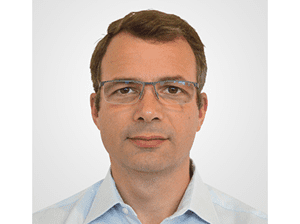
Among business leaders today there is a persistent fear of missing out on something transformative. They know they are sitting on a goldmine of data; they know this data could conceivably be turned to profitable ends. What they struggle to do is leverage this data. And the more time they waste, the worse the problem gets because their stores of data keep compounding.
For many years, this proliferating data was pooled in data lakes. Data was extracted from a variety of sources, transformed in line with a new data structure, and then loaded into a central data warehouse to be synthesized and analyzed for actionable insights. At a certain point, the quantity of data involved (and the sheer effort involved in extracting it) became untenable and the cutting-edge of data science shifted to data fabrics and data meshes.
Defined roughly: A data mesh stands for decentralized data ownership and management, while a data fabric is more centralized with a unified layer for data access. A data mesh is a set of principles, an implementation pattern; a data fabric is more of a knowledge construct. It might sound like these two concepts are opposed, but they aren’t. Most successful data operations will implement a hybrid approach, combining the principles of data fabric and a data mesh while – crucially – overlaying knowledge graph architectures to find essential information.
Unlocking the latent revenue potential of internal data means understanding precisely how these pieces fit together – and how to leverage their combined power to access transformative business insights.
Replicating the Facts, Not the Data
Right now, too many businesses are deploying an outmoded approach. In this approach, any time a new application is created, it needs to be connected to all existing data sources. Generally, this job falls to the developer, who then has to interpret all of those data sources, stay on top of their fluctuations, and keep data interpretations current. The problem is that developers weren’t trained for this: It is not their area of expertise and it creates the potential for real governance risk. This labor-intensive interconnection also serves to slow down the whole system, which will inevitably run at the pace of its slowest component.
A wiser approach – one that uses knowledge graph architectures in tandem with the data mesh and data fabric – is to replicate not the data, but the facts. Businesses should isolate only those data points that are useful in answering a given query. What they should be after is not the data itself, in other words, but a map of that data – because that is ultimately all you need to run the relevant analytics.
This is where knowledge graph architecture comes in. Overlaid on the data mesh-data fabric hybrid, it can provide an ontological and semantic map of your data, shaping it to useful and profit-driving ends.
Why Knowledge Graphs Matter
For an analogy, let’s turn to the pharmaceutical industry. Drug companies must be able to generate quick, comprehensive answers to any question they might have about a drug. The stakes are high, given the potential for patient harm and subsequent regulatory action or legal trouble. But current data paradigms make generating high-quality, actionable information about drugs a challenge.
Why? As it happens, it’s all in the name – or, more precisely, names. The lifecycle of a drug that makes it to market is long with countless distinct stages: research, trials, FDA certification, manufacturing, commercialization, post-approval monitoring, etc. At each of these stages, the drug in question might be filed under a different code name, or project number, or file number, or brand name. For instance, the drug might have five different names in five different companies, and 10 different names within a company as it is commercialized in different markets. Drugmakers need a 360-degree view of this data to make good decisions and avoid liability, but when the data they need to properly answer a query is (for instance) a layer above in their model, they are forced to operate off impartial and potentially misleading information.
This is where knowledge graphs come in – they allow you to combine disparate ontologies and look at your information through that overlapping lens. This matters for many reasons, not the least of which being that it broadens the range of people who can execute complicated queries: You no longer need to be fluent in best SQL practices to acquire important insights.
The revenue implications are significant here regardless of industry. For instance: manufacturers. Right now, many manufacturers are focusing less on acquiring new clients and more on optimizing revenue from existing ones. Proper deployment of knowledge graph architectures in this context can help flag things like improper discounts or the potential for CPI increases, handily increasing profit with a minimum of extra spend.
Overcoming Implementation Challenges: Never Lose Sight of Business Objectives
So, what’s the best approach for businesses who want to revamp their data operations along these lines?
Think in terms of data as opposed to applications, look for integrated solutions, and create a data-centric company culture.
That last point is important. Too often, data initiatives get bogged down in “plumbing” and effectively become IT projects disconnected from broader business goals.
I’ll illustrate this with a story – a composite, but one that is far from implausible.
A large healthcare provider decides it wants to investigate incidents of deaths in ambulances en route to the emergency room. The endless plumbing begins and after one year of incalculable effort, a team of 20 people manages to answer the question. They bring their answer to the higher-ups, who, nonplussed, ask them about the number of those same cases in which a paramedic was on board. The plumbing starts up again. Six months later, they return with their answer – and are asked, in how many cases was a defibrillator on board? Right back to the plumbing. Another six months, another question from the higher-ups: Was the paramedic on board trained to use the defibrillator? This time, no plumbing: The IT team simply gives up.
This project – and the many others like it that are undertaken every day – was led by developers as opposed to data scientists. It was not business-centric, nor did it deploy the kinds of knowledge graph architectures discussed above. What it points to is the importance of creating a proper data culture, of leveraging your data stewards, modeling your data correctly, and fighting with IT when business objectives are on the line.
The ultimate objective here is fluidity: You want to be able to rewash your data at any time in line with shifting business requirements, to answer new questions as quickly as possible without necessarily having to get IT involved. Internal data, leveraged properly, can be a fantastic source of growth and revenue, but only when you have a 360-degree view of your data. That is what the data mesh and the data fabric – brought alive through knowledge graph architectures – can offer.