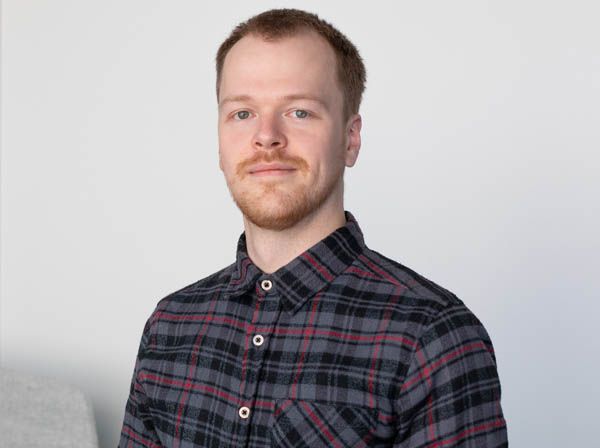
High-quality data can produce powerful insights that enhance decision-making. For data-driven organizations, this leads to successful marketing, improved operational efficiency, and easier management of compliance issues. However, unlocking the full potential of high-quality data requires effective Data Management practices. That’s why a robust data lineage strategy is essential – it helps increase information utility and maximize data value throughout your organization.
What Is Data Lineage?
Data lineage tracks the characteristics, transformations, processes, and relationships of different data elements. The practice comprises methods that map the data flow over time to clearly explain where the data originated and how it traveled through the system to its ultimate destination.
Benefits of a well-planned and executed data lineage strategy include:
- Increased transparency through detailed mapping
- Improved Data Quality through the identification of issues and errors that decrease accuracy and consistency
- System optimization by tracking errors to their root cause
- Enhanced compliance with regulatory requirements and data privacy laws
A robust data lineage strategy is one of the most powerful ways to maximize data value. The benefits to the organization’s systems are immediate in most cases and will flow out to reach every department in it.
Developing an Effective Data Lineage Strategy
Data lineage is established by capturing and visualizing information through various practices and applications that include manual documentation, metadata management systems, data mapping tools, and data flow diagrams.
Depending on your systems, the process can be time-consuming and complex. Here is a basic summary of the steps involved to give you a general idea:
- Identify data sources to include in the lineage: databases, files, applications, and other sources
- Document data flow from its source to the end destination by identifying systems, applications, and processes involved in collecting, processing, changing, and storing data
- Map data transformation by identifying any steps that changed the data, including data cleaning, normalization, aggregation, and other data hygiene processes
- Collect and record metadata to ensure correct data classification and optimized future use
- Establish governance through versioning, quality checks, and change management processes to ensure data is appropriately managed and maintained over time
A data lineage strategy also includes the following techniques to properly track, classify, and organize data sets:
Lineage by data tagging
Lineage by data tagging is a method of capture that associates metadata tags with the data as it flows through the system. Each piece of data is tagged by a transformation engine and read to determine and portray its lineage. Metadata tags typically include the data’s source, schema, format, and transformation rules.
This technique only recognizes data movements picked up by the transformation engine, making it suitable for closed data systems. Tools and applications used for this process include metadata management systems, data catalogs, and Data Governance frameworks.
Lineage by pattern
As the name implies, lineage by pattern uses patterns to generate or classify data instead of codes. The technique evaluates metadata for reports, tables, and columns, and analyzes them for similarities and patterns. Its primary advantage is that it only screens data, not data processing algorithms.
Another benefit of the lineage by pattern method is that it can be used consistently in the same way across all database technology, including Oracle, MySQL, and others. However, it’s sometimes inaccurate because the metadata doesn’t always reveal data connections. This typically occurs when data processing logic is not evident in the code and invisible in human-readable form.
However, in most cases, lineage by pattern is suitable when remote or inaccessible code prevents a thorough understanding of programming logic.
Lineage by parsing
Lineage by parsing is a method that automatically reads data processing logic to capture its transformation across systems. A significant benefit of the technique is that it provides total end-to-end traceability by reverse engineering data transformation logic. However, it can be challenging to set up because a thorough understanding of all the tools and programming languages used to transform and process the data is required.
Best Practices of an Effective Data Lineage Strategy
A successful data lineage strategy has numerous components and requires an extensive team of data architects and analysts, along with IT and legal professionals.
Here is a summary of some best practices to get you started:
- Identify business requirements to have a clear understanding of specific data types critical to business operations
- Develop a comprehensive data model to ensure all relevant data elements are captured in lineage documentation, including identifying all of the data sources, transformations, and outputs
- Use automated tools to produce documentation if possible, and leverage additional features such as data profiling and impact analysis
- Establish governance processes that ensure proper Data Management and maintenance over time, including versioning, quality checks, and change management processes
- Implement data security controls throughout the entire data lineage process to protect sensitive data and ensure it is used properly
- Regularly review your documentation so it remains accurate and up to date
It’s also important to consider that data is being generated constantly as processes and procedures in most organizations are increasingly digitized. As a result, a robust data lineage strategy requires constant refinement over time to adapt to evolving technical requirements.
How Data Lineage Practices Unlock the Potential of Dark Data
“Dark data” refers to unstructured data that is collected, processed, and stored but not used.
Examples include:
- Emails and attachments
- Former employee data
- Server log files
- Financial statements
- Sensor data
- Social media posts and website comments
- Customer call records
- Network device and application data
- Geolocation data
- CCTV camera data
According to a report by Gartner, dark data comprises roughly 55% to over 80% of total data. Since this information may contain critical insights or trends, dark data may prevent managers from obtaining an accurate picture of the organization.
Data lineage practices can solve this problem by identifying, tracking, and classifying dark data so it can be effectively utilized. A significant amount of dark data on inventory may prevent a business from deriving insights that could improve its catalog, shipping, and other product-related operations. Data lineage practices can help unlock this information to inform marketing decisions and optimize inventory processes.
Conclusion
The benefits of data lineage branch out to every area of the organization. Strong data utilization practices create massive value for the company through critical insights that optimize operations and enhance decision-making. An effective data lineage strategy can help tackle the challenge of dark data, improve security, and enhance compliance, which benefits the company’s clients, partners, and the entire business community.