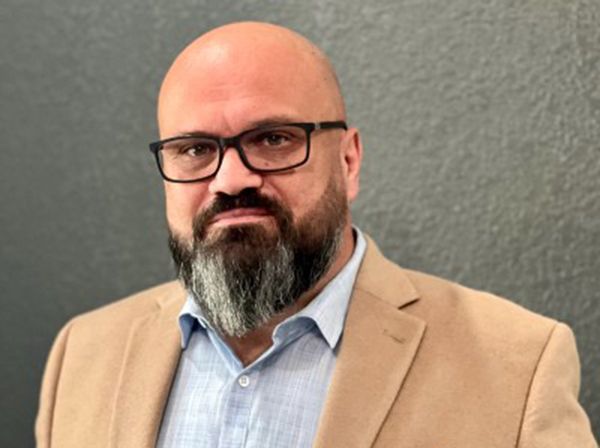
Generative AI (GenAI) is all the rage in the world today, thanks to the advent of tools like ChatGPT and DALL-E. To their credit, these innovations are extraordinary. They’ve put the power of artificial intelligence and machine learning (AI/ML) into the hands of everyday users. However, these tools have also skewed our perceptions of what is most important right now in the age of accessible AI/ML.
Generative AI is one subset of Data Science. There are other aspects of Data Science that businesses of all sizes can take advantage of. The hurdle that most companies will have to jump concerning Data Science is a fact-based hurdle, not a technical one. The fact is this: You cannot have a Data Science strategy if you do not have a data strategy. Too many leaders are putting the cart before the horse right now – they are investing in GenAI before having a clear understanding of how to unify, store, analyze, and apply data at scale. These fundamental capabilities are being overlooked, which will lead to challenges down the road when trying to create value with Data Science initiatives, including GenAI.
The answer to long-term success with AI/ML is to pursue data readiness. Data readiness for generative AI means putting the right processes and architecture in place to manage big data effectively. The great news for organizations is that pursuing data readiness for AI is valuable in and of itself. There is still significant opportunity to innovate, improve services, and drive growth with big data before introducing GenAI to the mix. What’s more, cloud service providers, like AWS, make this easier than ever today. Let’s see why.
How Do I Achieve Data Readiness for Generative AI?
Data readiness exists when the following two components live in harmony under a comprehensive data strategy:
- Data Architecture
- Data Engineering
Data Architecture refers to the tools and resources we use to get data into a state where it can be engineered for Data Science pursuits. Think of it this way: If data is the new oil, the well has to be dug and the derrick installed to get it out of the ground, consistently, efficiently, and dependably.
AWS offers a variety of tools for deploying data architectural patterns. These include data lakes, data ingestion pipelines, data warehouses, data marts, and data migration tools. The process involves designing and building a specific Data Architecture. This architecture unifies organizational data in a certain pattern. It provides a 360-degree view of the data needed to answer business questions. These are the questions that Data Science seeks to answer. If engineers don’t architect the data well for engineering activities, it can hamper progress. This applies to both unified, holistic organizational data and divided, distributed data. Both can equally obstruct progress in Data Science, including generative AI. Getting the Data Architecture right is the first step. It helps in drawing insights from the massive volumes of data available to organizations today.
Data Engineering is how we get data ready for the complex work that data scientists and machine learning engineers do. Think of it this way: If data is the new oil, the crude will have to be refined into usable “products.” Data engineering involves activities like data processing (e.g., cleaning, categorizing, labeling, etc.), data analytics, and data visualization. It also includes ETL jobs that move data into purpose-built data stores, such as data marts and data warehouses for downstream analysis, inclusive of data scientists. Data can be engineered for purpose in transit between data architecture components. Or it can be engineered in place depending on the nature of the component.
Data Science is the practice of using software development and statistics within a specific domain. This assumes that the data is properly prepared. Consider this analogy: If data is the new oil, it must be refined into usable “products.” Someone must then apply these products in the real world to maximize their value.
This is the role of data scientists and machine learning engineers. They structure data for consistent delivery and availability. Then, they prepare and analyze it to ensure it’s complete in engineering. They can use AI/ML managed services, Amazon SageMaker’s low code or no code tools, or custom models in SageMaker. These tools help derive insights from the data. These insights can answer business questions. If answered well, they can transform the business.
The Importance of Data Readiness and Strategic Implementation
The challenge is that many enterprises overlook the importance of building a basic yet effective data strategy as the roadmap for a healthy and sustained data science practice that can serve as the foundation for larger GenAI efforts. They hire data scientists and expect them to operate across all three of the areas above. This leads to mixed results or good results at poor velocities. This should only really happen in startups or small businesses with limited resources.
The ideal approach to leveraging GenAI is to invest thoroughly in Data Architecture, Data Engineering, and Data Science, which require a multitude of technologies and skills. Then, these lanes must come together under a well-defined data strategy. Companies that implement Generative AI without these components in place are building on top of a house of cards that can’t deliver real value.
Fortunately, the cloud makes it easy to achieve data readiness by offering solutions across the entire Data Management spectrum. These solutions are available as pay-as-you-go managed services, so companies don’t have to deal with the underlying IT infrastructure. Put simply, the cloud gives modern enterprises everything needed to unlock data readiness for GenAI. Of course, this is easier said than done.
Accomplishing this requires expertise in the bottom four layers of the Data Science Hierarchy of Needs, created by data scientist Monica Rogati. Good Data Architecture and Data Engineering services cover the following four layers of the pyramid: Collect, Move/Store, Explore/Transform, and Aggregate/Label.
Data Readiness Next Steps
If you’re hoping to leverage generative AI in your organization, make sure you’re ready. Build a robust Data Science practice first that’s based on sound Data Architecture and Data Engineering principles. Ensure your data is accessible and reliable for those who need it. And implement efficient data processing and analysis to uncover new insights for your AI projects.
Innovation in this space is happening at a dizzying pace. Rather than jump on the latest bandwagon, first start by building a sustainable data foundation that is ready for yesterday, today, and whatever tomorrow brings.