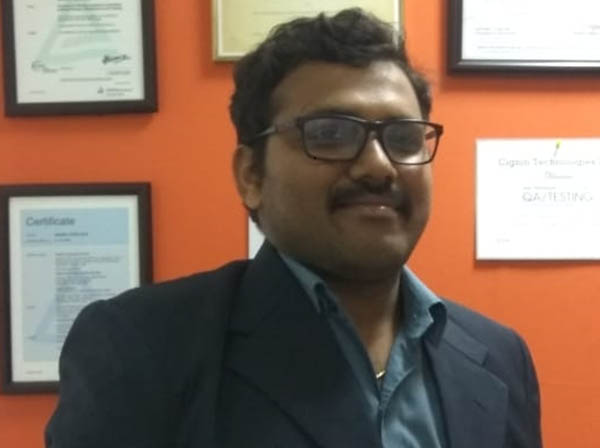
With organizations embracing digitization in a big way, the generation of data has grown manifold. According to IDC, the growth of data will be huge across industries, from 16 zettabytes to 160 zettabytes. The data being talked about is useful for businesses to draw insights, formulate strategies, and understand trends and customer behavior, among others. Even if collating, storing, analyzing, and working with such a sheer volume of data is challenging, it does not cover the whole picture. The fact that data is stored in silos and in the wrong format makes it a real challenge for enterprises to work with it, let alone make any sense of it.
As per the Big Data and AI ExecutiveSurvey 2021 by NewVantage Partners, enterprises continue to struggle to extract value from big data. Consequently, only 48.5% of enterprises drive innovation with data, and only 41.2% compete on analytics. Also, only 29.2% of them have made an impact on digital transformation using data, and only 24% are found to be data-driven. The growing demand for agile data analytics from huge data volumes has given rise to concepts such as an enterprise data lake.
What Is an Enterprise Data Lake?
Enterprises today face insurmountable challenges in accessing and managing an enormous volume of data available in multiple data types and formats and emanating from several touchpoints and third-party sources. As a result, business stakeholders often find it difficult to draw the right insights on time and make decisions. The demand, therefore, is to have a central repository of data and analytics solutions offering more agility and flexibility compared to a conventional data management system. An enterprise data lake is a data storehouse to hold huge volumes of raw data in native formats. According to Gartner, a data lake for enterprises is the storage of various data assets in the exact or near-exact format of the originating source. Thus, data lake services play an important role in supporting enterprise data architectures and uniting data physically.
What Are the Benefits of an Enterprise Data Lake?
Data lake implementation can deliver several benefits to enterprises:
- Data gets stored in a centralized repository accessible across departments
- Overcomes the limitations of legacy system architectures
- Derives insights for analytics
- Accelerates discovery and access to analytics
- Transforms data for business processes not possible at the source
- Ensures a standard for storing, governing, and retrieving data across multiple sources
Enterprises are adopting advanced data management approaches to take data across functions and processes and deliver value from them. However, an enterprise data lake can fall short in delivering results for enterprises if the bulk of the data is stored in its native form. In other words, businesses need to invest money to make the data ready for analysis. Earlier, enterprises linked BI tools to such repositories, giving way to a few challenges. These include reduced collaboration, high latency, and an inability to provide context for domains leveraging such data. Further, such storage solutions offer challenges to conducting self-service for inferring new insights.
How AI-Powered Search and Analytics Can Maximize ROI
Artificial Intelligence has become a critical technology in analyzing patterns in data and garnering suitable insights. Even though most enterprises are aware of the key role of AI in next-gen technologies, they are not fully prepared to take the leap of faith. Unless aided by AI, enterprises cannot deal with massive sets of data using human resources. This is due to the fact that it is really expensive to transform data lakes to optimize workflow and make accurate data-driven decisions using human resources. Artificial Intelligence, on the other hand, is optimal for performing valuable analyses and achieving business transformation. AI can help enterprise data lake engineering services transform data lakes to yield desired results.
Use of Metadata: At the outset, adding relevant metadata to optimize the data lake framework would help stakeholders to understand the origination of data (when and where). Thus, the more descriptive the metadata is, the more connections can be made by AI. For instance, if a data lake contains weather-related data gathered from a weather sensor, it is useful to know certain pieces of information. These may include the geographic location of the sensor, date range, the software version of the weather sensor, and the specific sensor function, like wind speed, among others. So, the more descriptive and correlated references are there in the metadata, the more it becomes easier for AI to analyze.
Data Cleansing and Profiling: AI can cleanse data and enhance its quality by performing interactive data analysis. It helps to onboard and curate quality information by scanning every field in the data lake repository. AI can analyze data to flag any problem area and suggest the way to address the same, be it related to structural issues, duplicate records, standardization, or missing fields. It allows the export and upload of data to source systems for addressing issues at the source.
Structuring Data Lakes: Data lakes are extremely flexible and scalable and accommodate all data types. These help enterprises to draw greater insights at scale, quickly and cost-effectively. However, given that some of the incoming data to the repository can lack context and organization, data lakes can lose their perceived value and appear to be dumping grounds. Artificial Intelligence-led solutions with self-managing and self-organizing capabilities can help enterprises to profile, clean, and attach new data to the existing structures. It prevents data lakes from becoming data swamps and keeps them organized for fast retrieval and analysis.
Conclusion
AI-powered search and analytics can identify trends and outliers from data lakes. However, data present in an enterprise data lake needs to be organized for better automation and to help AI make better decisions. It is only by using the right AI-powered platform that connections can be established between cloud-native data lakes for faster optimization and better ROI.