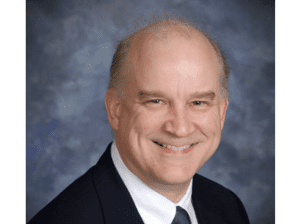
Welcome to the latest edition of Mind the Gap, a monthly column exploring practical approaches for improving data understanding and data utilization (and whatever else seems interesting enough to share). Last month, we explored the data chasm. This month, we’ll look at analytics architecture.
From day one, data warehouses and their offspring – data marts, operational data stores, data lakes, data lakehouses, and the like – have been technological workarounds. In “Building the Data Warehouse,” the 1992 book that launched modern decision support, Bill Inmon recognized the need for a decision support architecture that was different from the existing operational systems architecture, largely due to technological limitations at the time:
- Operational systems didn’t have the processing power to accommodate both analytical and transactional workloads simultaneously.
- Operational systems didn’t have the disk space to store historical data or multiple versions of an individual record.
- Networks didn’t have the throughput to merge data between operational systems.
But what if we didn’t have these CPU, storage, and network limitations?
Consider the evolution of how we watch movies. For decades the only options were going to the theater or tuning into whatever happened to be on TV. In the 1980s, VCRs became affordable for most families. Blockbuster Video flourished, and we could watch whatever we wanted whenever we wanted – as long as the store was open, the tape was in stock, and we went and got it.
I remember getting my first DVD player in the early 1990s and watching my first DVD. The picture was so clear. No static or fuzz. Suddenly videotapes seemed so primitive. Even though Blockbuster added DVDs to its in-store inventory, Netflix and its original by-mail DVD subscription service drove it out of business. You could still watch whatever you wanted to watch (mostly), but you had to wait.
By the next decade, improvements in internet infrastructure and advancements in video compression technology enabled streaming. Cable companies and streaming services, including Hulu, Amazon Prime Video, and a reoriented Netflix, could provide video on demand. Subscribers could watch what they wanted (mostly) when they wanted.
But that’s still not the end of the story. High-resolution, big-screen televisions exposed DVD quality limitations, so Blu-ray disks were introduced. Then came 4K and later 8K. Delivery methods evolved and accelerated as well, from over-the-air broadcasts to physical videotapes and discs to wired networks to cellular. We can now stream high-definition video on our cell phones. On demand.
What is the point of all this? Compare the evolution of on-demand movie watching with the evolution of on-demand data access. There is no comparison.
Technology improvements enabled data warehouses to evolve into bigger data warehouses that use files instead of an RDBMS – then into even bigger data warehouses that use both files and an RDBMS. Technology improvements enabled greater streaming velocity, query complexity, analytics sophistication, and data volume. Then we put it all into the cloud and called it a “modern architecture.” It’s not.
We are still implementing basic variations of 1990s architectures.
If we didn’t have CPU, storage, and network limitations, would we still move all of the data into huge, centralized data repositories?
The cloud provides the perfect opportunity to explore new, truly modern analytics architectures.
The separation of compute and storage means that operational systems are no longer bound by disk space. It’s sort of like water skiing. It doesn’t matter if the lake is 60 or 600 feet deep, you only need the six at the surface. Repository size can increase arbitrarily and data storage cost can be optimized according to access frequency.
Similarly, operational systems are no longer bound by the CPUs in the physical hardware installed in the data center when the application was deployed. Processing power is available on demand as needed. And the operational and analytical consumers can provision their own processing to access shared data.
That’s all great, but it’s axiomatic that data must be collocated to be joined. It’s the same with streaming data. Any lookup or correlated data must be available where the stream is processed. And what about queries that are run repeatedly or that use data frequently associated between disparate systems?
The key is to build intelligence into the analytics architecture and infrastructure, optimizing data placement and data access based on content and utilization characteristics. Data that’s used together frequently will migrate to become proximate automatically, organized by an overall data topology or ontology – one that is either defined a priori or discovered a posteriori.
Eventually, the data warehouse will evolve into a cache.
We’re moving in the right direction with the data mesh and data fabric concepts. I don’t want to wade into the present debate between the two, at least not now, which is oftentimes rooted in each advocate’s company’s product offerings.
The point is to facilitate access to enterprise data, to make it available faster and easier to consume. It’s about elevating the purpose of the enterprise analytics team from engine room data shovelers to producer/consumer relationship facilitators. And what does all of this depend upon?
Data understanding is the prerequisite for any modern analytics architecture.
I guess it’s not a mystery why progress has been so glacially slow. But it’s actually worse than that.
The data warehouse and its progeny have collectively devolved into an excuse not to understand the data.
How many times have you heard, “Just put it into the data lake and my analysts and I will figure it out”? Is your data lake derisively referred to as a “data swamp”?
New, modern analytics architectures will be free of the limitations we have worked around for decades. They will decentralize accountability, and, interestingly, simplify information management. The ownership and stewardship responsibilities of the operational system team (and its business partners) are clear. It’s no longer the analytics team coming in and getting the data, nor is it the operational system team throwing the data over the fence into the data lake regardless of content or quality.
Of course, this will require reorienting the relationship between operational systems and analytics. Only genuinely data-oriented companies will successfully pursue this path, and they will be the ones to reap its benefits in flexibility, depth of insight, and speed to market.
It’s time to drag ourselves into the 21st century.
Originally published on the author’s The Data Brains blog and reprinted with permission.