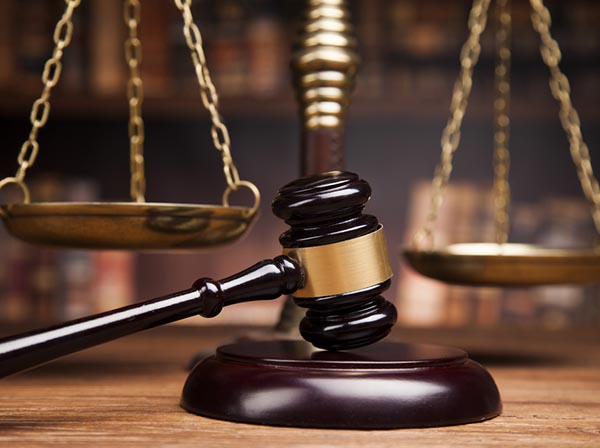
Order in the court! Order in the court!
The case being heard is a serious one. The defendant—the Chief Data Architect of VeraVisionFake Inc.—is accused of having ignored the need to establish a valid and sound common data vocabulary in her organization. She is up against The Common Vocabulary Value regulatory agency, whose mission is to make sure that organizations have defined at least a minimum common vocabulary—shared terms and definitions that identify and describe data items.
The judge and plaintiff enter the courtroom garbed in white wigs and black robes. The action gets underway.
You’ve probably figured out that this is a mock trial – it was titled You Are Liable for Not Establishing a “Common Vocabulary” in Your Organization!and was staged at the DATAVERSITY® Enterprise Data World Conference. Its purpose was to reflect the struggles of those who have to develop a common data vocabulary—and the price they might pay for failure—in a witty and amusing way.
It starred Thomas C. Redman, head of Data Quality Solutions, as the judge; Len Silverston, President of Universal Data Models, as the plaintiff; Danette McGilvray, President and Principal at Granite Falls Consulting, as lawyer for the defense; Laura Sebastian-Coleman, Data Quality Lead at Aetna, as the defendant; Ron Klein, Global Data Governance Leader at Royal Bank of Canada, as the clerk; and Katherine O’Keefe, Director or Training and Research at CastleBridge, as the expert witness.
Silverston made the case that defendant Sebastian-Coleman gave up on her duty of establishing a common vocabulary, one of her highest priorities. That led to VeraVisionFake being out of compliance with GDPR, which exposed thousands of people to identity theft, and the company to a $200,000 GDPR fine as its share price dropped 20 percent.
McGilvray’s opening argument was that it was a mistake to think that one person can build a common vocabulary on their own; business metadata is not a one-person job but requires organizational commitment, she said.
An expert witness for the plaintiff testified that the defendant’s lack of defining a common vocabulary meant that the business could not properly identify data at risk in the organization and that workers became very confused about who to call and how best to contact appropriate parties when needed. The defendant, she said, failed in her fiduciary responsibilities.
Sebastian-Coleman, the only data architect at the fictional $1 billion company, admitted that she did have responsibility to build a common vocabulary in nine months—using Excel—but found herself pulled into other jobs. IT and business people were at her back, not recognizing there was a process problem and a culture one, too.
The jury (AKA the audience) voted that she was not liable, leading to clapping and cheers from the sympathetic attendees. Redman weighed in that he thought she was guilty—but of a lack of imagination as evidenced by her failure to see that standard approaches to Data Management just don’t work. What she should have done, he said, was to be far closer to the business—even integrated with it. His recommendation: Over the next 100 days, hold one-one-one meetings with business counterparts up and down the organization. In his summation, he explained that the idea of taking this approach was to inspire those in Data Management to aim for a higher standard: get systems to talk by getting people to talk.
Onto the Leaders’ Data Manifesto
It was a good follow-up to the earlier session on the Leader’s Data Manifesto. The latest incarnation of the Manifesto builds upon earlier versions, urging that anyone who needs data to do their job pick an area that interests them (Data Quality, for example) and find and talk to the people who are responsible for that area, starting small and making definitive improvements.
It remains a call for data professionals to build connections with business counterparts, learn to speak their language, and help them to succeed with data. “Be a data provocateur,” said Thomas Redman, one of the principals behind the Manifesto. “Lead change. Do more, do it bigger and do it faster.”
This time there is a sharper and narrowed focus on the notion of Data Quality and machine learning. “The cold, brutal reality is that the data is not good enough to support machine learning in practically every company,” said Danette McGilvray, also a leader behind the Manifesto. “We are headed for epic fail.”
The call is going out to Manifesto signatories to get onboard with that agenda. That means homework, which is for data pros to reach out to people in the organization in different ways and to work collectively together on how to stimulate change in the machine learning area, Redman said—before an epic fail.
John Ladley, Chief Delivery Officer at advisory firm First San Francisco Partners and also a Manifesto principal, added that it’s important to be specific in talking to people about how data can help with machine learning. Ask, for example, “Have you looked at Data Quality as you are doing this?”
Further Thoughts on Data Quality and Machine Learning
Redman spoke further about machine learning, Data Quality, and the need for data and business pros to communicate, in an interview conducted by DATAVERSITY. Regarding machine learning, he pointed out that everyone is interested in building algorithms—but to train them effectively, high-quality data, and a lot of it, is important.
“It must be accurate and unbiased before the machine learning model is introduced,” he said. “Bad data does dumb stuff—like in criminal justice, the idea was to remove bias in sentencing, but the model was trained on data where bias was built into sentencing.”
Also, a Data Quality problem is that people tend to think of it as being what you get after you clean data. “But,” said Redman, “it’s easier not to make mistakes in the first place.” A fundamental problem is that more mistakes are being made that then have to be cleaned up—and the fact is that that data may be cleaner but not clean.
Nonetheless, he commented:
“I’m excited about using machine learning to attack problems we haven’t been able to using traditional means. Most encouraging is a thousand points of light, where [machine learning] is used to help people figure out how to do better hiring or drilling for oil better or giving better advice to financial clients.”
Want to learn more about DATAVERSITY’s upcoming events? Check out our current lineup of online and face-to-face conferences here.

Here are the videos of the Enterprise Data World Presentations:
Image used under license from Shutterstock.com