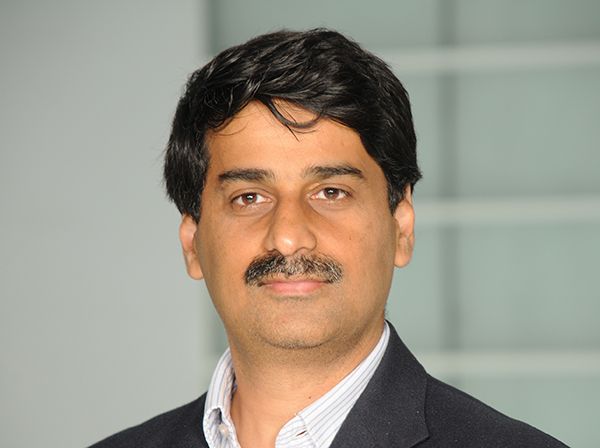
AI is everywhere, and it is growing. In a 2022 edition of an annual global AI survey, a leading consulting firm found that adoption among enterprises had more than doubled in five years, with about 50% of respondents using it in at least one business unit or function. Thirty-two percent of enterprises reported cost savings from AI, while 63% had seen their revenues increase. However, the survey also returned one finding of concern – that despite ramping up the use of AI, enterprises had not increased their efforts to mitigate its risks by a significant degree. A dialogue was started on the growing need to self-regulate AI when an open letter from many respected tech leaders called for a six-month pause on developing systems more powerful than GPT-4, citing several concerns. Sam Altman, OpenAI’s co-founder, also urged U.S. lawmakers in a Senate hearing to expedite the development of regulations. This is probably the first time in history that private institutions are asking government agencies to impose regulations on them.
Meanwhile, AI is rapidly escalating in day-to-day life. When the Pew Research Center surveyed about 11,000 U.S. adults in December 2022, 55% were aware that they interacted with AI at least several times a week. The remainder of respondents believed they did not use AI regularly. However, the reality is that a very significant number of people engage with AI without being aware of it. This means that they could be unwittingly exposing themselves to its risks, such as privacy violation, misinformation, cyberattack, and even physical harm. Now, with generative AI bursting onto the scene, the risks are multiplying to include copyright infringement, misinformation, and rampant spread of toxic content.
A strategy to mitigate potential risks of generative AI should ideally follow a three-pronged approach consisting of the following:
1. Technical guardrails
When it comes to generative AI, the risk of inherent bias, toxicity, hallucinations, etc. becomes very real. Enterprises need to invest in a fortification layer to monitor and mitigate the risks. This layer will ensure large language models are not using sensitive or confidential information while training or in the prompt. Further screening can be undertaken for detecting toxic or biased content and restricting certain content to select individuals in the enterprise, as noted in company policy. Any prompts or outputs that are not in line may be blocked or marked for review by regulatory/compliance teams in the enterprise.
These systems need to be explainable and transparent so that users understand the reasoning for the decision. These functions are served by various tools that are emerging and need to be adopted or built in-house by the organization. For example, Google’s Perspective API and Open AI’s moderation APIs are used to detect toxicity, abuse, and bias in generated language. There are a lot of examples of open-source frameworks that provide personal identifiable information (PII) detection and redaction in text, images need to be used as guard rails in machine learning operations (MLOps) workflows. For preventing hallucinations, there are open-source tools like Microsoft’s LLM Augmenter, which has plug-and-play modules that are placed upstream from LLM-based applications, and can fact-check LLM responses by cross-referencing it against knowledge databases. Recently, NVIDIA also has developed the open-source NeMo Guardrails, which can enforce topical security and safety guardrails on generative AI assistants so that responses are in-line with organizational policies.
We are currently working with a global healthcare company to build a control and monitoring framework for adopting OpenAI APIs. In this effort, various aspects of privacy, safety, and filtering specific querying intents like passing restricted information, auditing end user’s actions, history, and an incident auditing dashboard will monitor and mitigate issues that arise while adopting ChatGPT in their organization.
Apart from tools, platforms, and accelerators, enterprises need to look at building a responsible AI reference architecture, which can be used as a guideline for all AI pursuits. This reference architecture will map all the accelerators and tools along with a catalog of APIs that need to be factored in different use cases and lifecycle stages. This also will act as a baseline for building a comprehensive and integrated responsible AI platform that will implement common patterns and expedite AI adoption across the organization.
2. Policy- and governance-based interventions
Enterprises need a comprehensive policy covering people, processes, and technology to enforce the responsible use of AI systems. Without specific government or industry regulation, AI companies need to rely on self-regulation to stay on the right path. Several frameworks can be used as guidance, including the recent AI Risk Management Framework (AI RMF) from the National Institute of Standards (NIST), which provides an understanding of AI and its potential risks. Apart from a robust governance framework spanning the AI lifecycle, there should be a structured approach that allows the principles to be put into practice, without stifling innovation and experimentation. Some of these are:
- Laying a strong foundation by defining the principles, values, frameworks, guidelines, and operational plans to ensure responsible AI development across the AI lifecycle including development/fine-tuning, testing, and deployment.
- Develop risk assessment methodologies, performance metrics, conduct periodic risk assessments, and evaluate mitigation options.
- Create systems for maintaining and upgrading documentation on best practices, guidelines, tracking, and traceability for compliance monitoring.
- Build a responsible AI roadmap to scale existing best practices and technical guardrails across use cases and implementations.
- Set up a supervisory/model risk management (MRM) committee for examining each use case for possible risks and suggesting ways to mitigate them. A review board should also be established for conducting regular audits and compliance inspections.
- Assemble an internal team with representation from legal, risk, technical, and domain areas to define and evaluate the appropriate AI solution to represent diverse groups.
- Establish clear accountability for policy enforcement and mechanisms to detect oversight.
- Conduct periodic training for employees to sensitize them to best practices of responsible AI, tailored to their specific roles.
- For a multinational organization, a robust research team to keep an eye on various draft and proposed regulations across the organization would be a prudent investment to ensure a future-proof policy framework.
3. Collaboration
All organizations leveraging generative AI to build new innovations should foster an atmosphere of collaboration and share their best practices on how they are building guardrails. Enterprises need to collaborate with each other, system integrators, academic institutions, industry associations, think tanks, policymakers, and government agencies. These collaborations should focus both on policy and technical aspects of developing guardrails by sharing code repositories, knowledge artifacts, and guidelines.
There should be concerted efforts across the AI community, not just enterprises and institutions, to fast-track these efforts by knowledge sharing and providing feedback. This will ensure that the engines of innovation can move forward with improved focus on AI safety and governance.