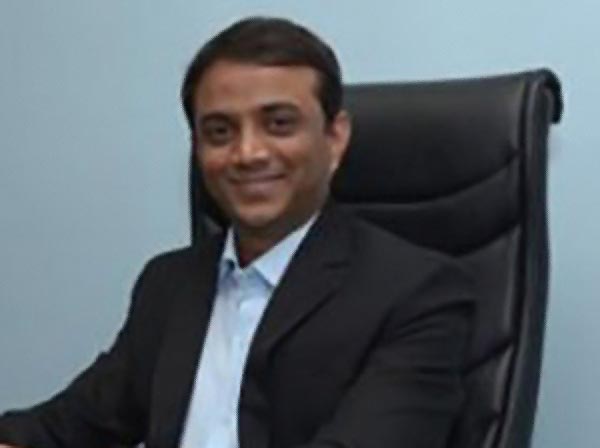
Click to learn more about author Ashish Parmar.
Healthcare dynamics are changing. The industry has moved from volume-based healthcare to value-based healthcare due to growing demands from customers for modernized, quality, and regulated healthcare services. Healthcare providers are under pressure to stay productive and efficient, in addition to addressing the problem of a constantly growing torrent of data and complexity.
The taxing situation creates a need for technology that enables healthcare providers and payers to make informed decision at an accelerated pace for delivering better outcomes. Predictive analytics – a branch of advanced data analytics – can help by predicting future events or activities utilizing data mining, modelling, statistics, and artificial intelligence technology.
Healthcare providers can look into the future and identify the trends that can positively or negatively impact patient care, cost savings, staff management, and others. Savvy leaders have embraced predictive analytics to influence healthcare outcomes, drive revenue growth, and build competency in the market.
A few of statistics for predictive analytics in healthcare:
- 60 percent of healthcare executive consider predictive analytics to be a budget saviour.
- 87 percent of executives say predictive analytics is the future of the healthcare business.
- Over the next five years, spending on predictive analytics is anticipated to increase by 58 percent.
It seems that a sizeable chunk of healthcare providers are taking the technology seriously to remove inefficiencies, meet the increasing demand for information access, and unlock insights from information to drive clinical and operational improvement. Let’s discuss in detail how technology is working like a pro and also bringing risk.
Why Healthcare Executives are Looking at Predictive Analytics as a Priority
- It promises healthy budget savings
Healthcare providers are ramping up their analytics capabilities to reduce healthcare costs by a great margin.
The supply chain is another place where predictive analytics holds great potential in understanding the ordering patterns and supply utilization and then optimizing the variation in supplies to diminish unnecessary spending and improve supply chain efficiency. Proactive, data-driven decisions in the supply chain could save millions of dollars for hospitals. It improves operational management efficiency
- It improves operational management efficiency
Conventionally, due to some seasonal occurrences, the shortage of beds or changes in staff levels arise that make providing effective treatment difficult.
Analyzing the historical data and real-time patient admittance rate, a hospital gets alerted about a bed shortage ahead of time and employs extra staff to deal with the issue before it arises. The insights help in managing optimal patient-to-staff ratio and improve the accuracy of diagnosis, which in turn results in quality care.
The predictive models can also help in the recruitment of extra staff to optimize the operations by assessing the competencies of new staff. For instance, there is an increasing demand in aged-care services for fully trained staff who can best handle the aging population. The use of the competency model and logistic regression model during the interview help recruiters to shortlist the best candidates.
- It enables diagnosis and treatment to be more precise
Every individual is unique so every medicine doesn’t work on every patient. For the health practitioner, it’s not feasible to analyze the lifelong health-related history of the patient before prescribing accurate treatment. Predictive analytics work at an individual level by connecting the dots (personal health history, current medication, family health history, and previous illness) and uncovering hidden insights (analyzation of big data) in a couple of seconds. When the technology is applied, the practitioners can effectively come up with the medication for a particular illness.
Before the patient undergoes any surgery, predictive analytics is leveraged to reveal the death risks based on the patient’s current health status, previous medical history, and current medication. The statistical data also help in taking the right medical decision and mitigate the mortality rates.
- It prevents the worst consequences by using risk scoring
Prediction and prevention are closely connected and can’t be separated. When the caregiver identifies the patient with a high risk of developing chronic conditions in the initial stage, the patients can be helped to avoid long-term health problems that would be expensive and difficult to heal in the future.
Predictive modelling helps in creating a risk score based on a patient’s diagnosis data, biometric data, health-related data, and other determinants that further aids in classifying the patients at high risk of poor health outcomes. The insights into every individual patients’ health enable the caregiver to provide the enhanced services that ultimately improve risk management and make the transition to value-based services seamless.
- It supports responding proactively to patient deterioration
Often, after being admitted into the hospital, a patient’s health is negatively impacted due to hard-to-treat infections or unexpected changes in the clinical conditions. The caregivers have to make decisions faster and precisely, else it poses a potential threat to the patient.
When the clinical decisions are backed by predictive analytics tools, the accuracy of the decisions surpasses the accuracy of gold-standard tools because the analytics tools are damn good at collecting and analyzing every piece of data that human practitioners can overlook.
When health practitioners are powered with predictive models, they can identify the deterioration in a patient’s health before the symptoms can be seen with naked eyes and make changes in the patient’s medication accordingly.
- It can help a patient avoid unnecessary trips to the hospital and handle unexpected gaps in patient follow-ups
Repetitive hospital visits or readmission for a simple health problem interferes with the hospital’s daily operations and puts a hole in the patient’s pocket as well. A predictive tool such as remote health monitoring allows the patient to provide health-related data to the caregiver and intelligent machine learning technology calculates the risk score; when a measurable variation is found, the practitioner will get an alert. Thereafter, the changes can be made in the treatment or the patients can be asked to visit or get admitted to the hospital.
On the flip side, predictive analytics can find out when the patient is likely to skip an appointment without any prior notice. The information helps in keeping the slot open for another patient, trim revenue losses, and enable faster access to care. Also, the data can be used to send reminders to high-risk patients who fail to show-up or provide alternative schedules that best suit the patient’s schedule.
- It ensures data security
It’s not just financial institutions that are prone to cyberattacks. Healthcare organizations are too. Here, predictive tools can analyze the patterns in data access, sharing, and utilization and warn the concerned person about the changes made and signal that ransomware has penetrated the network.
The calculation of the risk score in real-time for the specific request or transaction by the analytics tools enables the system to either block or grant access or apply multi-factor authentication. The adoption of intelligent technology solutions across the infrastructure allows the healthcare organizations to defend against the attackers, no matter how complex the attack is.
- It strengthens patient engagement and satisfaction
Just like the ecommerce industry, the healthcare industry has turned from a service-oriented to a customer-oriented industry where engaging the patients with clinical and financial responsibilities is all-important. Customer relationship management is vital to promoting wellness, reducing clinical spending, and ensuring smooth communication.
Healthcare organizations are using predictive analytics to create individual patient profiles to create meaningful plans, send customized messages, engage patients, and identify the strategies that are positively impacting every individual. With patient’s behavioral patterns, the list of patients can be created that are willing to change and later, the patient can be approached accordingly, which further helps in bolstering the long-term engagement and diminish the risk associated with diseases.
The Barriers to Adoption
The inability to get relevant data from multiple disparate sources inhibits the delivery of meaningful insights at the right time and stymies predictive modeling technology adoption. The inability to capture information in real time, the lack of staff skilled enough to use analytics in business processes, the absence of sponsorship executives, ineffective data governance, and regulatory issues such as HIPAA compliance are the primary obstacles to analytics adoption. Moreover, some organizations find that the perceived costs of analytics adoption outweighs its benefits.
Conclusion
On the predictive analytics spectrum, the performance gains, financial gains, and quality gains of predictive analytics upon complementing the healthcare services give no reasons to the healthcare organizations and practitioners to ignore the technology. When the patients are already preferring modernized services, then how the healthcare services can stay behind and doesn’t make the most out of the lucrative opportunities. Don’t wait for the competition to embrace the technology and innovate, else your patients won’t wait for you anymore.