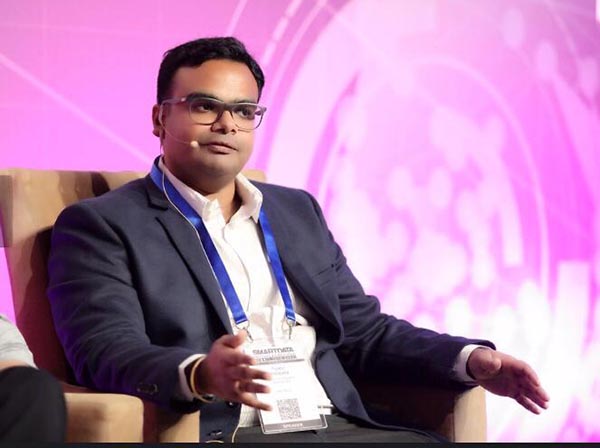
Click to learn more about author Tejasvi Addagada.
Today’s data landscapes in enterprises are increasingly based on core principles of Data Discovery, Right Data Interpretation, Coverage, Availability and Inter-operability.
Often the first step is knowing what data exists in our firms, while also interpreting it in the right way by managing the meaning. Business Intelligence often describes “Data Discovery” as finding insights from data, but I would rather state discovery as a first step to understanding and finding the basic data, its structures & movement.
As data is interpreted in the right way, by people; data can be “Actively managed by its meaning”. This is done through dictionaries & glossaries captured for metadata.
Having worked with firms, that went through acquisitions, I can state that “Coverage“ of the right data for Artificial Intelligence use-cases, is often a challenge.
For example, in an investment management firm, which has not gone through product rationalization; there can be different segments of customers as well as different sub-products associated. Not being able to aggregate customers and associated products, often render misleading outcomes or insights.
Even from IQ International, we have been pushing the industry to leverage “Availability” as a primary dimension of Data Quality, for AI & Analytics. Availability of data often becomes a challenge which leads to less impactful decisions and reduces the data advantage that the organization can have.
Data needs to be “Inter-operable”, required for process, systems or services to interface in the right sense & in an un-burdened way. Data inter-operability addresses the ability of services that create, exchange and consume data. Availability of standards internally and externally as well, make it a necessity for data inter-operability. We can quote some enablers like identifiers including LEIs, open banking & formats including ISO 11179 that define data harmoniously.