analytics combines AI and ML with human oversight, where people review and make final decisions on the suggestions provided by machines. This blend enhances accuracy by minimizing errors from both sides and automating many routine data tasks.
As a result, data collection and analysis become quicker and less reliant on constant human intervention, freeing up time for more strategic activities like deriving insights, planning, and process optimization.
3 Challenges of Augmented Analytics
Data Quality and Governance
Augmented Analytics relies heavily on the quality and integrity of the underlying data. Ensuring reliable, accurate, and well-governed data is crucial for generating trustworthy insights.
Organizations need to have robust data management practices in place to address issues like data silos, inconsistencies, and inaccuracies. Balancing the automation of augmented analytics with human oversight and quality control is essential.
Ethical Considerations
As augmented analytics leverages powerful AI algorithms to uncover insights, important ethical considerations must be addressed. These include potential biases within the data or the algorithms themselves, as well as the need for transparency and interpretability of the analytical models.
Organizations must maintain fairness and avoid unintended consequences as they adopt Augmented Analytics.
Organizational and Cultural Barriers
Successful implementation of augmented analytics often requires a shift in organizational culture and mindset. Business users may be hesitant to embrace new technologies or may lack the necessary skills and confidence to become “citizen data analysts.”
Overcoming resistance to change, providing adequate training and support, and fostering a data-driven culture are crucial for driving widespread adoption of augmented analytics across the organization.
Conclusion
As data volumes and complexity continue to grow, the need for accessible, AI-powered analytics has become paramount. Augmented analytics represents a transformative shift in how organizations leverage their data to drive strategic decision-making.
As the technology continues to evolve, with advancements in areas like natural language processing and edge computing, the future of augmented analytics looks increasingly promising. Organizations that embrace this tech will be well-positioned to thrive in an increasingly data-driven world.