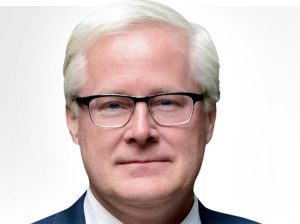
Artificial intelligence has become a cornerstone of public sector modernization, putting a higher importance on data centers, the energy they consume, and how to make them operate more efficiently.
Generative AI models require immense computational power to operate. For example, an AI workload like a ChatGPT query can take more than 10 times the energy of a Google search. In response, the biggest tech companies invest in nuclear power to meet skyrocketing electricity demands. Amazon and Google are opting for a new generation of small modular reactors to meet pledges for emission-free operations by 2030.
However, these environmental pledges were made before AI’s ascendency, as were most public sector agencies’ sustainability goals. Many CIOs now feel stuck between the need to increase capabilities for AI and automation projects while also reducing energy consumption at the same time.
But this balance isn’t impossible, and the solutions go beyond graphics processing units (GPUs). Advances in server technology and AI accelerators can equip data centers to integrate AI securely while also ensuring long-term scalability and sustainability.
Bridging the Gap Between AI Ambition and Sustainable IT
For over a decade, public sector agencies have focused their modernization efforts on migrating appropriate workloads to cloud environments. Updating data center hardware wasn’t a priority until agencies started questioning the security of putting sensitive data into public genAI tools.
Many agencies can use their existing central processing unit (CPU)-based platforms for AI workloads without purchasing specialized GPUs. Replacing aging data center components with the latest generation servers and processors offers agencies a significant boost in computing power and energy efficiency. Refreshing a five-year-old server with the latest processors can lower total ownership cost by 77% by reducing the number of servers needed for the same performance, according to IDC research. Consolidating workloads on fewer servers and cores can also lower software licensing costs and shrink the data center’s overall footprint and operating expenses.
AI accelerators are part of what makes this possible, as it is essentially like boosting a car’s performance with nitrous oxide. These CPUs are architected for advanced computing with higher memory and data throughput capabilities, making them especially well-suited for tasks like AI inference. GPUs, on the other hand, fall short in the memory specs. Their strength is processing tasks in parallel, such as training AI models. However, most AI workloads – roughly 80% – center on inference.
Quick Wins for Public Sector AI
Developments in AI are always rapidly moving so it can be challenging to know where to start with AI adoption. There’s no well-trodden playbook for the public sector to follow. Agencies’ first impulse is often to build their version to maintain control of sensitive data and enforce security requirements. However, this approach is often incredibly time-consuming and expensive while also lagging behind the capabilities developed in the private sector.
Rather than investing in expensive GPU clusters or building large language models (LLMs) from the start, agencies can take advantage of pre-trained small language models (SLMs) and data processing techniques that take advantage of existing data centers. In less than a week, agencies could launch a simple chatbot that answers citizens’ questions, significantly improving citizen services without spending time building everything from scratch.
Leaders should also prioritize experimentation. While public genAI tools like ChatGPT and Anthropic offer a quick start, data privacy is a legitimate concern. Private genAI uses open-source LLMs or SLMs that provide agencies with a safer space to try new approaches without leaking data into the public sphere. Depending on what architectures they use, agencies can skip training models but still foster an understanding of potential use cases and limitations. Every day, developers create new techniques to extend private genAI use cases to include things like summarizing documents, translation services, and even augment decision-making. The time for agency leaders to get their hands dirty planting seeds for a future harvest is now.
The path to AI adoption in the public sector isn’t a choice between innovation and sustainability. Thoughtful data center refreshes can deliver the performance and capacity for AI projects while keeping energy costs down. As the landscape of AI continues to evolve, public sector CIOs have the opportunity to lead the charge in responsible AI adoption, demonstrating that technological advancement and environmental stewardship can go hand in hand.