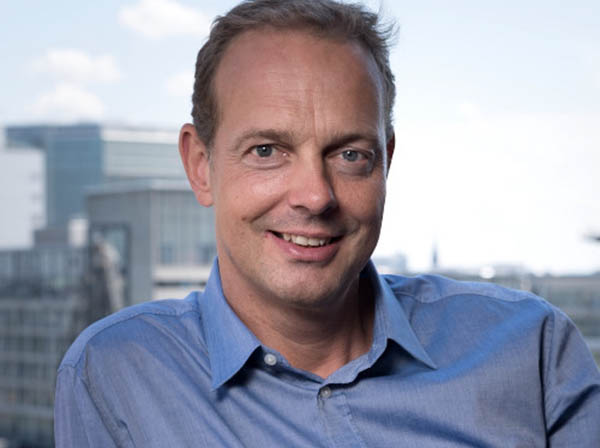
Click to learn more about author Bernd Gross.
In today’s digital business age, enterprises are rapidly moving applications to an event-driven model to achieve the speed, agility, and responsiveness they need to remain competitive. Digital businesses — and their supporting technologies — must become more adept at responding to changing circumstances, and they must do so quickly. At the same time, organizations are dealing with ever-increasing volumes of data from an ever-increasing number of data-generating sources.
Imagine how much faster organizations could make an impact if they could glean real-time insights instantly from all the high-volume, fast-moving big data streaming in from global markets, mobile devices, IoT sensors, internal transactional systems, and a myriad of other sources connected within the enterprise.
How Streaming Analytics Benefits Businesses
Streaming analytics has become an indispensable tool for companies to gain precise insights and operational intelligence from their ever-growing volumes of data. As the number of big data streams expands — and networks become increasingly more complex — high-performance streaming analytics enables enterprises to integrate and analyze large volumes of changing data from a wide number of concurrent applications.
With this real-time insight, streaming analytics allows organizations to maintain an overview of the integration and analysis of their data. In turn, this grants them the power to identify and act on future risks and opportunities, increase operational visibility, and make instant decisions at scale — positioning organizations for business success by allowing them to respond to important events immediately.
At a high level, streaming analytics delivers powerful insights across a wide variety of industry verticals, including:
- Retail: Streaming analytics can help marketers better understand a customer’s buying experience, allowing them to detect and take real-time action based on customer behavior. For example, marketers can personalize customer offers based on real-time location information and historical reference data to maximize offer uptake.
- Finance: Streaming analytics can help traders stay ahead of fast-moving foreign exchange markets. Credit card companies can also use streaming analytics to detect fraud across multiple channels and millions of transactions.
- Supply Chain: Streaming analytics can power omnichannel fulfillment, delivering greater order visibility, and helping to find products customers want across any channel in the distribution network. In addition, it can also alert an organization whenever something might not be going according to plan — such as delivery delays due to severe weather — allowing them to alert customers proactively.
Capabilities of Successful Streaming Analytics Platforms
Ultimately, the right streaming analytics tool provides organizations with the ability to react quickly to real-time data. In order to accomplish this, organizations should ensure that their streaming analytics platform is able to:
1. Accommodate many data formats and integrate with different event streams and app environments.
2. Recognize and analyze patterns in real-time.
3. Aggregate data and perform fast analytics, filtering, and correlating.
4. Enrich streaming data with context data for deeper, richer insights.
5. Design continuous real-time analytics scenarios.
6. Enable rich real-time visualization.
7. Support extreme scale and performance.
8. Be implemented in local, server, or cloud platforms.
9. Create business dashboards for end-users to monitor data streams.
10. Enable simple visualization of key performance indicators.
Oftentimes, sifting through big data to find the most valuable insights can feel like searching for a needle in a haystack. With the help of streaming analytics, businesses can put the data to work for them by making it easier for them to drive higher-quality business results through faster and more accurate data-backed decisions.