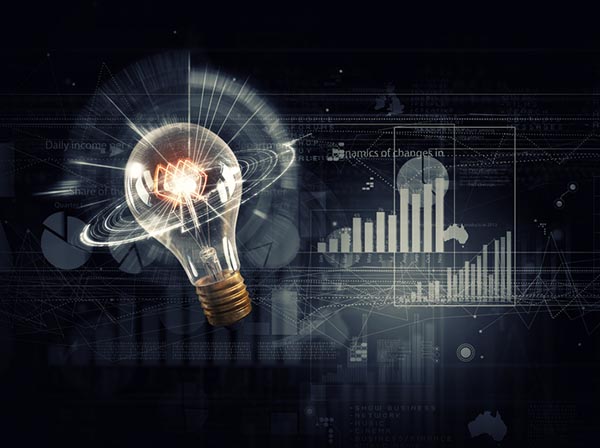
This past fall, all aspects of the computable knowledge structure KBpedia – its upper ontology (KKO), full knowledge graph, mappings to major leading knowledge bases, and 70 logical concept groupings called typologies – became open source. Making big strides in increasing definitions and mappings has been a main focus of KBpedia v. 1.60.
As it always has, KBpedia combines key aspects of Wikipedia, Wikidata, schema.org, DBpedia, GeoNames, OpenCyc, and UMBEL into an integrated whole and supplements these with twenty leading vocabulary mappings that bring in new knowledge bases and integrate with existing vocabularies, schema, and instance data to work within the KBpedia structure. There are 55,000 reference concepts in its guiding knowledge graph, which ties into an estimated 30 million entities, mostly from Wikidata.
“Besides all of the reasons we designed KBpedia in the first place, there is a huge demand to bring a workable structure that can leverage Wikidata and Wikipedia to provide users with a coherent means to search, retrieve, and organize their assets for their purposes,” says Mike Bergman, senior principal for Cognonto Corp. and lead editor for the KBpedia knowledge structure.
This may prove to be a major impetus for greater adoption of KBpedia. Wikidata and Wikipedia are the two principle resources that inform KBpedia, and what’s needed in both for users to manipulate the information in a coordinated way is to have a computable overlay atop them, says Bergman.
While that’s a clear use case, Bergman also hopes that by open sourcing KBpedia, “others may see its value in ways that we couldn’t, and I think our satisfaction will be to see new ideas and contributions blossom,” says Bergman.
For example, KBpedia has always helped support machine learning and knowledge-based artificial intelligence for the enterprise. With large-scale knowledge graphs, almost every node is an entry point or facet. Being computable, the KBpedia structure can be reasoned over and logically sliced-and-diced to produce training sets and reference standards for Machine Learning and data interoperability.
In the KBpedia open source announcement, it was noted that “tremendous strides have been made in the past decade in leveraging knowledge bases for artificial intelligence.” But, the announcement continues, limitations remain. One is relying on knowledge sources like Wikipedia that were never designed for AI or data integration purposes. The second problem is that no repeatable building blocks can be extended to any domain.
“AI is sexy and attractive, but way too expensive. We hope the current open source release of KBpedia moves us closer to overcoming these problems,” the release reads.
The facilitation of AI in KBpedia – capabilities that always existed but were never before open-sourced and freely available to everyone – comes by way of more than 300 features upon which users can train machine learning. These features include a rich pool of labels for doing supervised machine learning. Additionally, definitions, synsets to broaden semantic search, and robust text are available for nearly all of KBpedia’s 55,000 reference concepts. The organization of KBpedia into entities, events, concepts, attributes, and relations provides still further discriminatory power.
For supervised, semi-supervised, and distant supervised machine learning “you can present unknown inputs and get output labels that identify and categorize entities,” Bergman says.
“In supervised learning, a major cost is labeling outputs, and with properly and logically and consistently structured knowledge graphs, users can create those training labels and sets in minutes.”
In traditional approaches to supervised learning, the same tasks would consume 60 to 80 percent of the total effort required.
With unsupervised learning, users can create new functionality with the right kind of data sets – “the corpus of information that is appropriately bounded by manipulating the KBpedia knowledge structure,” Bergman says. In unsupervised learning, the outputs are not labeled in advance, and the trick to building a sound unsupervised learning structure is to create training corpuses to run algorithms against.
“You have to make sure that the algorithm for the input corpuses on which to learn is properly bounded to the problem you are trying to address. So, being able to finetune the scope and boundaries of corpuses is useful to get better unsupervised learning results as well,” he says.
Help for C-Level Data Execs
With the open-source KBpedia v. 1.60, Bergman is addressing the fact that many CIOs, CTOs, CDOs, or others who have responsibility for an organization’s knowledge resources want to learn more about what to do with them.
“They hear about things that are happening with machine learning, but they have all their internal data sets that don’t talk to one another and internal issues of having a coherent enterprise-wide view of their information,” he says. Those issues are addressed by the fact, “that KBpedia is a scaffolding for bringing together existing internal knowledge resources to overcome the ‘stovepipe’ problem. Second, once so organized, they can use the inherent structure of KBpedia to slice-and-dice their own training sets and training corpuses for supervised and unsupervised machine learning at greatly reduced costs.”
Bergman’s book, A Knowledge Representation Practionary, that aims to help organizations work through these issues. “There are ways to think about these problems and to approach them pragmatically and logically,” he says. “The building blocks are there and with open source they can manage their ways out of dead ends.”
This book on knowledge representation, like KBpedia itself, is based on the writings of Charles S. Peirce, a twentieth century logician, scientist, and philosopher. He provided practical guidelines and universal categories in a structured approach to knowledge representation that captures differences in events, entities, relations, attributes, types, and concepts. “This book is context and background of why we built KBpedia, as an attempt to reconstruct his theories about how to represent knowledge,” Bergman says.
This current release of KBpedia and the others to come immediately after it are meant to complete the ‘baseline’ of KBpedia, “The completion of the baseline means full mappings to all existing external sources (the seven constituent knowledge bases including Wikidata and Wikipedia) and full definitions for all concepts.” Once this baseline is complete, plans include mapping to other constituent knowledge bases. That could include the addition of things like product catalogs, language resources like WordNet, and other classifying systems, he says.
“We want other ways to create crosswalks to other high-quality knowledge bases,” Bergman says. “We want the baseline to be relatively complete and to have as much coverage as possible to external contributions.”
Image used under license from Shutterstock.com