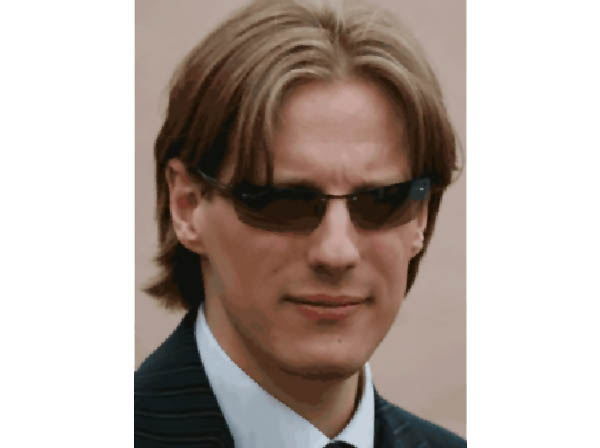
Click to learn more about author Christophe Antoine.
Analysts predict the big data market will grow by over $100 billion by 2025 due to more and more companies investing in technology to drive more business decisions from big data collection. However, companies who don’t adopt well-thought-out big data quality strategies will waste their investment because they won’t leverage the information stored in their data critical to making business decisions.
Currently, fewer than one-third of major enterprises can turn data into actionable insights. That’s because, for decades, managing and using data for analysis has been focused on the mechanics: collecting, cleaning, storing, and cataloging as much data as possible, then figuring out how to use it later.
Poor data insight has given way to an even more pervasive issue: poor data health. The abundance of data that exists today makes it harder to sort through the chaos and is causing companies to drown under a digital landfill of corporate information. Companies don’t know what data they have, where it is, or who is using it, and, critically, they have no way to measure their data health.
Setting a Data Quality Standard
Companies in every industry are dependent on their data to provide customer insights, identify new opportunities, and create untapped revenue streams. In big data architecture or collection, the essential piece isn’t the technology, the platform, or the volume of the data but rather the value you get out of data. This value is often hard to determine and score. The ability to score data value is becoming more critical, thanks in part to general practice to determine the accuracy, pertinence, and completeness of the data.
Having data issues in your chain can cause improper decisions, missed opportunities to maximize business, and misinterpretations or misconceptions about your business. Data Quality is essential to data health. Data Quality covers the discipline, methodology, techniques, and software that counteract these issues. The first step is establishing a well-defined and efficient set of metrics that allow users to assess the quality of the data objectively. The second is to prevent quality issues in the first place and improve the data to make it even more effective for its intended use.
Data Quality needs to be a company-wide priority, so data analysts don’t face the challenges of combining disparate sources and instead focus on driving essential decisions. Start by asking who will consume the data on your platform and how frequently and when they will consume it. It is also important to determine the short-term and long-term goals and benefits of the data you collect before you begin collecting it. Once you have an established and specific process for your data scientists, refine and examine the Data Quality and data value. The overall health of your data will guide you in determining how much you need to invest in technology and infrastructure. Data also needs to be traceable, ensuring you have Data Governance to manage data security and privacy. Finally, automate as much as possible, but make sure there’s human analysis of the data to provide deeper insights down the road.
Data Quality assessment must be a continuous process, as more data flows into the organization all the time. However, don’t view Data Quality only through the lens of assessment. Organizations should look at Data Quality as an opportunity for continuous improvement. Reacting to problems after they happen is costly. Organizations that are reactive instead of proactive will continue to suffer questionable decisions and missed opportunities. Systematic Data Quality assessment is a big step toward avoiding bad decisions and compliance liabilities, but it’s just a prerequisite. Continuous improvement is the endgame.
Ensuring Healthy Data
For years, we’ve treated data as simple, concrete units – cells on a spreadsheet, fields in a database, passive digital objects waiting for an analyst. But that’s no longer a sufficient model. Data is complex and constantly changing. New inputs flow in and out, updated by users and transformed by shifting contexts. Those inputs and actions both provide an opportunity to learn about and change the value of the data itself. To truly understand our data, we need a more responsible, holistic view of that data.
Every organization has its unique requirements, regulations, and risk tolerance. Data health is different for companies of every age, life stage, and maturity level. There are four primary focus areas to establish data health: reliability, visibility, understanding, and value. The industry needs a universal set of metrics to evaluate the health of data and establish it as an essential indicator of business strength.
By establishing a culture of continuous improvement backed by people equipped with the best tools and software available for Data Quality, we can protect ourselves from the most significant and most common risks. If we embed quality functionality into the data lifecycle before it enters the pipeline, while it flows through the system, and as analysts and applications use it, data health can become the norm – just like big data collection and storage are.