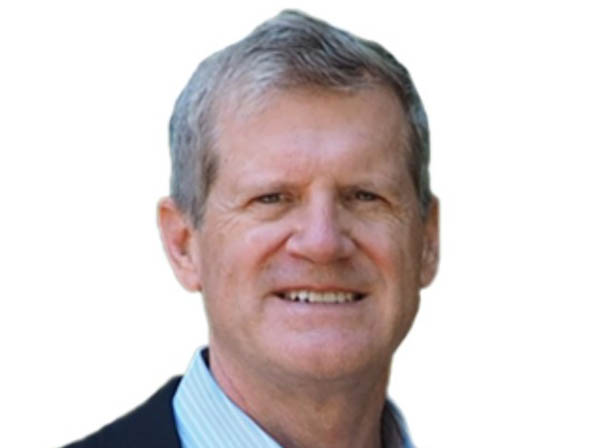
Data fabric is redefining enterprise data management by connecting distributed data sources, offering speedy data access, and strengthening data quality and governance. Many enterprises are already extracting business-relevant intelligence by putting their data to work through an efficient data fabric architecture. This article gives an expert outlook on the key ingredients that go into building an ideal data fabric architecture and streamlining data management.
Data fabric is the hot topic that is making heads turn in the IT industry. Its game-changing capabilities help maximize the value of data volumes that enterprises are sitting atop, by managing and monitoring data more efficiently. Yet experts do not necessarily view data fabric as a novel concept. They believe it has been evolving over the past few years, with different names like enterprise information architecture or enterprise data architecture.
What Does Data Fabric Really Do?
Data fabric is a design concept that integrates data sources into a layer, with connected processes. It drives the continuous analysis of discoverable metadata assets across a hybrid or multi-cloud environment, to produce business-critical intelligence.
In its newest instance, data fabric lays prime focus on metadata and connectivity across various data sources. Metadata essentially means data about data, which is curated for easy discovery, usage, and management of information.
Earlier, information architecture was focused on data integration alone, which helped access data in the respective data sources and consume it within multiple types of warehouses.
Today’s successful data fabric leverages comprehensive data catalogs, data glossaries, and metadata functions. AI and machine learning are also being embedded into data fabric to drive automation and self-learning for Data Governance, which validates organizational control, access, and use of sensitive data.
At its current evolutionary stage, experts have reasonable clarity on what an ideal data fabric should look like. We’ll delve into that in the next section.
Building the Ideal Data Fabric Architecture
Constructing the components of a data fabric is a slow and evolving venture that necessitates a sizeable investment of time and money. But above everything, enterprises must begin by understanding that the data at their disposal is closely tied to multiple processes and the associated teams that access that data. It is critical to consider integrating those processes while assembling the components of the data fabric.
A successful data fabric is only achievable when a robust Data Governance program is in place to dictate the rules that users and systems must obey while supervising, managing, and monitoring enterprise-wide data.
The components that form the foundational layer of data fabric are as follows:
- Master Data Management: Master Data Management (MDM) helps maintain clean, de-duplicated data ready for advanced analytics to provide a unified view of the customer, product, and other enterprise information. In an advanced implementation, MDM will typically export metadata into a graph database that enables downstream analytical capabilities.
- Business Glossary: A business glossary is essential to capture all of your business terms, policies, and processes and visualize relationships among data elements and systems.
- Data Catalog: Data cataloging helps you scan and identify all of your technical data assets across the enterprise, enriching data with tag annotations and linking to business terms from the glossary.
- Metadata: The strength of the data fabric lies in connecting all relevant enterprise data. It is imperative to collect the associated metadata because data catalogs, glossaries, indexes, and policy implementations, all determine the degree of access you have to data.
- Data Lineage: With data lineage, your enterprise can track the end-to-end data lifecycle from origin to consumption, ensure compliance, and perform impact analysis for changes to any data elements.
- Policy Management: Defining data policy rules helps your enterprise monitor and report on applications and ensure data alignment with governance policies.
Lastly, it’s almost a given that if you’re going to have a robust data fabric, data integration is an essential backbone that helps access and manage data virtually and remotely from anywhere.
While kick-starting the transformation journey with data fabric, enterprises must organize their priorities strategically. Establishing an overarching view of the outcome expected from data fabric integration is necessary. Decision-makers must decide and chalk out critical objectives, roles, and responsibilities of teams and optimal performance standards for the implementation.