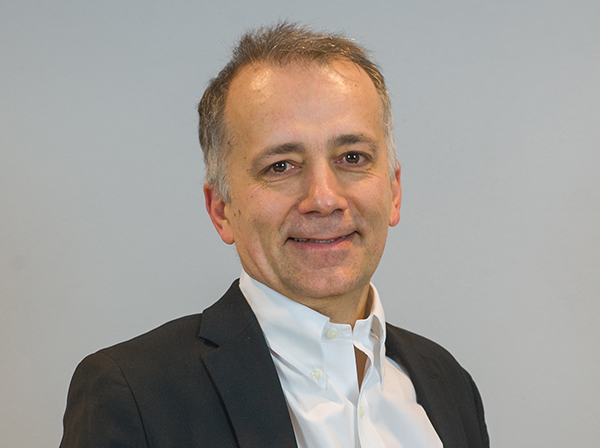
Click to learn more about author Luca Scagliarini.
Artificial intelligence (AI) is on an upward growth trend and there is no sign it will level off any time soon. Recent research by Fortune Business Insights suggests the market will jump nearly tenfold from $27.23 billion in 2019 to $266.92 billion by 2027. This represents a staggering 33% compound annual growth rate (CAGR) as AI infiltrates just about every industry on the planet, creating new applications and systems that cannot even be imagined today.
Despite this massive uptake, however, complete trust in AI is elusive. Yes, it brings greater value to digital business models by automating and simplifying many complex and costly processes, but it also creates unease among consumers that their personal privacy is being exploited by uncaring bots that don’t have their best interests at heart.
To leverage AI to its full potential, then, enterprises must demonstrate that they are utilizing it in a respectful, responsible manner, and the best way to accomplish this is to build as much transparency into the algorithms that drive AI processes without hampering performance or accuracy. Fortunately, we already have the means to achieve this goal by merging the many forms of AI that exist today into a hybrid model.
The Trust Factor
AI is an extraordinarily powerful tool that supports more effective decision-making, streamlines workflows, and lowers costs. But the fact remains that typically we do not know how it operates and know very little of what happens between every input and output.
This produces results that cannot always be readily explained to the ordinary user, and it becomes particularly problematic if customers feel they are being treated unfairly. Bias can never be fully eliminated from any digital platform, but without the ability to detect where it exists, it becomes all but impossible to eliminate.
Ultimately, this creates a level of operational risk that can undermine the value and efficacy of AI as a business tool. Once a company’s reputation becomes damaged over its use of AI, it is very difficult to recover, even after the problem has been resolved. Now, it’s not just the AI initiative that’s in jeopardy but the very future of the organization itself.
This is why many forward-leaning enterprises are taking a close look at their AI programs to ensure they can provide trustworthy, explainable results, particularly when those results run counter to, or do not completely fulfill, users’ desires.
Opening the Box
It might be difficult, understandably, to explain every AI system to a typical user, but we should make the effort to ensure the behavior of an AI system can be explained in order to form a trusting relationship with the technology. Transparency is particularly difficult in machine learning (ML) and deep learning (DL) models. In fact, ML is often defined as “black box AI” because its algorithms are trained to use inference, not actual knowledge, to identify patterns and extract information. ML models must have access to large volumes of data to maintain continual training and learning, but as long as its internal mechanisms are hidden in the black box there is no way to pinpoint the cause of erroneous or biased conclusions. This requires the entire system to be retrained from scratch, which is costly, time-consuming, and usually frustrating.
In the same way, DL is difficult to penetrate because it creates an artificial neural network that learns without human oversight. This allows it to take on more difficult challenges, like detecting fraud or money laundering, but it also produces a highly complex, data-saturated system.
The Hybrid Approach
Newer forms of AI, however, are starting to peel back the layers of transparency. Symbolic AI, for example, leverages high-level, “human-readable” representations of logic and knowledge. It is a critical component of emerging natural language understanding (NLU) platforms that mimic the ability to understand normal speech or day-to-day language.
Symbolic AI utilizes a rules-based approach that provides full visibility into any given model. With this transparency, users can delve right into the inner workings of a process to quickly detect errors in either the data or the algorithm and then create new rules to correct them. Not only does this streamline AI projects and lower costs, it reduces the risk inherent in data collection by shining a light on how it is being used – knowledge that can be shared with customers, clients, or any other user base.
A hybrid approach to natural language, based on the combination of ML and symbolic AI, provides the best of all worlds: a complete understanding of human language that can extract value from unstructured data with the data processing capabilities of ML.
With this framework, we can begin building responsible AI that will allow the enterprise to expand its business model much further and much faster than previously thought possible.