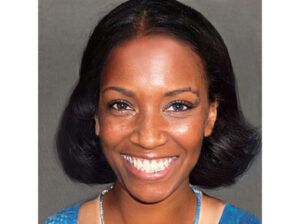
From Fortune 500 companies to local startups, everyone’s swimming in a sea of numbers, charts, and graphs. But here’s the thing: While structured data like sales figures and customer demographics have long been the backbone of analytics, there’s a growing realization that unstructured data is the real goldmine.
Think about it. Every tweet, email, customer review, and social media post is a treasure trove of insights, but this kind of data is just not that easy to analyze using traditional methods. That’s where linguistic analysis comes in. It offers a new lens through which we can decode the hidden messages within unstructured data.
Below, we’ll examine the tools and techniques that give voice to unstructured data and look at real-world examples of how this is reshaping industries.
The Convergence of Linguistics and Data Science
In the current digital era, unstructured data is exploding – it makes up about 80% of the information generated daily. From emails and chat logs to online forums, unstructured data is everywhere.
This data is rich in insights, but it doesn’t fit neatly into rows and columns. Fortunately, that’s where linguistic analysis shines. Linguistic analysis in data science goes beyond traditional number-crunching. It’s about understanding the nuances of language within data.
Unlike conventional methods that focus on structured data, linguistic analysis delves into the complexities of human communication. It examines patterns, context, and meaning in text data, allowing us to extract trends and insights from sources like social media posts, customer reviews, and open-ended survey responses.
Key Principles of Linguistic Analysis
Linguistic analysis in data science marries principles from the two fields. From linguistics, we borrow concepts like syntax (sentence structure), semantics (meaning), and pragmatics (context). These help us understand not just what words say, but how they’re used and what they imply.
On the data science side, we leverage technologies like machine learning and natural language processing (NLP). These technologies allow us to automate the analysis of large volumes of text, identify patterns, and extract meaningful information at scale.
When preparing data for linguistic analysis, it’s crucial to ensure the data is in a format that preserves its linguistic integrity.
Applications of Linguistic Analysis in Data Science
The fusion of linguistic analysis and data science isn’t just theoretical – it has real-life applications both in business and research. Here are some key ways linguistic analysis is transforming raw text into actionable insights across various industries and domains.
Sentiment Analysis: Decoding Emotions Through Language
Sentiment analysis is the process of determining the emotional tone behind words. It analyzes language to understand attitudes, opinions, and emotions expressed within text and identify whether a piece of text is positive, negative, or neutral.
This offers insights into how customers feel about products, services, or brands, leading to data-driven decision-making, improved customer experience, and better marketing strategies.
Multiple linguistic tools and techniques are involved in sentiment analysis. Lexicon-based approaches use pre-defined dictionaries of words associated with positive, negative, or neutral sentiments. For instance, words like “love” and “excellent” are typically positive, while “hate” and “awful” are negative.
Real-Life Applications
Sentiment analysis has proven its worth across various industries. In marketing, companies use it to monitor brand reputation and tailor campaigns based on customer feedback.
In finance, it influences trading decisions by helping investors gauge market sentiment from news articles and social media posts. On a smaller scale, businesses can use linguistic analysis to better extract invoice data and add a verbal elaboration to their financial moves and accomplishments.
Trend Prediction: Forecasting the Future with Linguistic Insights
Linguistic analysis also offers a unique lens through which we can anticipate emerging trends by studying language patterns across various text sources. By analyzing how certain words, phrases, and topics evolve, organizations can identify and navigate shifts in consumer preferences or societal changes before they become mainstream.
This proactive approach to trend prediction enables businesses and policymakers to stay ahead of the curve and make strategic decisions based on forward-looking insights.
Techniques and Approaches
Trend prediction using linguistic analysis relies on several techniques. One of these is topic modeling, which involves identifying clusters of related words that frequently appear together in large datasets, revealing what people are talking about most. Clustering methods group similar conversations together, helping to spot nascent trends.
Additionally, sentiment analysis can be combined with these methods to understand the emotional undertones of these trends, providing a more comprehensive view of the future landscape. Advanced natural language processing techniques can even detect subtle changes in word usage that might signal a shift in public perception or interest.
Applications in Various Sectors
Various sectors have embraced linguistic analysis for trend forecasting. In fashion, companies use social media analysis to predict upcoming style trends, allowing them to adjust their inventory accordingly.
Similarly, tech giants like Google analyze search queries to predict technological trends and consumer interests, while political campaigns analyze social media discourse to gauge public opinion and predict election outcomes. Even in healthcare, linguistic analysis of medical literature and patient forums helps predict emerging health concerns or treatment preferences.
Comparative Analysis for Decision-Making
Some companies are exploring new purposes for linguistic analysis, such as comparative analysis of huge documents. The techniques used for these comparative analyses include named entity recognition (NER), text classification, semantic similarity analysis, and information extraction.
Applying these linguistic analysis techniques to dense, technical documents allows businesses to quickly extract and compare key information, streamlining complex decision-making processes.
This is especially important when choosing vendors or third-party software, mainly at a time when cloud costs are rising, AI bandwidth is becoming more resource-intensive, and as a result, almost every SaaS provider is looking to upsell their services.
In this context, companies can use comparative analysis to compare prices of different cloud services through linguistic analysis of T&C and user guidelines docs, allowing decision-makers to make the right call without wasting time on too much research.
Another example, albeit a bit more high-stakes, is in the pharmaceutical industry, where companies use linguistic analysis to quickly compare clinical trial reports and scientific literature.
Customer Behavior Insights: Understanding the Consumer Mindset
In today’s competitive marketplace, understanding customer behavior is the cornerstone of business success.
Knowing what drives customers’ decisions, preferences, and actions allows you to anticipate needs, personalize experiences, and build lasting relationships with your audience. Linguistic analysis offers an in-depth look into the consumer mindset that goes beyond traditional data points.
Linguistic Markers in Customer Feedback
Linguistic analysis goes beyond surface-level feedback to uncover the underlying emotions and intentions of customers. By examining linguistic markers – such as word choice, tone, and sentence structure – businesses can gain deeper insights into how customers truly feel about their products or services.
For instance, the use of first-person pronouns might indicate a more personal connection to the product, while future-tense verbs could suggest anticipation or an intention to purchase.
Similarly, the use of certain adjectives or repetitive phrases can signal levels of satisfaction, frustration, or confusion, offering valuable clues into the consumer mindset.
Sentence structure can also be telling – short, fragmented sentences might indicate frustration, while longer, more elaborate responses often signal engagement and thoughtfulness.
Practical Applications
Linguistic analysis in understanding customer behavior has vast practical applications. For example, e-commerce platforms use this approach to refine product recommendations by analyzing customer reviews and feedback.
Similarly, marketing teams tailor their campaigns based on the emotional tone of customer conversations, leading to higher engagement and conversion rates.
By decoding their customers’ language, companies are not just reacting to expressed needs – they’re anticipating desires, addressing unspoken concerns, and creating more meaningful connections with their audience.
Wrapping Up
Linguistic analysis and semantic technology are revolutionizing how we interpret and leverage data. It’s transforming raw text into a goldmine of insights, unveiling consumer sentiments, predicting trends, and decoding customer behavior with unprecedented accuracy.
This powerful approach is bridging the gap between the vast sea of unstructured data and actionable business intelligence. Looking ahead, the integration of linguistic analysis with data science promises even more exciting possibilities.