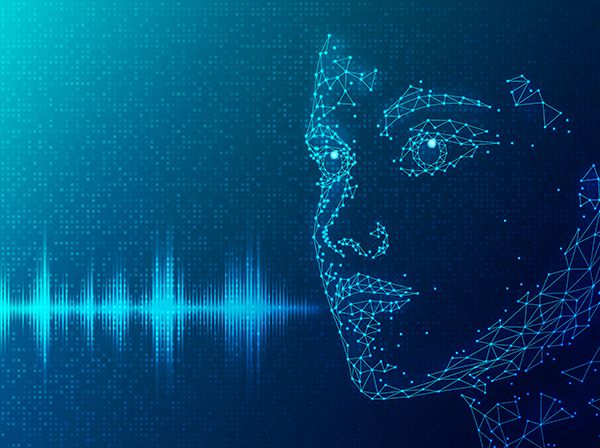
The trends of semantic technology are based on the desire to improve the computer’s understanding of data. The goal of combining semantic technology with a computer system is to discover relationships within the data. Semantic technology can be described as tools and methods that can process, categorize, and find patterns in the data.
Semantics is a subdivision of linguistics – the study of language and its structure. Semantics focuses on words and phrases, and how they relate to one another when communicating information. The technology uses semantics to apply meaning and context to the different kinds of data that are used.
Semantic technology focuses on the meaning of data, rather than the structure of the data.
Semantic technology links and defines data by building languages for computers that can provide rich descriptions of how the data is interrelated. These languages allow computers to process and interlink the data. It uses tools that have been around for some time. Examples of semantic technologies currently being used include:
- Classification: Classification technologies that use heuristics (mental shortcuts for problem-solving) and rules for tagging data to help with searching for and analyzing specific information.
- Semantic searches: Locates data by way of concepts, rather than keywords or key phrases.
- Natural-language processing (NLP): NLP technologies are used to process unstructured text, extracting names, organizations, dates, etc.
- Data mining: Uses pattern-matching algorithms to seek out patterns and correlations stored in large collections of data.
Semantic Technology Becomes a Trend
Until recently, many IT professionals considered semantic technologies to be a quirky and experimental form of Data Management – primarily because they didn’t understand it. The study of semantics is quite different from the development of software. Currently, however, the growing popularity of knowledge graphs combined with the evolution of artificial intelligence has promoted the use of semantic technologies.
According to Marco Varone, CTO of expert.ai,
“A lot of things are happening in the semantic language understanding space. Many more things have happened in the last three, four years than in the previous 10 to 15. In the last few years, the change has been from experiments in semantics and language to real projects.”
Semantic technology can index massive amounts of data and process very long strings of characters. The technology can also store, manage, and retrieve data by using meaning and presenting logical relationships. The use of semantic technologies with a variety of data processes has recently gained popularity. Some of the most recent trends include:
- Combining semantics technology and data fabric
- Knowledge graphs
- Data lakehouses
- Semantic technology and AI
- Conversational chatbots
Combining Semantics Technology and Data Fabric
The concept of data fabric is fairly new, and focuses on the idea that data can be taken from a variety of sources, and then woven together to create a reasonable model of reality. As businesses move to the cloud, the amounts of data they handle, and different types of data, continue to increase. The goal of data fabric is to seamlessly weave together data, making a business using that data more efficient.
Mark Beyer, an analyst at Gartner, said,
“The emerging design concept called ‘data fabric’ can be a robust solution to ever present-day management challenges, such as the high-cost and low-value of data integration cycles, frequent maintenance of earlier integrations, the rising demand for real-time and event-driven data sharing, and more.”
The data fabric model uses active metadata and will transform data into a standardized format before storing it. Data fabric uses metadata to locate and interlink the requested data files. By combining semantics technology with data fabric, an extremely effective research process can be developed.
Knowledge Graphs
Knowledge graphs connect a large number of data descriptions and a variety of generally useful concepts. When semantic technologies are combined with linked data technology, relationships between data of various formats and sources are formed, and help to create context. When interlinked in this way, these pieces of raw data form a weblike structure of data, or “a knowledge graph.”
The knowledge graph represents a model of interlinked information – a weblike collection of descriptions for concepts, entities, and relationships.
As we use the internet, we constantly interact with knowledge graphs (and their underlying graph databases) without realizing it. The use of knowledge graphs has been embraced by several business sectors, ranging from the auto industry to the oil industry to the pharmaceutical and health industry to publishing, etc. They are often used by streaming services to predict which movies and shows a person might enjoy. For those using virtual assistants (such as Siri, Alexa, or Google Assistant), knowledge graphs can provide personalized search results.
Knowledge graphs support:
- Descriptions that contribute and build on one another, forming a network
- Diverse data is interconnected and described using semantic metadata
- Descriptions using formal semantics that allow both computers and people to process and understand them efficiently
Data Lakehouses
Data lakehouses are quite new and use semantic technology. In fact, they could not exist without it. The primary problem with data lakes is finding the desired data, and as a consequence, data lakes can quickly become data swamps. A data lakehouse typically comes with its own storage system but can also be used to work with additional data lakes and data warehouses.
The primary distinction between data lakehouses and data lakes is that lakehouses use semantic technology, while data lakes do not.
Data lakehouses are primarily used in cloud environments and can accept data from a variety of different sources. This includes unstructured, semi-structured, and structured data. The use of semantic technology in data lakehouses is why they have started gaining popularity.
Semantic Technology and AI
Often called “semantic AI,” semantic technology with machine learning is being used more and more. (For generalized purposes, such as article titles or advertising, the term “machine learning” is often replaced with “AI.”) Machine learning algorithms generally work well with structured data and text but have difficulties functioning with other data formats. Semantic technology helps to resolve this problem.
Semantic technology helps machine learning algorithms understand unstructured data.
Machine learning can help in expanding knowledge graphs, and knowledge graphs can help in developing ML algorithms. This self-expanding process ultimately leads to a self-optimizing system. It promotes the evolution of machine learning algorithms based on use, rather than preplanned expectations.
Additionally, semantic technology can be used to help chatbots and virtual assistants speak in a more human-like fashion.
Conversational Chatbots
Conversational chatbots are also called virtual or digital assistants and are much more sophisticated and interactive than task-oriented chatbots (single-purpose software performing one function). These chatbots are aware of context and use NLP, as well as ML, to learn and improve. They can use predictive intelligence and analytics to provide a personalized customer experience, based on their profile and past behavior.
Over time, virtual assistants can learn a customer’s preferences, providing recommendations and anticipating needs. In addition to monitoring data, they can also initiate conversations. Siri and Alexa are examples of predictive conversational chatbots.
Advanced virtual assistants can be used to connect several different single-purpose, task-oriented chatbots under one umbrella. This design typically allows data from different sources to be gathered. This gathered data is then combined to perform a task. This is done while still maintaining context, and prevents the chatbot from becoming “confused.”
Summary
Semantic technologies are focused on meaning and context. They can search directly for concepts, topics, and relationships spanning a wide variety of sources. Semantic technologies can be used in several different contexts, such as:
- Auto-recognition of themes and concepts
- Information and meaning-extraction
- Semantic data integration
- Taxonomies/classification
The use of semantic technology can be a powerful tool for research and processing data, especially when combined with machine learning and artificial intelligence. Semantic technologies offer a new data paradigm, which provides more intelligent and relevant responses than did previous intelligence technologies.
Image used under license from Shutterstock.com