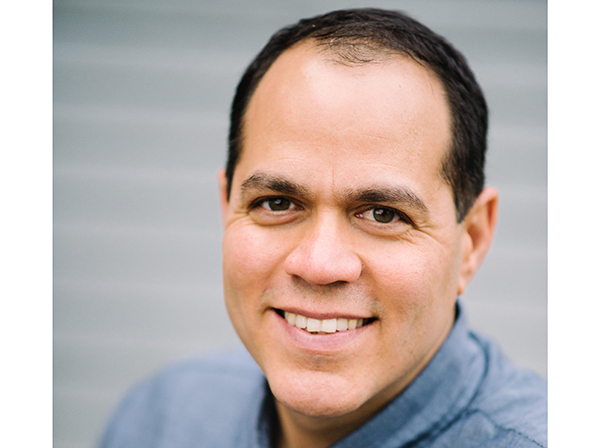
Synthetic data is at an inflection point of utilization. The emerging technology is just beginning its adoption cycle and value to the enterprise, but change is on the horizon. According to my company’s recent survey, industry leaders believe that, on average, 59% of their industry will utilize synthetic data in five years, either independently or in combination with real-world data. Many industries and companies are experimenting with the technology and recognizing the use cases and relevant applications.
While 2020 and 2021 saw the adoption of synthetic data by those in the machine learning and computer vision fields, 2022 will be the year synthetic data appeals more to the majority, ensuring they view its integration as critical to staying ahead.
Synthetic Data and Its Disruptive Capabilities
Synthetic data is computer-generated data that serves as an alternative to real-world data. It is created in simulated digital worlds rather than collected from or measured in the real world. Combining tools from the world of visual effects and CGI with generative AI models, synthetic data enables companies to create vast amounts of photorealistic, diverse data on demand to train computer vision models.
Synthetic data is a disruptive approach to training AI models through the use of computer-generated images and simulations. A single dataset may contain tens of millions of elements. To manually collect and label data of this magnitude is time-consuming and costly for organizations, not to mention prone to human errors. Synthetic data aims to simulate real-world scenarios to train AI systems virtually. This approach reduces the time and resources needed to build these models by delivering vast amounts of perfectly labeled data to organizations in a matter of hours. Also, since synthetic data isn’t generated from real-world sources, data privacy and bias issues are reduced. According to the aforementioned survey, 89% of technology executives agree that it is a new and innovative technology that will transform their industry.
Barriers to Further Adoption
While synthetic data can transform industries and how organizations use data, there is still work to be done to address barriers to adoption. Two-thirds (67%) of technology executives agree that their organization lacks the knowledge and understanding of implementing it. Additionally, almost half have concerns that models built with synthetic data are not as good as real-world data.
A key to further implementation is educating colleagues throughout the entire organization, not just the C-suite, as there is confusion and a lack of understanding among many groups. Organizations already using vision data are positioned to lead this charge, as they understand the value of vision data and how it can benefit their industry. Among those working with vision data that don’t use or have only started using synthetic data, only three in 10 (30%) respondents cite a lack of tools to create and manage synthetic data as a barrier to broader utilization.
Synthetic Data Enabling Emerging Industries
AI is driven by the speed, diversity, and quality of data. Today’s systems leverage “supervised learning” approaches in which humans label attributes in image data to then train AI models. This approach is fundamentally limited, as humans do not scale and, more importantly, cannot label key attributes (e.g., 3D position, interactions, etc.) necessary to enable emerging industries such as AR/VR, autonomous vehicles, robotics, and more. Synthetic data is predicted to be a key solution to address these shortcomings to bring these emerging industries to the mainstream.
The metaverse, for example, cannot be built quickly or efficiently without the use of synthetic data. To recreate reality as a digital twin and build out enough 3D-rendered objects in a way that is time- and cost-efficient, it’s necessary to deeply understand humans, objects, 3D environments, and their interactions with one another. Creating these AI capabilities requires tremendous amounts of high-quality labeled 3D data – data that is impossible for humans to label.
Synthetic Data’s Inevitability
According to “Synthetic Data for Deep Learning,” new research is starting to provide proof points around the utility of synthetic data across use cases, including robotics, autonomous vehicles, smart homes, consumer products, manufacturing, logistics, health care, and more. These use cases and the growing buzz around other emerging industries will be central to the growth of synthetic data because simply put, they won’t be possible without it. Synthetic data will inevitably come to define a new paradigm in AI and enable the next generation of more capable models and products in 2022 and beyond.