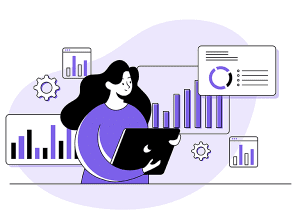
Self-service analytics empowers the non-technical users in an organization. Traditionally, data analysis was the domain of specialized data scientists or IT professionals with skills to manipulate and interpret complex datasets. In self-service analytics, user-friendly tools enable ordinary business users to conduct data analyses without expert knowledge or support.
These tools typically feature user-friendly interfaces, drag-and-drop functionalities, and pre-built templates that make it easier for users to generate insights from raw data. The goal is to expedite decision-making processes by reducing dependency on specialized personnel for generating reports and interpreting data trends. By enabling real-time access to actionable insights, self-service analytics promotes efficiency and innovation.
Key Features of Self-Service Analytics
Key features of self-service analytics include intuitive interfaces – often with drag-and-drop capabilities – for creating visualizations and reports. These platforms often integrate with various data sources, providing a seamless experience for users to pull in the data they need. Advanced features like natural language processing (NLP) allow users to query data using everyday language, making analytics more accessible.
The benefits are multifaceted: organizations can foster a culture of data-driven decision-making across all levels, leading to faster insights and improved agility. By democratizing data access, self-service analytics reduce bottlenecks typically associated with traditional BI processes, where business users rely heavily on IT teams.
This autonomy not only accelerates decision-making but also frees up IT resources for more strategic tasks. Ultimately, self-service analytics enhance productivity and drive innovation by empowering employees with the tools they need to analyze and interpret data independently.
Robust Data Governance Networks
Robust data governance frameworks ensure data quality, consistency, and security while providing users with access to relevant datasets. Techniques such as metadata management help in cataloging and indexing data sources, making it easier for users to find and utilize the information they need.
Integration of Self-Service Analytics with Real-Time Data
Another significant trend is the integration of self-service analytics with real-time data streams. This will empower organizations to make on-the-fly decisions based on the most current information available, enhancing agility and responsiveness. Additionally, enhanced data visualization tools will offer more dynamic and interactive ways to explore data trends and patterns.
The Advantages of Self-Service Analytics
Enhanced productivity and business efficiency: Enhanced productivity and business efficiency are among the foremost advantages of self-service analytics. By empowering employees across various departments to access, analyze, and visualize data independently, organizations can significantly reduce the bottlenecks associated with traditional data analysis processes.
In conventional settings, data queries often need to go through specialized IT or data science teams, creating delays and limiting agility. Self-service analytics tools democratize data access by providing user-friendly interfaces that require minimal technical expertise. This shift not only speeds up decision-making but also fosters a culture of data-driven thinking at all levels of the organization. Employees can rapidly generate insights relevant to their specific roles without waiting for centralized approval or support.
End-users have instant access to real-time insights: Self-service analytics empowers end-users with real-time insights and quick decision-making. In business intelligence (BI) models, data analysis often requires the mediation of specialized IT personnel or data scientists, creating bottlenecks that delay crucial decision-making processes. Self-service analytics democratizes data access, allowing employees at all levels to harness the power of real-time insights without needing extensive technical know-how.
This immediate access to data fosters a more agile and responsive organizational culture. When end-users can explore datasets, generate reports, and visualize trends on their own, they are better equipped to identify opportunities and mitigate risks swiftly.
Moreover, real-time analytics provide a dynamic view of operations as they unfold, enabling proactive rather than reactive management. By bridging the gap between raw data and actionable intelligence, self-service analytics empowers individuals to contribute more effectively toward achieving organizational goals, fostering a culture of continuous improvement and innovation.
Cost-effective and time-saving benefits: Self-service analytics offers significant cost-effective and time-saving benefits that can transform how organizations handle their data. By empowering employees across various departments to access and analyze data, companies can reduce the need for extensive, ongoing training sessions and costly external consultations.
The simplicity of drag-and drop tools reduces the learning curve and allows users to swiftly generate reports and visualizations. Consequently, organizations save significant amounts of time that would otherwise be spent waiting for IT departments to process requests.
The reduction in dependency on specialized personnel not only cuts down on labor costs but also allows these skilled professionals to focus on more complex tasks that require their expertise. As a result, businesses can allocate resources more efficiently while maintaining high levels of productivity. In essence, self-service analytics streamlines operations by making data analysis both accessible and economical, driving more timely and informed business decisions.
Improved data governance and security: Improved data governance and security are critical benefits of self-service analytics, as these systems are designed to empower users while maintaining stringent control over data access and integrity. This shift necessitates robust governance frameworks to ensure that only authorized individuals can access sensitive information.
Improved governance mechanisms help maintain data quality by enforcing standardized practices across various departments. This ensures that all analytical outputs are consistent and reliable, fostering a culture of accountability and accuracy in decision-making processes throughout the organization.
Modern self-service analytics platforms come equipped with sophisticated security features such as role-based access controls (RBAC), encryption protocols, and audit trails. These tools allow organizations to define who can view, modify, or share specific data sets, thereby minimizing the risk of unauthorized access or data breaches. Additionally, audit trails provide a transparent log of all user activities within the system, facilitating easier compliance with regulatory standards like GDPR or HIPAA.
The Disadvantages of Self-Service Analytics
On the flip side, here are some disadvantages of using self-service analytics:
Lack of expertise and inadequate training: Self-service analytics promises democratized data access, empowering employees across an organization to make data-driven decisions. However, one significant drawback is the lack of expertise and inadequate training among users. Not everyone has the necessary background in data science or statistical analysis to accurately interpret complex datasets. This knowledge gap can lead to misinterpretations and misguided decisions, potentially causing more harm than good.
Furthermore, while self-service tools are designed to be user-friendly, they often require a foundational understanding of analytical concepts to be used effectively. Without adequate training, users may struggle with the nuances of these tools, leading to inefficiencies and errors in their analyses. Training programs can mitigate this issue but are often expensive and time-consuming, representing a substantial investment for organizations.
Finally, over-reliance on self-service analytics could marginalize the role of specialized data professionals whose expertise is crucial for nuanced interpretation and validation of insights. In essence, without proper expertise and training, self-service analytics can transform from a powerful enabler into a source of misinformation and poor decision-making within an organization.
Misinterpretation and bias in analysis: Misinterpretation and bias in analysis can pose significant challenges in the context of self-service analytics. When users who are not trained in data science or statistics engage with complex datasets, there’s a heightened risk of drawing incorrect conclusions. This can stem from a lack of understanding about how to properly clean, filter, and interpret data. For instance, users might overlook confounding variables or misapply statistical tests, leading to misleading results.
Moreover, cognitive biases can further distort analysis. Confirmation bias may drive individuals to seek out data that supports their preconceived notions while ignoring information that contradicts them. Availability bias might cause users to give undue weight to recent or easily recalled events rather than a comprehensive view of the dataset.
The absence of a rigorous peer review process increases the risks of flawed data analysis. In traditional analytics workflows, multiple layers of oversight helped ensure accuracy and objectivity. Self-service models often lack this safety net, making it easier for flawed analyses to go unchecked.
Compliance issues and inconsistent reporting: Self-service analytics, while empowering users to generate insights independently, presents significant challenges in terms of compliance and reporting consistency.
One major issue is the potential for non-compliance with regulatory standards. When data governance is decentralized, ensuring that all analyses adhere to legal and industry-specific regulations becomes increasingly difficult. Users may inadvertently access or share sensitive information without proper authorization or fail to apply necessary data-masking techniques, leading to breaches of confidentiality and hefty penalties.
Inconsistent reporting is another critical disadvantage. With multiple users creating their own reports and dashboards based on varying interpretations of the data, discrepancies can arise.
This lack of uniformity can lead to confusion and misinformed decision-making at higher organizational levels. Different teams might rely on divergent metrics or definitions, causing a fragmented understanding of business performance.
Scalability challenges and inefficient decision-making: Scalability challenges and inefficient decision-making are significant disadvantages of self-service analytics. As organizations grow, the volume and complexity of data increase exponentially. Self-service analytics tools, while initially empowering for individual users, often struggle to scale effectively within large enterprises. The infrastructure required to handle massive datasets may not be robust enough in these tools, leading to performance bottlenecks and slower processing times. This can frustrate users who rely on quick insights to make timely decisions.