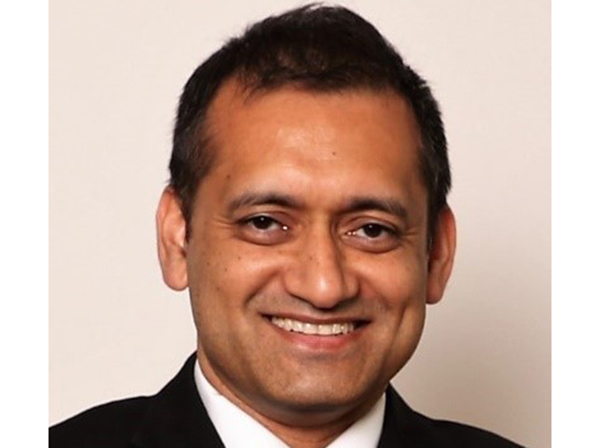
Click to learn more about author Gary Bhattacharjee.
After decades of languishing as the exhaust from the business processes, in the last decade, “data” has earned its rightful place as an asset of the business. However, for the most part in a data economy, it is a loss-leading asset, meaning while it is invaluable to conducting and competing in business, the upkeep and value of it are not directly realizable in a balance sheet unless the business itself is selling data.
In most enterprises, data is managed centrally by IT or at best by an operations cost center. No matter how deeply in sync this central organization is with the analytics needs of the business, in a rapidly evolving environment, it can sometimes take months to provide the right solutions, at the right time, and a miss can prove costly – especially if the business opportunity slips away. Bound by the limitations of legacy architecture, enterprises mostly have had little option but to follow a centralized model for data-powered analytics and insights.
With modern design patterns for managing and utilizing data in cloud ecosystems, experts predict that, in the coming months, 75% of centralized analytics initiatives will make way for a federated model where local business domain analytics units will come to share authority and decision-making with the central team. This implies not just the devolution of power, but also the evolution of analytics from a business support activity into a core business function.
Data and Analytics as DNA
To optimize a loss-leader asset like data, an enterprise should embed Data Literacy in every section of its business model. Thus, forward-thinking organizations have developed governance models that encourage “citizen data scientists” to innovate on data and analytics. This essentially translates into effecting a cultural transformation, so D&A is seen as a valuable resource owned and nurtured by the business.
How to Get There
A change of this scale needs to be driven from the top, with leadership responsibility extending all the way to tackling the challenges of operationalization.
Data-Native Teams
Extensive collaboration between diverse/cross-functional teams – both within the enterprise and in tandem with external ecosystems – is critical for ensuring the right resources are available to the program. For example, when Kraft Heinz decided to be “best-in-class” and change how the company performed analytics now and into the future, instead of cosmetic fixes, a radical step function meant moving to the cloud in a wholesale fashion and turning off the legacy applications. The vision was for a single data hub for the company that powers both the new digital transformation initiatives and the day-to-day analytics that run the company. They chose Snowflake for their data cloud.
It is also crucial to balance the IT and business roles, so that in allowing business teams greater autonomy, the expertise of the (erstwhile) central IT team does not go unutilized – for example, knowing how and where to deploy the most influential technologies like AI, IoT, mobility, cloud computing, robotic automation and, of course, various types of analytics.
Leaders need to ensure that their companies adopt data organization models that deliver enterprise, as well as domain-specific, objectives. There should be well-defined success metrics for process efficiency, savings, customer experience improvement etc., which must be connected with the data and analytics resources that produce these outcomes. An example is Amazon, which embeds engineers along with analysts inside business teams constituted to achieve specific business outcomes.
Making data sharing the default, rather than the exception, will ensure information is accessible when needed throughout the enterprise. However, data must not only be readily available, it also has to be of good quality – accurate, clean, not duplicated – and relevant. Good Data Governance takes care of this. However, as enterprises change their approach to data and analytics, they are also shifting to adaptive Data Governance, which recognizes that governance affects not just data initiatives, but the business as a whole. This ties in with another Gartner prediction, which says that by 2025, the bulk of Data Governance initiatives will focus on achieving business outcomes, rather than on conforming to data standards, which will be seen as essential capabilities.
When centralized analytics recedes, or disappears altogether, and decentralization elevates analytics and insights into a core business function, organizations will naturally evolve into truly data-driven enterprises.