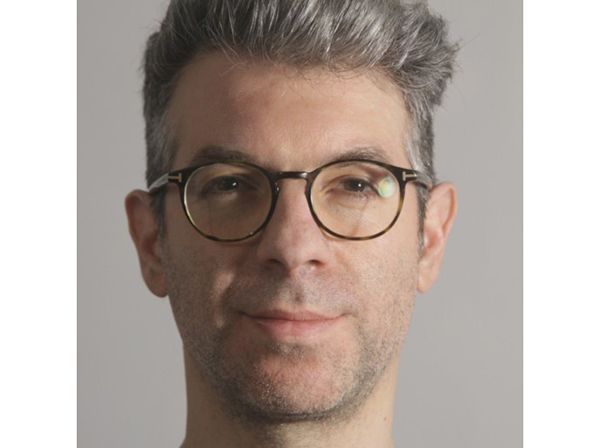
As we head into 2023, machine learning (ML) professionals are taking stock of the past year and identifying potential key opportunities moving forward. To that end, my company recently polled 200 U.S.-based ML decision-makers to better understand what those opportunities might be. One area we focused on was the challenge behind operationalizing machine learning, which respondents flagged as a key issue.
While machine learning can bring a great deal of value to organizations in every industry, it’s important to recognize that businesses can only actualize that value when they can operationalize an ML model. With that in mind, here are some of the most interesting findings from our research, plus thoughts on how the MLOps category can rise to the occasion and improve to make ML more useful and accessible across industries.
Inability to Operationalize ML Models Hurts Revenue
When we asked machine learning experts if their organizations were challenged to create business and commercial value from ML investments – by deploying or productizing machine learning pipelines and projects at scale – virtually everyone (86%) agreed, with almost one-third (29%) saying they were “very challenged.” Similarly, almost three-quarters said their company was missing out on revenue or value creation due to challenges in operationalizing ML at scale, with roughly half describing these challenges as either “severe” or “very severe.”
Obviously, these numbers speak to fundamental issues that need to be solved for in 2023 and beyond. For example, the need for more investment in tools to support basic machine learning processes to improve the development, deployment, and maintenance of models. As well as a focus on automating the process of building, testing, deploying, and managing machine learning models in a production environment, enhancing collaboration, project management, and operationalization.
Investments in ML Process Automation Will Be a Priority
Some in the industry believe a recession will undercut AI and machine learning investments. In reality, spending is likely to continue. However, what will change are the types of AI and ML that companies will want to invest in.
I anticipate companies will invest in technologies that can improve efficiency and productivity in the immediate term. As companies look to optimize costs and streamline their operations in 2023, they will likely turn to AI and ML platforms to help them automate processes and tasks on a large scale. By automating these routine activities, functions, and systems, companies can free up capital, talent, and other valuable resources to focus on more high-level, value-added projects. This will allow them to free up resources and save costs quickly, ultimately improving their profitability and time to market.
We also see this trend toward automated optimization play out in the survey, as leaders expressed interest in continued investment in resources to maximize ML processes, especially automation and orchestration. By automating their ML operations, organizations can do more with less, and this focus on efficiency and productivity is particularly valuable in times of economic downturn.
Unclear Goals Hurting Operationalization
Not surprisingly, there is a disconnect between organizations and their machine learning projects, which is impacting the operationalization of models. Our study found that nearly 20% of respondents claim “unclear organizational strategy and goals” are challenging operationalizing ML at scale within their company.
To solve this, organizations must take more of a holistic approach to their ML workflow, ensuring that there’s more clarity of ML’s purpose and impact to the organization across the board. This means that ML teams and C-suite leaders should work together to identify the specific business goals and objectives that the organization hopes to achieve through its machine learning initiatives. This should include defining metrics for success, such as increased revenue or improved customer satisfaction. It also means that both teams should regularly review and assess progress on ML initiatives to ensure that they are meeting their goals and delivering the expected value. In closing this gap between ML teams, DevOps, and the C-suite and creating more transparency and collaboration, the industry can better address this obstacle of unclear strategy and goals.
To summarize, our research shows that ML operationalization is a key challenge as well as an opportunity for investment and growth in 2023. As organizations look to optimize investments in a challenging economic environment next year, I believe achieving excellence in ML operationalization will be a top priority.