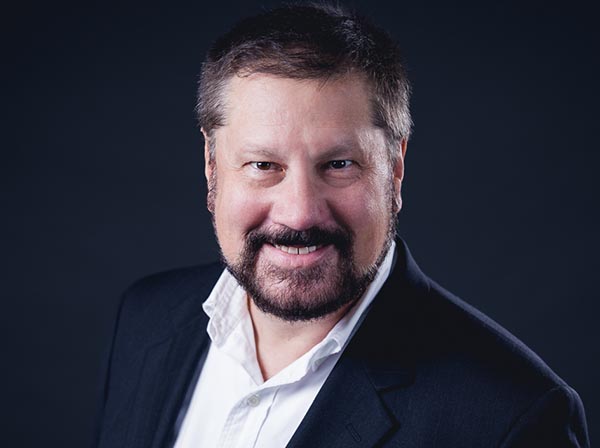
Click to learn more about author Frank Jablonski.
The challenge when implementing high availability (HA) in the cloud is the high cost. HA requires provisioning redundant resources to ensure being able to maintain desired performance levels during any failure. The resources in a standby instance are often configured to match the peak load of the active instance. Such 1:1 configurations are always able to satisfy stringent recovery time and recovery point objectives (RTOs and RPOs), but they can be difficult to cost-justify for all but the most mission-critical of applications.
Depending on the cloud service provider (CSP), the services used, and the contract terms, implementing HA in this way can double the cost of running applications in the cloud. To preserve the cost-saving potential afforded by the cloud’s enormous economies of scale, organizations may be tempted to implement inadequate HA provisions. After all, why pay so much for something that might be used for only a few hours a year?
All CSPs offer the ability to provision resources dynamically, but these capabilities were not designed for HA purposes. Such dynamic provisioning can be unreliable or employ time-consuming (and error-prone) manual processes, forcing cloud customers to either spend more than necessary or fall short of meeting service levels during periods of peak demand.
For these reasons, IT departments are looking for CSPs to help them operate more efficiently through machine learning (ML) and artificial intelligence (AI) technologies that are capable of delivering more effective and fully automated dynamic resource management. Achieving this will require the system to understand when it needs more resources and then automatically scaling up those resources to meet the increased demand. Conversely, the system will need to understand when certain resources are no longer needed and safely reduce their allocations to minimize costs.
ML and AI are ideal technologies for making HA provisions more cost-effective. The approach requires monitoring each application on a 24×365 basis to understand usage patterns throughout the year during different times of the days, weeks, and months. As those patterns are learned, the system will continuously improve its ability to adjust standby resource allocations to maximize savings without compromising protection.
To meet demanding RTOs, the CPU and memory resources allocated in the standby instance will need to be adjusted constantly to match those currently being consumed in the active instance. These are the most expensive resources and minimizing them when possible promises to deliver potential savings of roughly 50 percent over current practices. To meet demanding RPOs, data will still need to be replicated constantly, but data storage in the cloud is relatively inexpensive.
The ML/AI-based dynamic HA provisioning system will interface with the CSP’s ordinary provisioning capabilities through published APIs, enabling it to operate automatically. Since automation need not mean giving up control, these systems will offer graphical user interfaces (GUIs) for monitoring the actions being taken, and to enable operators to specify various options for different applications. The GUI should also clearly show the cost savings currently being achieved and may offer the ability to perform “what if” analyses to optimize the settings.