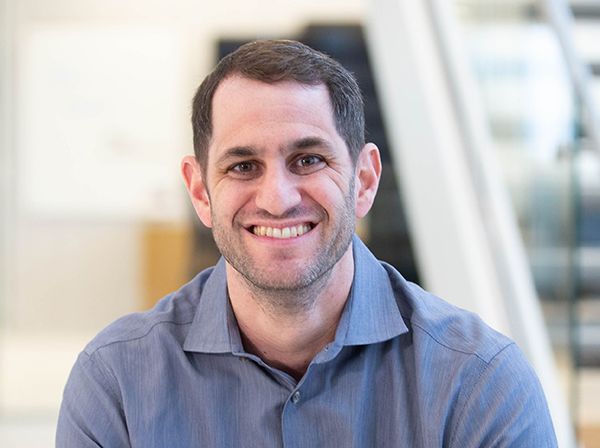
The list of use cases powered by artificial intelligence (AI) and machine learning (ML) technologies is growing exponentially across nearly every business sector. Enterprises of all kinds are leveraging these advanced capabilities and scaling them through automation to improve business process management, sharpen organizational strategies, and reap more analytical and predictive insights from data for decision support.
However, in order to avoid operational and security problems and achieve sustainable ROI on their AI and automation investments, organizations must first have a solid data management and optimization strategy in place. Let’s examine why developing this strategy is such a foundational step in establishing AI and other advanced technologies as drivers of stronger value and performance in the enterprise.
AI and Automation Can’t Succeed on a Shaky Data Foundation
AI and ML are revolutionizing how organizations design, manage, and continually improve business operations, especially when combined with automation to eliminate manual tasks and scale complex processes. These deployments are fueling some of the most advanced and impactful use cases – from predictive analytics to intelligent recommendations, knowledge assistants, and the rise in AIOps applications that help automate and streamline operational workflows.
The caveat is that the effectiveness of these technologies depends on the integrity of the underlying data layer in the organization. If you don’t have standardized, well-integrated, easily accessible, and sovereign data, then your AI platform can’t properly enrich and augment the data; you can’t adequately train the models. Your AI will churn out answers that it feels confident about, but which are dead wrong. It’s a modern version of the age-old “garbage in, garbage out” scenario – resulting in bad search results, bad extraction, bad classification, and bad predictions.
The picture is equally troubling when it comes to automation. Trying to automate without clear definitions and access rules for your data will result in costly, slow, and incomplete processes. When the data itself is fragmented and duplicative, with no clear governance and no alignment of standards, then bringing in automation under those conditions is simply scaling the dysfunction. You’ll also be scaling the inefficiencies, errors, blind spots, instability, and even security issues that are caused by the underlying challenge of a poor data strategy.
Designing Your Data Management and Optimization Strategy
The combined impact of the above pain points makes building AI and automation on a shaky data management foundation like building a house without a solid foundation or consistent architectural standards. In each case, it’s a costly and ultimately unsuccessful investment that results in a poorly functioning, substandard outcome.
Fortunately, the right data management and optimization strategy can bring consistency and order to the underlying data layer, providing a coherent and comprehensive framework for AI/ML and other advanced technology platforms to operate. While the specific strategy will likely vary for each organization depending on the structure of the operation and the nature of the data, a few common objectives should remain at the heart of every strategy.
Rationalizing data, including establishing common standards for metadata, business context, and interoperability, is a critical priority. It’s not uncommon to see definitions and data attributes – such as what constitutes a “customer” – fragmented and scattered across various disconnected enterprise systems. An effective strategy will rationalize to ensure consistency of these values, whether a database is located in the accounting, marketing, CRM, shipping fulfillment, or other system. This allows an AI/ML platform to compare apples to apples when pulling from different data sources to perform instant computations, do advanced analysis, or carry out AIOps functions like alert management or authentication tasks in real time.
Another strategic focus of the plan should be on ensuring reliable and performant access to the data systems necessary to support AI-powered applications. The plan should thoroughly map where all data is stored and ensure access is secure and streamlined for both human users and automated authentication protocols used by AI systems. This reduces knowledge gaps and latency issues that can slow down AI/ML platforms and reduce their effectiveness – particularly in scenarios where real-time analysis, time-sensitive decision support, or AIOps automation are required.
Conclusion: Implementing the Right Data Strategy for AI and Automation
A well-designed data management and optimization strategy will improve AI/ML operations and ROI while minimizing risks from inaccuracies, knowledge gaps, reporting omissions and other security and compliance breakdowns that can lead to cyber attacks, downtime, reputational damage, and regulatory fines. A key priority to remember during implementation is that a solid data management and optimization strategy will, itself, need to be founded on the right underlying data architecture.
Several agile data frameworks – including data lakes, data mesh, and data fabric architectures – are designed to break down silos and better connect data. Among these options, data fabric is the gold standard. It works by eliminating ETL by instead accessing data where it resides – and then adding an abstraction layer to virtualize an enterprise-wide view of data that’s aligned and contextualized for the AI/ML platform to work with.
While all these steps require strategy, investment, and workforce training to smooth the adoption curve, the ROI is well worth the effort in the form of a strong data strategy and architecture that reaps maximum performance and value from enterprise AI and automation.