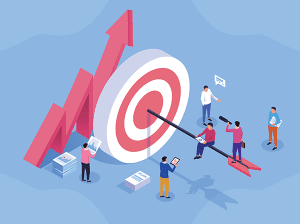
Data has become one of the most valuable assets of modern businesses. The data a company collects, analyzes, and monetizes is now perceived as a distinct “asset class” rather than merely a byproduct of its IT operations. However, the value of data to an organization diminishes rapidly as the information becomes less accurate. Companies are responding to the challenge of data accuracy by devising ways to maintain and enhance the value of information by keeping it fresh.
Ensuring the accuracy of data begins by identifying the characteristics of specific data elements that make them useful to the company. These data quality dimensions are unique to your business and reflect how your managers and staff gather, store, analyze, and protect business information to support decisions, measure performance, and track progress toward achieving your company’s goals. By measuring and sustaining the accuracy of data, everyone in the organization – from the executive suite to the shop floor – has more confidence in its planning and decision-making processes.
What Is Data Accuracy?
Data accuracy is measured by determining how closely information represents the objects and events it describes. A common example is the accuracy of GPS systems in directing people to their destination: Do they lead you directly to the doorstep, or point you to a spot a block or two away? For businesses, data accuracy relates to the information they collect, maintain, and use concerning their customers, products, and marketing and business intelligence operations. It applies whether the data is contained in a spreadsheet, database, or enterprise resource planning (ERP) system.
Data accuracy differs from data completeness, which refers to the extent of data coverage, such as accounting for all sources of incoming revenue in a comprehensive financial plan. Similarly, data accuracy isn’t synonymous with data quality, though the two concepts overlap. Data accuracy relates to the information being free from errors or mistakes, while data quality goes further in measuring how useful the information is to the organization, and how much value the company can realize from it.
Another concept related to but different from data accuracy is data integrity, which is defined as maintaining and guaranteeing the consistency, accuracy, and reliability of data. Data integrity verifies that the data hasn’t been altered or compromised and that it remains in the state it was in when created, transmitted, or stored. Preserving data integrity entails processes such as validation, access control, audits, and up-to-date backups. Both data accuracy and data integrity contribute to the data quality controls required to ensure regulatory compliance and overall data governance.
Why Is Data Accuracy Important?
The primary challenge of data accuracy is to verify the correctness of data values, confirming that the information is error-free and that it accurately represents the real-world condition it relates to. A common misconception in business is that the accuracy of data is static: If it’s accurate today, it will be accurate tomorrow. In fact, data ages rapidly and begins to lose accuracy from the moment of its initial capture. Data depreciation affects certain categories of data more than others and is especially important to marketers who must respond in near-real time to changing consumer preferences.
One way to measure the value of data accuracy to businesses is by considering the cost of inaccurate data. Gartner estimates that organizations lose an average of $12.9 million a year due to poor data quality. Those costs are likely to escalate as companies increase their reliance on AI and other data-driven technologies. Sophisticated machine learning models and other AI applications depend on accurate data for their training and continuous improvement components.
High data accuracy helps firms identify and respond to problems faster and more effectively. It lets them take better advantage of new market opportunities by getting the jump on the competition, and gain a clearer and more complete understanding of their customers’ tendencies and preferences. Among the data quality dimensions of data accuracy are timeliness (which may involve refresh rate and latency), attributes (consistency between data models and real world), usability (how easy it is to apply the data to enhance business processes), and reliability (whether stakeholders consider the data to be trustworthy and credible).
Examples of Data Inaccuracy
Data is considered inaccurate if it is factually wrong, but also if it is incomplete, imprecise, or ambiguous. As stated above, data may have been accurate when it was collected but rendered inaccurate with the passage of time. The data may also no longer accurately represent the real-world state it was created to represent, such as a census report on a city’s population. These are among the common sources of data inaccuracies:
- Manual data entry: Spell checkers and data validation rules can minimize the number of human errors made while entering data by hand, but the mistakes can’t be eliminated entirely. Data entry errors also occur as a result of OCR scans, incorrect data inputs and formatting, inadvertent deletion or duplication, and incorrect measurement or unit values.
- Lack of data standardization: Various groups within an organization may adopt different data formats, such as one formatting dates using 01-01-2024 format and another entering dates as “January 1, 2024.” Similarly, international firms may need to convert dates entered in their European offices as “DD-MM-YY” into the standard North American data format of “MM-DD-YY.”
- Data decay: While time is the primary source of natural data decay, the information may also become inaccurate or irrelevant as a result of internal changes to the company’s data systems. An example is a database entry pointing to a URL that has been changed as a result of a website update.
- Data silos: It isn’t unusual for the same data to reside in multiple locations within a single organization. When these locations are updated asynchronously, it renders the others inaccurate for however long it takes for the data values to be reconciled. Alternatively, an employee may enter a different data value in one system because the person wasn’t able to access the same data that had been entered into one of the company’s other internal systems.
- Poor data culture: Workers who haven’t been trained in appropriate data quality practices are likelier to introduce data errors into systems. Even when the repeated data is accurate, the duplication creates problems related to data consistency and integrity.
How Data Inaccuracy Impacts Businesses
Even the most important data has no value to a company until it is able to convert the information into revenue, whether directly or indirectly. Retailers can deepen their ties with their customers by learning about their interests and preferences, but an inaccurate reading of data can reduce the effectiveness of their personalization efforts. For example, if they track sales to a sports venue, they may infer that their customers are sports fans. However, the sales may have actually occurred during a non-sports event at the venue, such as a concert.
Raw location data is an example of low-quality information because it entails many fraudulent and duplicate signals. The data becomes high-quality only after it has been processed to remove the irrelevant signals, which may mean stripping away more than half of the raw data that was initially collected. Relying on low-quality data causes sales teams to waste time following poor leads and makes it more difficult to stay in contact with customers if the company’s CRM retains outdated addresses, phone numbers, and other personal information.
Perhaps the greatest risk to firms posed by data inaccuracies is causing poor business decisions. The accuracy of forecasts for sales, revenue, and other uncertainties relies on high-quality data, the absence of which leads to lost revenue through missed opportunities, reduced efficiency and productivity, and customer dissatisfaction. Inaccurate analysis of data can also cause reputational damage, endanger regulatory compliance, and increase operational costs unnecessarily.
Techniques for Overcoming the Challenge of Data Accuracy
The first step in improving the accuracy of your organization’s data is to devise a reliable method for determining the quality of data. Among the characteristics assessed by data quality metrics are relevance, factuality, timeliness, integrity, completeness, and consistency. These are some of the techniques for monitoring and evaluating data accuracy:
- Data profiling reviews and analyzes data to present a high-level overview of its accuracy.
- Outlier detection spots and removes data whose values vary significantly from the other data points in the set.
- Cross-field validation requires that a field use another field’s value to be validated.
- Data cleansing is sometimes referred to as data scrubbing and entails aggregation and auditing to verify the accuracy, completeness, consistency, and uniformity of data.
- Data integration and transformation uses extract, transform, and load (ETL) tools or other techniques to validate the accuracy of data at each step of the data pipeline.
- Data observability monitors data in real time to track its lineage, create visualizations of data relationships, trigger alerts when specific conditions occur, catalog data assets, and enforce data governance policies.
Data drives nearly every aspect of modern business. The more accurate the data that’s driving your operations, the better chance your company has of achieving its goals. By devising a comprehensive and agile data management and governance policy, organizations improve the accuracy of their analyses and forecasts, boost the efficiency of workers, and gain a competitive advantage by capitalizing on AI and other burgeoning technologies.
Image used under license from Shutterstock