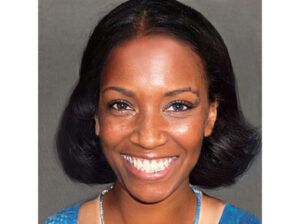
As organizations scale, data debt can accumulate silently, affecting productivity, efficiency, and decision-making quality. Data debt refers to the accrued cost of shortcuts and inefficiencies in data management that may have once seemed expedient but ultimately hamper long-term growth.
Over time, whether it’s due to process changes, platform switching or growth, organizations find themselves boggled down in plenty of data dead ends. Hence, addressing data debt in its early stages is crucial to ensure that it does not become an overwhelming barrier to progress.
Understanding the Components of Data Debt
Data debt can take many forms, from inconsistent data standards and incomplete documentation to fragmented systems. This debt accumulates as organizations make short-term decisions without adequate thought to scalability.
Likewise, data models that worked when the company was smaller can quickly become untenable when an influx of data from new customers, products, or markets overwhelms systems designed for a different era. That’s why recognizing these components is the first step to building an effective strategy for managing data debt.
One of the major challenges in growing organizations is that legacy decisions – ranging from temporary data storage solutions to poor metadata practices – often become permanent fixtures. As data volume and complexity grow, these ad hoc solutions form the backbone of data debt.
It just goes to show that every data management practice must be implemented with long-term feasibility in mind.
Prioritizing Debt Through Impact Assessment
Not all data debt is created equal. Growing organizations experiencing data sprawl at an expanding rate must conduct a thorough impact assessment to determine which aspects of their data debt are most harmful to operational efficiency and strategic initiatives.
An effective approach involves quantifying the potential risks associated with each type of debt – such as compliance violations or lost customer insights – and calculating the opportunity cost of maintaining versus mitigating them.
For example, debt that directly impacts customer interactions should often be prioritized, as it can influence customer satisfaction and retention. Likewise, data debt affecting financial processes may require immediate attention since it hinders critical functions like timely payment and cash flow analysis.
The same goes for usage data from iOS or Android apps, or even the performance of certain marketing campaigns or promotional efforts. Finally, it’s essential to recognize the importance of proper data labeling, categorization, and annotation.
Establishing Data Governance and Standards
A core approach to managing data debt is to establish strong data governance practices that address inconsistencies and fragmentation. Before anything else, you must establish an adequate access control system and ensure its imperviousness.
Next, you must think about implementing robust validation mechanisms that will help prevent further debt accumulation. Data governance frameworks provide a foundation for minimizing ad hoc fixes, which are the primary drivers of data debt.
That’s why, as a growing organization, investing in policies that ensure data quality – such as regular audits and strict adherence to data integrity rules – is key to tackling both existing and future debt.
Automation and Workflow Management
Automation can be one of the strongest allies in data management, effectively combating data debt. By automating manual, error-prone processes—like data entry and reconciliation—organizations can eliminate opportunities for human error and prevent the introduction of poor-quality data into their systems.
Even laypeople in teams can grasp this concept, mainly because 77% of all devices now feature some form of AI, which led to a rise in no-code solutions and a more practical outlook towards data. Likewise, organizations should also consider automating routine data clean-up processes.
Regularly scheduled cleaning scripts that identify duplicate entries or incomplete records can help prevent issues from piling up. Automation in this context isn’t just about improving efficiency; it’s about ensuring that data problems do not reach critical mass.
Investing in Scalable Data Architecture
Short-term, cost-effective solutions can be a tempting way to address immediate data needs, but they often lead to structural weaknesses as the organization grows. Investing in scalable data architecture means planning for growth from the outset.
In particular, data lakes and data warehouses should be designed with elasticity in mind – ensuring they can handle not only current data loads but also future growth without major overhauls.
An architectural shift that facilitates scalability can help avoid the bottlenecks that arise when data outgrows its infrastructure. Technologies like cloud platforms offer scalability without heavy up-front investments, allowing organizations to expand their capacity in line with their growth.
Training and Awareness
Somewhat surprisingly, data debt often results not from malice but from misunderstandings or the lack of clear communication about data best practices. It occurs when employees who are unaware of proper data entry standards or who cut corners when documenting processes can inadvertently contribute to growing data debt.
That’s why establishing a culture of accountability by providing targeted training is key to managing data health over the long term.
It requires making sure that teams understand the downstream impact of their data-related actions to help reinforce the importance of good practices. Employee awareness initiatives can also help team members know what to do when they’re getting hacked, as data vulnerabilities often stem from poor data hygiene, like weak password protocols or unsecure data sharing.
Building a Business Case for Data Debt Reduction
Securing buy-in from stakeholders for addressing data debt requires framing it in a way that aligns with broader business goals.
Data debt is not just an IT concern; it affects customer experience, operational efficiency, and financial outcomes. Making a clear case to stakeholders about the benefits of addressing data debt – such as faster decision-making, increased compliance, and reduced costs – can lead to the allocation of necessary resources.
Demonstrating a return on investment for tackling data debt, including faster customer response times or lower maintenance costs for data systems, will help stakeholders see why reducing this debt matters. One approach is to tie data debt reduction directly to customer pain points, such as inconsistencies in service quality.
Monitoring and Iteration
Reducing data debt isn’t a one-off project; it requires continuous monitoring and adjustment. Even the smallest lapse can result in issues down the road.
As the organization grows and evolves, new forms of data debt can emerge, and strategies must evolve accordingly. Implementing regular reviews and health checks ensures that data quality stays consistent, avoiding the need for major interventions down the line.
Data observability tools can help track key metrics related to data debt, such as the volume of redundant data or the frequency of data inconsistencies. These insights are crucial for taking proactive measures before data issues begin to hinder strategic goals.
Conclusion
Data debt is an inevitable consequence of growth, but proactive strategies can prevent it from becoming a major bottleneck. By understanding the different forms of data debt, prioritizing high-impact areas, and investing in scalable systems and automation, organizations can keep their data assets healthy.
Successful data management is a combination of culture, tools, and architecture – ensuring the organization remains agile and capable of leveraging its data, rather than being hamstrung by it. The sooner you tackle your data debt, the more efficient your data management practices will be.