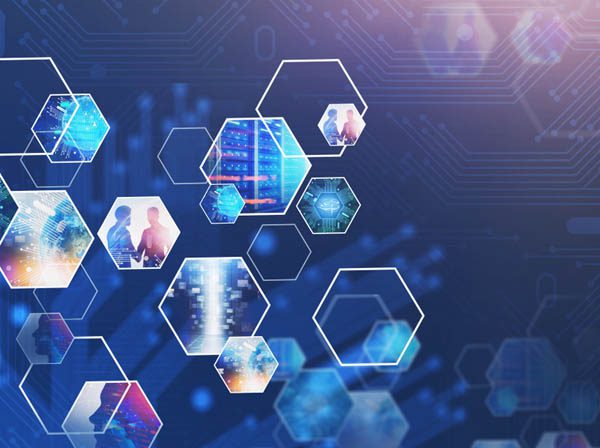
Augmented analytics is one of the top 10 technologies Gartner identified with the potential to cause significant digital disruption in the next three to five years. The hallmark of augmented analytics is its high reliance on AI and ML technologies.
AI and ML together will transform enterprise Data Management in the coming years, and many emerging technologies like the multi-cloud, edge, serverless, IoT, and big data will collaboratively contribute to the forthcoming digital disruption in global businesses. Augmented analytics, the major force of 2020, will impact all facets of Data Management like “Metadata Management, Master Data Management, data integration, and databases.”
As newer and better data technologies continue to emerge, more advanced database technologies will also surface to keep pace with fast-evolving business Data Management challenges. The data today is not only high-speed and high-volume, but also multi-channel, sensor-driven, and complex. Thus, database technology has to match the needs of advanced data analytics and BI in the enterprise.
Relational databases are no longer suitable for real-time, big data analytics, or self-serve BI because the scope of such databases is too limited. The more recent trend with the graph database is rapidly replacing the older database technologies in business analytics. A common misconception that has circulated over the years is that graph processing is only relevant for traditional social network data or network channels. Gartner has predicted that graph processing and graph databases “will grow at 100 percent annually over the next few years to accelerate data preparation and enable more complex and adaptive data science.”
Hidden Opportunities in Emerging Technologies
Gartner Identifies Top 10 Data and Analytics Technology Trends mentions that Donald Feinberg, Vice President and Distinguished Analyst at Gartner, said:
“It’s critical to gain a deeper understanding of the technology trends fueling that evolving story and prioritize them based on business value.”
The evolution of the relational database into the graph database has been happening for several years now, but now it has gained critical importance in handling high-volume, multichannel, real-time data. Speaking in Sydney, Australia, Rita Sallam, Research Vice President at Gartner, commented that:
“Data and analytics leaders must examine the potential business impact of these trends and adjust business models and operations accordingly, or risk losing competitive advantage to those who do.”
One emerging technology that works well with both AI and ML, especially in the realm of business analytics, is the graph database, which has been described as “the future of database technologies.”
Graph databases define inter-relationships in terms of “nodes” and “edges.” In sharp contrast to the relational database, the graph database stores relationships inherently. This trend reflects that “data” and “relationships” share equal importance in this database model. The graph database has suddenly assumed tremendous importance due to its apparent advantages and flexibility, and is now often trending on technology news sites.
Role of Graph Processing in Augmented Data Management
As data volume is growing exponentially, and the Data Science talent gap is a dark reality, global businesses are rapidly gearing up for automated Data Management solutions.
According to a Dzone article, in the database technology world, graph databases offer significant advantages over relational ones, considering the “explosive volumes of data, real-time query response time, and more intelligent data-activation requirements” of today’s data managers. Recently, graph processing has disrupted supply-chains, e-commerce, fraud prevention, utility sectors, and other sectors with commercial solutions like Neo4j, DataStax, and TigerGraph shooting into overnight fame.
Here are some advantages of using graph databases over relational databases:
- Clear semantics for each query with no hidden assumptions
- In this unique database model, “a native graph” has to maintain the information related to “its neighbor vertices” without having to “load or touch unrelated data for a given query”
- Graph databases can solve a wide range of business problems, whether practical or impractical for relational queries
- Graph databases can conduct queries and update data in real-time concurrently
- Graph databases offer flexibility of use — through continuous “adding or dropping of new vertex or edge types or their attributes to extend or shrink your data model”
- The conjunctive graph query (CQ) feature enables users to submit a “subgraph pattern and request the database to return all subgraph instances that match this pattern”
- Graph databases have the ability to “combine multiple dimensions to manage big data, including time series, demographic, geo-dimensions, and so on”
- Works well with both AI and M platforms
Graph Databases: Mining Social Data
In recent times, graph technologies, along with AI, have brought practical solutions to the business marketplace. Graph Databases: An Overview explains how the flexibility of these databases and “their focus on relationships” have contributed to the rising popularity of this database technology. It also indicates that, to a great extent, graph databases have been used to mine social network data.
The Flourishing of Insights and Data Analytics in 2020, however, suggests that though augmented analytics has benefitted from graph databases with their “deep link analytics,” augmented Data Management still has a long way to go, while the Data Management teams “struggle … with data bloat effect as an unforeseen consequence of abundance of data.”
Graph Processing in Business Analytics: Deep Explorations
Uncovering Data Connections for Competitive Advantage in 2020 shows how businesses, irrespective of size or annual revenue, can gain by implementing graph databases or graph processing in 2020.
Here is what Vishnu Maddileti, Director of Data Services and Analytics at Amgen thinks about TigerGraph:
“We deal with a humongous amount of data, electronic medical records, and comprehensive health records. With 5 billion vertices and 20 billion edges, it’s huge data, and finding inferences in that data is not easy, but TigerGraph has scaled for us.”
Another blog post from a UK-based company staff highlights how advanced algorithms may be used with graph theory to reveal hidden insights. According to this post, graph processing plays an important role in artificial neural networks but its potential is still unrealized in business analytics. Towards Data Science steers the reader through an involved technical discussion on the “Concept of Centrality” in graph analytics, and its application in major social networks like Twitter.
Graph Processing in Business Analytics: Applications
In a graph database, the basic assumption is that data is stored, managed, and queried in graphical format. Due to its graphical (visual) property, it becomes easier for users to discover patterns and relationships. In 5 Innovative Ways to Use Graph Analytics, Oracle developers share some useful applications of the graph database in real-life business situations.
Graph databases and graph processing have been voted the “future of database technologies” by many leading business publications and news sites, which is aptly reflected in the article Graph Database — the Future of Database Technologies.
Image used under license from Shutterstock.com