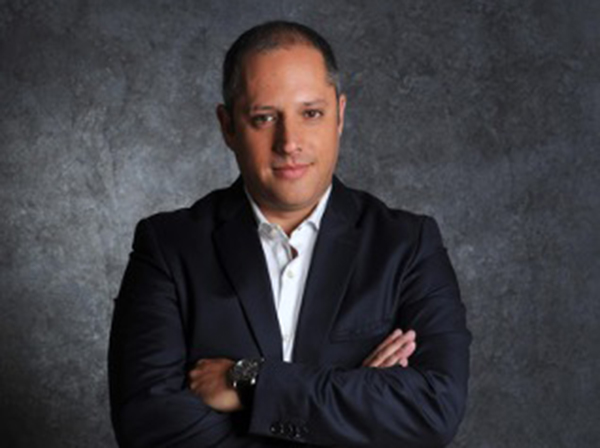
With over 12,000 years of experience in agriculture, humans have learned quite a bit about the many aspects of agriculture. From plant domestication to farming, agriculture has grown to be the backbone of the world – providing food, fuel, feed, and fiber.
What was once dominated by ox and plows now relies on technologically driven tractors. Decisions that were traditionally reliant on grower experience and luck can now be based on decades of data, statistics, and predictive analytics.
Farmers are bombarded with more tasks than simply watching the weather – they need to monitor fields for crop diseases or pests, follow fluctuations in commodity prices, time fertilization correctly, and more. With the risk that is innately tied to agriculture, combined with these responsibilities, predictive analytics can weigh all these factors and help farmers make the best decisions for the future using prediction.
Innovative technology will continue to help address and improve the global food system, and utilizing predictive analytics is one of those ways.
What Are Predictive Analytics Tools?
Predictive analytics tools use artificial intelligence and algorithms to predict crop outcomes, management inputs, and other system changes using agricultural, biological, climatic, hydrological, and economic data. This includes crop modeling, machine learning, and data mining, to name a few.
Predictive analytics takes precision agriculture to the next level. Precision farming has used technology to measure resources and inputs and allow growers to use the most precise and accurate amounts of inputs to maximize productivity. Precision analytics relies on statistical approaches and full systems modeling to predict future outcomes. Using numerous data measurements related to climate, soil, genetics, management, and crop physiology over a period of time, growers can continue making data-driven decisions for the future.
Taking the necessary steps to avoid problems is preferred to solving the problems once they exist, which is where prediction comes into play. There are many issues in agriculture where avoiding problems (e.g., through agricultural systems design or developing adequate crop management solutions) is a far preferable approach than solving problems once they have occurred. Being able to predict the best approach saves growers time, money, resources, and headaches.
With the seemingly never-ending datasets in agriculture, growers can get predictions to help with decisions relating to what crop to plant, when to plant, what fertilizer to apply and when to apply, when to irrigate, and what treatments to minimize the effects of anticipated pests or diseases, when to harvest, and more.
The Benefits of Predictive Analytics
Predictive analytics can be used in many steps of the agricultural process, from before the seed is placed in the ground through harvest. The benefits of using predictive modeling and analytics include: selecting the best crop, optimizing management practices, improving crop productivity, and assisting in sustainable decisions.
Select the best crop for your field
- By using soil analysis data, historical weather, and other parameters, growers can make the best crop selection for their field.
- Different varieties of crops are bred for specific latitudes based on relative maturity, stress tolerances that might be known or anticipated (biotic and abiotic), desired planting densities, and specific uses (food, biofuel, feed), among other things.
- Depending on the overall goal and the attributes of the field, growers can use predictive analytics to decide what crops would be best suited for a given growing season.
Optimize land management practices
- Correlating GPS-developed field management maps with crop productivity maps can allow farmers to optimize field operations based on specific field characteristics and performance.
- Using historical weather data, crop stress periods can be predicted, along with the optimal amounts of irrigation needed, based on crop growth stages and models.
- Predictive analytics can create management zones and prescription maps for various inputs, such as fertilizer and herbicides.
Improve crop productivity and yield
- With historical pest data and imaging, growers can understand the patterns with specific pests and adjust pesticide use to optimize crop protection and productivity.
- Using predictive analytics can help growers forecast future yield and productivity based on the resources they have available and modify the models, or take measures when needed.
- Predictive analytics can Identify fields and subfields where ROI is repeatedly lower and suggest if these fields should be let out of production. This can allow growers to save time, money, and resources in potentially wasted areas, depending on the ROI.
- With the expected increase in world population to near 10 billion by 2050, a substantial increase in crop yield will be needed. Being able to predict crop productivity will allow growers to continuously strive to increase yields and adjust various inputs to ensure goals are met.
Assist in sustainable decisions
- Sustainability-related factors, such as energy use, resource allocation, and greenhouse gas emissions, can be considered with predictive analytics to allow farmers to optimize resource efficiency and sustainability.
- Predictive analytics can help identify conditions where environmental pollution risks are high, relate agriculture actions to environmental footprint, and reduce detrimental environmental decisions.
- The long-term prediction ability can aid farmers in making decisions earlier and limiting resource waste, such as overbuying or over-applying fertilizer, needing to replant, over irrigation, crop loss, etc.
Predictive analytics tools can result in improvements in crop quality, crop yield, better ROI, and more sustainable agricultural production systems.
The Current Challenges of Predictive Analytics
There are a number of challenges that limit the effectiveness, or accuracy, of predictive analytics in agriculture.
- Prediction models rely on large datasets that are rich in the number of observations in a given year, and across a number of years. When datasets have missing entries, model results become less statistically sound.
- The large amounts of data that are necessary for predictive analytics require substantial computational resources for computing and storing, which can be a large financial investment.
- There are many data sharing barriers in place between public agencies and private sector companies relating to data privacy and differences in data standardization.
With the unprecedented change in climate and current challenges facing predictive analytics, it’s essential that developments are made to address these concerns.
The Future of Predictive Analytics
Predictive analytics tools are not limited to just the large industry companies – they will be the future for all growers and researchers.
Already, predictive analytics have played important roles in simulating crop modeling. Crop model simulations can predict yield, reproductive time, stress management, and other anticipated input needs. These models rely on biological, climatic, and hydrological data to make meaningful predictions. Some data measurements are more informative than others and some data measurements are highly correlated (meaning that time does not need to be used to collect all measurements, but a reduced set). Continued research and work will help fine-tune these agricultural data management and modeling tools to improve prediction accuracy and application.
As technology continues to advance and become more accessible, similar to what we’ve seen with GPS-enabled machinery and phone-based applications, growers with any amount of cropland will be utilizing predictive analytics to make decisions for their fields. With increased use and research on predictive analytics, the accuracy and overall capabilities could provide even more meaningful insights for producers.
Predictive analytics are important tools that will help achieve more prosperous and sustainable agricultural developments in the immediate future and long term.