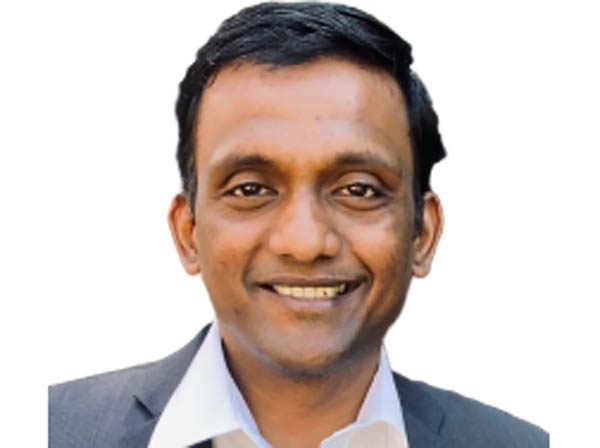
Click to learn more about author Balaji Ganesan.
As the song goes, “…the more things change, the more they stay the same;” the same holds true for the IT industry. Data warehouses began to appear on the IT scene in the late 1980s as a decision support system used for reporting and data analysis. These centralized data repositories were designed to integrate data from multiple disparate data sources and operational systems, such as sales, marketing, or service. Organizations primarily used data warehouses to provide a 360-degree view of their customers, integrating various disparate pieces of information from sales, marketing, and services to provide a comprehensive customer profile.
Today, companies are migrating their data from on-premises data warehouses, data lakes, and operational systems to take advantage of the lower cost structure and resource elasticity of cloud-based repositories. However, the leading cloud providers offer a dizzying array of services to store and analyze data (e.g., AWS, Azure, and Google Cloud collectively offer 70-plus services in the categories of storage and analytics alone).
If a company selects one of the public clouds as its exclusive cloud infrastructure, it is quite probable its data is still spread or duplicated across the various services it subscribes to — which can be further complicated by third-party services, such as Databricks and Snowflake, that operate on top of the public clouds. For example, if a company migrates its data to AWS, it is possible customer records, at the lowest level of granularity, are stored in S3 — or worse, the aggregated customer records could be stored in Redshift, Snowflake, or Databricks if the company plans to analyze the records.
Unlocking the Clues to the Data Privacy Scavenger Hunt
Data is scattered across multiple systems, services, and locations, resulting in a scavenger hunt for every data related query, change, and transformation. For example, when a company receives a request from a customer to delete all of their information from its records — as part of the right-to-be-forgotten provision of GDPR or CCPA — it forces data administrators to go on an unenviable scavenger hunt riddled with potential pitfalls.
Without an automated, comprehensive process in place to discover, classify, and manage sensitive data, organizations must manually access and analyze all data repositories to locate all elements of a customer’s record among the millions of locations it can be partitioned and stored. Under these conditions, the chance of missing one record among the many data stores is high, resulting in an inability to fully satisfy the customer’s request and potentially dire consequences from non-compliance.
To avoid this data scavenger hunt, enterprises need complete visibility into all the systems where customer information could be stored or analyzed. In order to fulfill such a customer request for the deletion of their data, a company needs a strong capability to scan its data for sensitive information such as personally identifiable information (PII) or other personal data as it is flowing across its cloud data supply chain.
The volume of data enterprises now manage is so massive, organizations are seeking, yet again, for technologies that can help them discover sensitive data with high enough performance, as well as match the agility and velocity associated with the data and their transformations with fidelity at an extreme scale. But to do so requires a variety of sophisticated techniques, such as rules, pattern matching, dictionaries, algorithms, machine learning, and natural language processing (NLP) based models.
The ability to discover sensitive pieces of data provides enterprises with the foundation to classify their data and apply suitable business context, such as classifications to data the system identifies as sensitive or PII. Similar to how airlines apply tags to luggage to ensure they reach their intended destination, these data tags follow sensitive information as it is stored, moved, transformed, or analyzed through the cloud landscape.
Once the process of identifying and classifying sensitive data has been completed, the information can be used to build policies that control the access of users within the enterprise, as well as other partners who have a need to use or process through data-sharing agreements. In such a complex, heterogeneous landscape, it is almost a given that each of the services from the major cloud providers (and third-parties) offers multiple, inconsistent, and fragmented approaches to managing users’ access to data.
For example, if a company’s data resides in S3, Snowflake, and Databricks, the data administrator must navigate to three separate interfaces and must be well-versed in the unique mechanisms to grant users access to that data at the precise level of granularity, so it can be appropriately shared with the right external or internal audience. As cloud services adoption grows within an enterprise, the number of failure modes and exposed surface of data makes it inefficient, if not impossible, to manage consistently. Data administrators need a centralized platform that provides the ability to build and administer access control policies from a single location for all the cloud services where their data is stored.
While many people are under the misconception that it is a simple task to grant access to sensitive data as a binary decision, in practice, it can be much more nuanced and based on complex conditions, usage limitations, data sharing agreements, etc. There may be nuggets of insight hiding in the sensitive data, or its context, for data analysts and scientists to understand and catalog without running afoul of any compliance or regulatory requirements. Therefore, enterprises need the ability to selectively grant access to sensitive data for analytic purposes by keeping it secured and “privacy-protected” at the same time.
The ability to de-identify data allows data analysts to enable protected access to sensitive data with a mask or a filter applied to the information where necessary, or selectively reveal data in the original form where necessary for appropriate usage scenarios. A scalable, effective framework is necessary to prevent your data administrators, data stewards, and compliance personnel from descending into this deadly scavenger hunt, while simultaneously balancing compliance with privacy and industry regulations, building trust with customers, and assuring their data is safe.