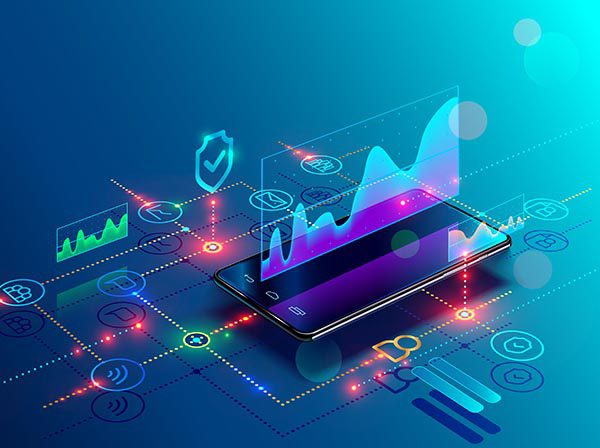
Data lakes were created in response to the need for Big Data analytics that has been largely unmet by data warehousing. The pendulum swing toward data lake technology provides some remarkable new capabilities, but can be problematic if the swing goes too far in the other direction. Far from being at the end of this evolutionary process, we are in the middle of it, said Anthony Algmin, CEO of Algmin Data Leadership, during his presentation titled Data Warehouse vs. Data Lake Technology at the DATAVERSITY® Enterprise Analytics Online conference.
The data warehouse, formerly the standard source for business insights, faces serious competition from the data lake. Providing ad hoc analytics, storage for unstructured data, and unparalleled scalability, data lakes are able to handle today’s data fast-growing data demands, but they have their limitations. Algmin compared data warehouse and data lake technology and their respective roles now and going forward
The Need for Big Data Analytics
Historically, as businesses began to rely more and more on computing technologies to do things from an operational perspective for the business, those systems and the data in them became isolated islands of business productivity. Demands arose to combine data with other functions, and CRM, HR, and ERP systems were created. These systems worked alongside operational-specific systems that were functionally oriented, reporting about their respective areas. “Yet when it came to analytics — even across different areas of the ERP system — [analytics] were added on as secondary thoughts,” without integrating external systems, Algmin said.
Organizations began to see the value of analytics and wanted reporting that included data in silos across the entire system, yet none of the existing applications were able to go beyond their own datasets. This led to homegrown solutions, such as large spreadsheets with multiple data sources copied into different tabs.
Algmin said that for many organizations, a point-to-point solution that “mashed together” different source systems to accomplish needed analytics is still in use today. There’s nothing wrong with that process, per se, he said, but it doesn’t scale very well. That need for scale led to the idea of the data warehouse, with a top-down approach to data modeling to create systemic conformity across data sources.
The Data Warehouse
The traditional data warehouse relies on relational database structures, where everything has its place. A dependence on dimensional modeling allows for intuitive data consumption and consistent performance. “Even to this day, if you’re doing operational reporting targeted to a particular process, your operational system’s probably pretty good at giving you insights about itself.”
Weaknesses include the need for tightly coupled relationships, complex processing logic, and the relative difficulty of change. As ETL processes (Extract, Transform, and Load) increase in complexity and data sources start to evolve, it becomes difficult to change, difficult to monitor, and difficult to troubleshoot. “You’ve got two numbers that should be the same, but they’re different. Why are they different? That can be quite an adventure to figure out,” he said.
The Data Lake
As volumes move past the terabyte range to the petabyte range and exabyte range, the traditional data warehouse is overwhelmed. Enter the data lake: a storage repository that holds a vast amount of raw data in its native format until it’s needed. Data lake technologies can scale to massive volumes of data, and combining datasets is easy with data stored in a relatively raw form. The process of data sharing allows patterns to emerge, providing a launching point for data warehousing, data marts, and a wide range of analytics capabilities. Although the focus has shifted from data warehousing to data lakes, “Data warehouses are probably more useful than they’ve ever been,” Algmin said, and the ability to create new data warehouses is unparalleled.
Weaknesses of Data Lakes
Data lakes have problems if growth is unchecked, requiring controls not needed by the data warehouse, Algmin said. Strong Metadata Management is critical or data use will become difficult and eroded. They also don’t work well in highly administrative environments because data lakes need a certain amount of individual freedom for it to be worth the effort of putting them together, he said. “The main challenge is not in creating a data lake, but in taking advantage of the opportunity it presents.”
The most effective data lakes make it easy for any data consumer to understand and find exactly what they need without help. “You do not want to have to go through an IT ticketing system just for a person to be able to use a new dataset,” he said.
Success Depends on the Basics
Metadata Management is how we tell the story of data, said Algmin, providing context and answers about the source of the data, its usefulness, its quality, and its meaning. Most organizations still struggle with Metadata Management, even with relatively small amounts of data. Without strong Data Governance and Metadata Management, a data lake is destined for failure, he said, and organizations that have struggled to realize the potential of their data lakes have even considered abandoning them to return to warehousing.
The massive scale of the data lake, “acts as an amplifier of those bad practices, which makes the mess even worse, and everything gets harder, not easier.” Without fundamental Data Management components, “we’re not going to be successful with data lakes,” he said.
The Future
Rather than serving as a single source of the truth, the data lake can serve as a collection of all truths with multiple perspectives, with the potential to evolve into open access data libraries. With scalability and extensibility prioritized over structure and control, the data lake reflects cloud’s core values and capabilities.
As data consumers refine and analyze data, the discoveries and insights they find can be put back into the data lake so they are available to other data consumers, to create “an engine of data improvement and data analytics capability that has never before been seen.” The critical process making the data lake more relevant is to complete that feedback loop, to use data, understand it, analyze it, make it better, and then return it, so other users can take it even further, he said.The business can evolve into using what Algmin calls a “smarter data lake architecture.”
Data Architecture used to be confined to the data warehouse, but now components can be swapped around as cloud opens up options for ephemeral data warehousing, he said. With technologies that can query data lake data directly, a database or visualization tool is not needed and, as a result, he sees tremendous potential for the future. “We don’t need to go to ten degrees of structure for every single thing that we do, but we do need to have a baseline understanding of the story of this piece of data.”
Rather than focusing on data lake or data warehouse technology, “It’s about how to turn data into insights that drive meaningful business value.” Algmin cautions against assuming that this period of rapid change is at an end. Instead he predicts more complexity and faster change as data volumes increase. “So, go make an impact, find a way to use data to make your business better, and that will guide you on the right overall path.”
Want to learn more about DATAVERSITY’s upcoming events? Check out our current lineup of online and face-to-face conferences here.

Here is the video of the Enterprise Analytics Online Presentation:
Image used under license from Shutterstock.com