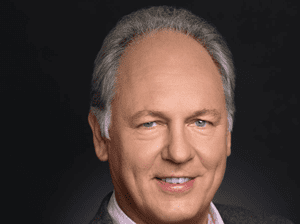
There are several reasons why the notion of semantic layers has reached the forefront of today’s data management conversations. The analyst community is championing the data fabric tenet. The data mesh and data lake house architectures are gaining traction. Data lakes are widely deployed. Even architectural-agnostic business intelligence tooling seeks to harmonize data across sources.
Each of these frameworks requires a semantic layer to ascribe business meaning to data – via metadata – so end users can understand data for their purposes and streamline data integration. This layer sits between users and sources, so the former can comprehend data without knowing the underlying data formats.
Additionally, a semantic layer must incorporate a digital asset knowledge graph for a unified description of data assets in all sources – like those feeding data lakes and data lakehouses. This catalog is especially important for identifying what data is in unstructured data sources, relational databases, streaming data, document stores, and other sources for data fabric or data mesh deployments.
Some “semantic layers” use non-standard, proprietary technologies to store metadata. This approach prevents the use of industry-wide ontologies like FIBO, (financial services), SNOMED (medical), SCONTO (supply chain), OBML (life sciences), CDM-Core (manufacturing), GoodRelations (e-commerce), or SWIM (aviation). It also complicates future data integration and reinforces vendor lock-in.
Conversely, semantic layers implemented with W3C’s Semantic Technologies are based on open-source standards that complement an organization’s existing IT infrastructure. They future-proof the enterprise, prevent vendor lock-in, and provide a uniform view of all data (regardless of differences in formatting, types, and structure) that’s optimal for data integration, data governance, and monetization opportunities.
Semantic Layers with W3C’s Semantic Technologies
The proliferation of unstructured and semi-structured data from external sources is partly responsible for the current demands for a semantic layer. Data fabrics, data lakes, and data lakehouses contain a surplus of such data, which is useful to everyone from data scientists to BI users. Applying a standardized semantic layer atop these architectures lets end users select data through a lens of business understanding, in which data assets are described by metadata in familiar business terms.
This layer is endlessly reusable for any use case, from building machine learning models to devising applications or running analytics. Standardized semantic technologies provide a semantic layer via RDF knowledge graphs which contain standardized data models, vocabularies, and taxonomies. The three main elements of a standardized semantic layer include:
- Digital Asset Knowledge Graph: This knowledge graph describes every data asset organizations have via the above metadata and data models. It’s an effective map of what’s in a data fabric, where it is, who owns it, and more.
- Ontology of Business Concepts: This semantic data model describes the important business concepts that give meaning to the data in the digital asset catalog. It contains the terminology, metadata descriptions, taxonomies, and schema in words business users understand.
- Inter-Graph Linkage: The final component links the business concepts and the digital assets. A strong link between these elements is necessary for a systemic data fabric and for making the data mesh architecture viable.
Tangible Advantages
Implementing a data fabric with standardized semantic technologies delivers a number of tangible advantages to the enterprise for the long-term reuse of data. Firstly, organizations will have a uniform view of all the data in their data sources. With the proper curation, this information is invaluable for selecting the best sources for analytics or for loading applications. This approach also disambiguates entities across silos, thereby eliminating silo culture and accelerating data integration.
For example, instead of having a different identifier for the same person in each database, there’s now one Universal Resource Identifier (URI), which is critical for governing data at scale. This characteristic also makes it easier to link data to all datasets in your company, which improves things like regulatory compliance, cross-selling, upselling, and more. Additionally, such a semantic layer makes it quicker and painless to create multiple knowledge graphs for different domains and integrate them—which is critical for data mesh or data fabric deployments.
Proprietary Semantic Layers
A semantic layer without standardized W3C Semantic Technology is predicated on proprietary data formats and technologies. These technologies are primed for vendor lock-in and limiting how organizations can utilize their data. Although they describe data aspects in business terms, they don’t include a digital asset knowledge graph. Without this characteristic, there are still myriad silos across organizations.
Organizations would also have to recreate industry-specific ontologies in their vendor’s proprietary format to use them, which is an unnecessary cost and time sink. But, since a standardized semantic layer with semantic technologies involves universal RDF standards, it’s immediately applicable to industry-wide ontologies. Companies can use any technology or data format with this semantic layer, which will naturally evolve to blend with the RDF standard and the specific data models these knowledge graphs contain.
Business Meaning
There are rare cases where a proprietary semantic layer may work and the organization might not mind getting locked into the ecosystem of a vendor for their metadata management needs. But for the majority of use cases, the best way to future-proof the enterprise is to adopt a standardized semantic layer with semantic technologies. This method provides a seamless business understanding of data that complements any current or future IT needs, while reinforcing data integration, analytics, and data governance.