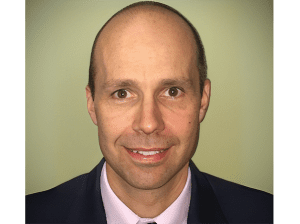
The life sciences industry has witnessed a surge in Data Management solution investments over the past few years. Despite these expenditures, however, these solutions all too often fail to keep pace with the latest technological advancements, leaving organizations struggling to address evolving pain points and integrate them with existing investments. Past Data Management systems, often lacking the adaptability necessary to continue to meet growing requirements or enterprise needs, quickly become outdated and therefore hinder innovation.
Unfortunately, due to the high cost of investment, many organizations feel compelled to continue using aging systems, negatively impacting the direction of future IT investments. This underscores the importance for life sciences companies to prioritize Data Management solutions designed with flexibility and adaptability in mind. Embracing this approach will not only accelerate business decisions but also enhance the value derived from data insights.
Traditional Challenges
Traditional Data Management models have emphasized customization, which, while beneficial in theory, often limits an organization’s ability to adapt and scale. In the dynamic world of life sciences, as organizations realize the downsides of this approach – increased complexity, rigidity, and scalability challenges – they are recognizing the value of flexibility in data management software, acknowledging its direct correlation with the overall value of information technology while exploring data management systems designed for fluidity and adaptability. Prioritizing a flexible Data Management system empowers life sciences organizations to swiftly address existing pain points and complement previous investments, ensuring that their Data Management infrastructure remains well-positioned for their evolving needs.
High Configuration, Low Code, and Automation Value
Historically, traditional software selection methods often involved licensing multiple solutions from different vendors and engaging consultants to deliver integrated Data Management systems, resulting in complex and costly implementations that few could effectively navigate. As mentioned earlier, the downside of customization is its heavy reliance on custom coding and associated high expenses. This approach not only demands significant time and investment in software development but also incurs substantial ongoing maintenance, update, and management overheads.
In contrast, systems that support low-code/high-configurability capabilities enable users to rapidly align Data Management processes to their specific requirements while minimizing time spent adapting to change and maximizing value realization. This includes the ability to automate and connect to legacy systems with ease. Highly scalable Data Management systems connect and integrate disparate data, allowing customers to get the most out of their software.
The value of software extends beyond low-code/high-configuration capabilities to address the automation of repetitive human tasks. For instance, defining configurations around a source system, such as integrating a multitude of objects, can be extremely time-consuming. Software that can automate such integration – including defining table names, attribute types, and formats – saves time and promotes cost-effectiveness. In addition to low-code and high-configuration software, automation plays a crucial role in accelerating integration processes.
Many organizations, particularly those of large size, complexity, and diverse nature, comprise multiple domains and workspaces. As a result, information technology budgets often compete for research and development and other essential areas. At some point, leaders must justify their spending and investments, demonstrating how these allocations can be applied across the entire enterprise.
Artificial Intelligence Use in Data Management
Most life sciences organizations manage vast amounts of data spread across internal and external servers. The ability to intelligently connect and gather this data is crucial for deriving meaningful intelligence – efficiently and accurately. Adding large language models (LLMs) and generative artificial intelligence (GenAI) to Data Management software can significantly improve data bridging, data warehousing, and data stewardship. This can not only speed up software updates but ultimately make better use of available data.
In data bridging scenarios, LLMs can generate language representations of data from various sources, facilitating seamless integration and analysis within a single platform. GenAI can further refine the process by correcting errors and filling in missing values, ensuring data accuracy and completeness. Data warehousing, a critical aspect of life sciences Data Management, involves handling large and complex datasets. GenAI and LLMs can automate data onboarding and connection, adding value at both the configurational and technical levels. This automation streamlines data warehousing processes, reducing manual intervention and improving overall efficiency.
Data stewardship, the practice of ensuring data quality, integrity, and accessibility, benefits immensely from GenAI and LLM integration. These technologies can automate data steering and discrepancy resolution, freeing up data stewards to focus on more strategic tasks.
The ability of GenAI to deliver software enhancements through code automation is a game-changer in data management. When companies require change, a data management system with GenAI capabilities can quickly adapt its functionality to address evolving needs. This adaptability ensures that the data management infrastructure is still aligned with the organization’s changing requirements. Elasticity, the ability to scale up or down based on demand, is another critical aspect of data management. GenAI and LLMs can dynamically adjust processes to accommodate fluctuating workloads, ensuring that the data management system remains efficient and cost-effective.
In Closing
By prioritizing flexibility in Data Management, companies will be able to drive greater insights out of their data, increase automation, and maximize software usage. Empowered by GenAI and LLMs, life sciences companies can foster a culture of connected intelligence across the organization. This connectedness enables better decision-making, increased innovation, and improved results for patients and other stakeholders.