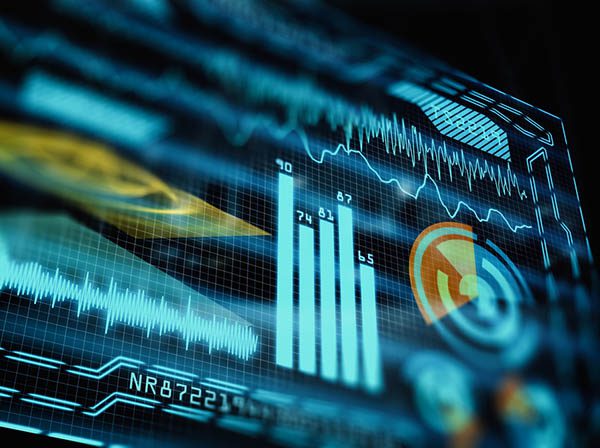
Self-service analytics offers dynamic reports to business users, who can analyze the data by sophisticated, in-built functions. This kind of user empowerment reduces reliance on IT staff. Moreover, self-service business intelligence (BI) capability may additionally equip users with external data access and built-in features to instantly generate finished reports. To use a complete range of analytics or BI functions, users do not need to understand anything of the underlying programming layer of the applications.
Advanced Analytics Use Cases makes a strong case for “self-service analytics platforms,” which, the author states, have the power of being advanced analytics tools to the ordinary user’s desktop.
The Self-Service Analytics Market
The North American self-service BI market is likely to dominate through 2022. The rapid adoption of big data and the increase of data-driven organizations have fueled this market, but some major drawbacks in the technology are security breaches, absence of clean data, and hefty investment costs.
While selecting appropriate solutions for their businesses, business operators are still faced with many challenges, like finding tools to monitor all aspects of business, locating the right functions to measure the KPIs, or understanding the right methods to analyze performance outcomes. Another significant challenge is understanding how to acquire data from different operational pipelines and manage them on a unified platform for further analysis.
Some of the common concerns of a business buyer are how much manual data entry or coding is allowed, what are the platform integration capabilities, are routine solutions available easily, and whether the software is scalable.
Building Trust between IT and Business Users
Although executives or business leaders may get fascinated by the razzle-dazzle of nifty tools, colorful dashboards, or multimedia reporting capabilities, it is prudent to involve seasoned IT staff in the buying decision. A good approach is to provide a “wish list” of technology requirements to the in-house technical experts, who can then survey the market to find solutions that adequately meet those requirements.
A Gartner press release announced that: “Self-service analytics and BI users will produce more analysis than Data Scientists will by 2019.”
As observed by Gartner’s senior staff:
- To be able to use the applications properly, self-service analytics users will have to be initially guided in using the solutions and tools by technical (Data Science) staff.
- The success of a self-service analytics platform will depend largely on the Data and Analytics Governance model, which must be capable of supporting “free-form” data discovery. More information is available in Governed Self-Service: Who Gets Data Access in Your Company?
- Rather than providing access to data and a handful of tools, the technical staff must invest time and effort in building trust between themselves and other users.
Self-Service Analytics Use Cases
Automated algorithms — with powerful capabilities to learn from historical data and apply the learning to pattern recognition for future predictions — were once the realm of “advanced analytics,” but have now moved into the territory of self-service analytics. Both the model-creation and model-deployment phases are critical to the success of a self-service analytics platform. In most cases, business users prefer a safe transition, which is a simple “predictive model,” or a handful of those to make decisions that are superior to their existing technological capabilities.
After the initial transition, business users will gradually want to do more than just sharing data or viewing automated dashboards. Users will want to learn how to analyze reports or results, and convert them into actionable decisions quickly, in the middle of work. Thus, some of the top requirements of popular self-service analytics platforms are: ease of use; ease of navigation; auto-update features; and easy integration with other applications.
Self-Service Analytics in Healthcare
Rado Kotorov, Chief Innovation Officer at Information Builders, believes:
“[O]rganizations have the opportunity to dig deeper to uncover significant indicators and metrics, explore information and discover its potential, interact with real-time data, automate the scheduling and delivery of vital information and use the resulting insights to drive better business outcomes. Analytical insights are no longer reserved for data scientists and other elite groups.”
The United States is the 50th out of 55 developed countries in the “effectiveness of healthcare delivery” in a study that measured quality patient care at affordable cost. While dealing with healthcare data troves, the analysts realized that barriers like rigid data frameworks, latency, system complexity, and understaffing can cause serious impediments to quality healthcare delivery. The most serious roadblock is often found in electronic health records (EHR), which can lead to decision bottlenecks and reduced outcomes.
In self-service healthcare analytics, all these challenges are considerably reduced, enabling healthcare professionals to directly access, analyze, and process patient data in relatively short time without having any Data Science knowledge. In most self-service analytics solutions designed for the healthcare industry, “data access, preparation, analysis, and reporting” are automated through advanced technology. The flexibility of self-propelled, insight-driven healthcare solutions can facilitate accurate diagnosis, timely treatment decisions, better treatment outcomes, and value-added healthcare delivery.
Data Governance is another area that still makes industry insiders question the reliability of stored data in the healthcare industry. The Impact of Data Governance on Self-Service Analytics states that in self-service, the phase of “data preparation” is partially or wholly automated, which indicates “that this technology may actually serve as a driver for greater Data Quality and Data Governance, not an inhibitor.” The most visible gains of a self-service healthcare analytics platform are freedom from reliance on IT staff, user empowerment, and accurate decision-making.
Self-Service Analytics in Marketing
With Looker Sales Analytics solution, any sales team is equipped with “actionable data” to filter “leads or deals.” Through Looker’s Sales Analytics platform, enterprise customers can collect and view all data through a unified Data Management platform. The article Building on the Platform: Custom Applications for Sales & Marketing explains how this unique application is delivering “custom data experiences.”
Self-Service Analytics in Manufacturing
In manufacturing business, business operators survive on “Six Sigma,” (alternatively, “Define Measure Analyze Improve Control Cycle [DMAIC]”), which serves as the primary quality benchmark. This quality tool can now be used in tandem with an enterprise-grade industrial analytics system to transfer results achieved in one unit or plant to other units across the enterprise, thus formalizing and replicating operational excellence throughout the business.
Six Sigma is aligned with data-driven applications to drive continuous quality improvements. If properly implemented and executed, the industrial analytics system can help any manufacturing plant achieve cost efficiency, a low carbon footprint, and greater safety.
In a lean manufacturing environment, a Six Sigma project may run from three to six months, within which time managers have to test and design performance metrics in an iterative manner. This Science Direct article describes implementing a self-service business analytics system that supports lean manufacturing.
Self-Service Analytics in Banking and Finance
In the financial services industry, accurate and timely financial decisions must reach the customer. The current challenges are disconnected data and lack of Data Science talent.
With the help of a self-service analytics platform, finance professionals can remove the problem of “data silos” through data integration tools. Moreover, a self-service BI platform will empower the financial services staff with auto-prepared data — all ready for analysis. This way, the financial services staff does not have to depend on technical IT manpower to get their jobs done.
End result? The customers get timely and accurate financial guidance. A Digitalistmag article demonstrates how self-service analytics for the financial services has empowered the staff employees. Not only are they making fast decisions, but the decisions are backed by sound data analysis.
Of course, the data security concerns are much higher in the financial service industry, but the newer, governed self-service analytics solutions can address those issues. Self-Service Data Analytics in Financial Services describes one such self-service analytic solution for the financial services business.
The article Top Five Banking Activities Best Handled by Digital Self-Service offers a specific list described by its title. Today, net-banking, with its wide array of digital-banking facilities, is one of the most widely used self-service banking applications across the globe. Banking customers love the power and flexibility of net-banking, and increasingly, banking customers of all ages are opting for digital banking.
Trust is one area where banking technology faces serious challenges; also, the frequency of online banking fraud is on the rise. In spite of advanced digital platforms available for use, banking customers still prefer to visit the branch or speak to a customer representative through phone banking. As digital banking technology gains more “trust” among worldwide customers, over time the bank visits will come down.
Retaining customers is another serious challenge in the digital banking age, where a competitor can whisk away a rival’s old customers through an online chat or an SMS. Thus, many banks now are refocusing on physical branch services for high-value customer retention.
Banks have learned to use Big Data analytics to identify and locate their high-net customers for branch banking. The same analytics platform may be used to identify low-value customers for self-service net-banking or kiosk banking.
The Last Word: Augmented Analytics
A Gartner post defines augmented analytics as the “automation” of competitive intelligence, signaling the dawn of AI-enabled business analytics. The greatest value of augmented analytics is that business intelligence may be conducted on-demand, because the system is automated. What is Augmented Analytics and Why Does it Matter? discusses the benefits of augmented analytics. Businesses that conduct a lot of predictive analytics have a lot to gain from this machine-learning-powered analytics technology.
According to Gartner:
“By 2021, the number of users of modern BI and analytics platforms that are differentiated by smart data discovery capabilities will grow at twice the rate of those that are not, and will deliver twice the business value.”
Image used under license from Shutterstock.com