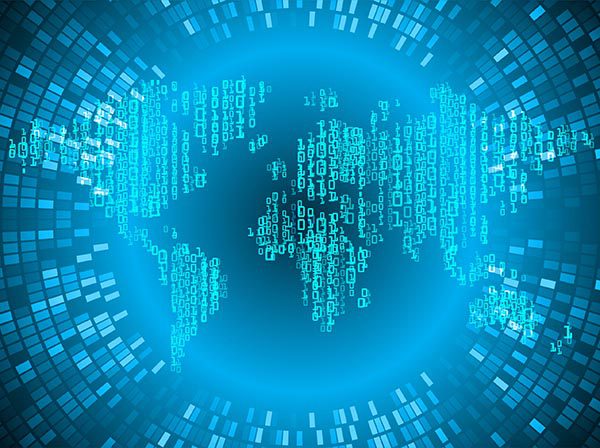
As the available industry literature suggests, Data Quality (DQ) is seriously hindering the success of many big data projects. However, it is apparent that without an organized movement in favor of Data Quality for big data, nothing will actually happen to change the current scenario.
In an interview with the CEO of Informatica, Anil Chakravarthy, reveals how the lack of DQ can inhibit innovation in information technology. The two major impediments to consistent DQ have been identified as high volume of data and inconsistent data elements, both of which could potentially restrict the power of business analytics.
Avoid Scandal: Don’t Let Your Data Maintenance Get Sloppy suggests it is easy to overlook the fact that data supports a number of key business activities. So, “sloppy data maintenance” involving security breaches and other risky business processes can lead to long-term reputation management issues for a company.
The above instances indicate that there is an urgent need to measure whether business data is meeting the key DQ criteria of accuracy, relevance, conformity, reliability, consistency, and uniqueness. Gartner has measured the “average financial impact” of bad data to be $15 million per year, which reaffirms how crucial Data Quality Management (DQM) is to global businesses.
How Does Poor Data Quality Impact Businesses?
According to Thomas C. Redman, noted DQ expert, “Data are of high quality if they are fit for their intended uses in operations, decision-making, analytics, and planning.” This means the data should be free of defects while being relevant, comprehensive, at the proper level of detail, and easy to interpret. Of course, to understand Data Quality we must define what makes high-quality data.
In his book Data Quality: Management and Technology, Mr. Redman, notes that “payroll record changes have a 1 percent error rate, billing records have a 2 percent to 7 percent error rate, and the error rate for credit records is as high as 30 percent.” This points to the fact the enterprise Data Governance (DG) frameworks are failing to meet the required performance benchmarks. This is serious business as it can increase regulatory risks, hinder decision-making, and reduce data security.
How Has Data Quality Impacted Big Data Projects?
Big data analytics is a key activity in a thriving industry because it can predict customer expectations, aid product design by analyzing popular tastes and references, enhance service facilities by studying shopping patterns, provide competitor intelligence, and tailor customer availability to influence decision-making.
The volume, variety, and velocity of big data create tremendous pressure on the “veracity” factor of business data, especially given the diverse data pipelines present in today’s average businesses. The big data lifecycle demands superior Data Management systems in place, which include DG and DQ at their focal point.
To drill down the impact of three V’s on big data management, “volume” of data impacts scalability, “velocity” affects data transmission, and “variety” impacts the operational consistency of data-management systems. Together, the three V’s have a high impact on the overall performance on any big data management platform.
One of the most critical challenges in big data quality is accounting for a variety of data sources, many of which fall outside the enterprise firewalls, which makes reliability of data a distant dream.
Also, the increased use of distributed computing environments like Hadoop for big data operations substantially increases security risks and threatens the smooth availability and reliability of data. Thus, it is not enough to have skilled data professionals to manage such projects; appropriate Data Governance frameworks with Data Quality checks and balances must also be present to ensure success. An IJKE report analyzes the crossover point between big data and data lifecycle.
Today’s big data lifecycles include clear DG blueprints, which can guarantee value to any big data management project from data preparation to actionable decision-making at scale. Moreover, the idea of a “context” for interpreting analytic results is crucial in big data projects. Big Data Context: Targeting Relevant Data that’s Fit for Purpose describes the correlation between high-quality data and “context” for big data.
Common Data Quality Issues for Big Data Projects
A starting point for measuring Data Quality can be the qualities of big data—volume, velocity, variety, veracity—supplemented with a fifth criterion of value, made up the baseline performance benchmarks. Interestingly, these baseline benchmarks actually contribute to the complexity of big data: variety such as structured, unstructured, or semi-structured increases the possibility of poor data; data channels such as streaming devices with high-volume and high-velocity data enhances the chances of corrupt data—and thus no single quality metric can work on such voluminous and multi-type data.
The easy availability of data today is both a boon and a barrier to Enterprise Data Management. On one hand, big data promises advanced analytics with actionable outcomes; on the other hand, data integrity and security are seriously threatened. The Data Quality program is an important step in implementing a practical DG framework as this single factor controls the outcomes of business analytics and decision-making.
Finally, DG also ensures full compliance and reduces risks. Data Quality Management: What You Need to Know explains how a strong Data Quality Management program aids the Data Governance framework within an enterprise.
How Big Data Challenges Data Quality Management
Another primary challenge that big data brings to Data Quality Management is ensuring data accuracy, without which, insights would be inaccurate. A Datapine post can serve as a comprehensive guide for setting up a solid Data Quality Management program.
Through an effective DQM, businesses can set up Data Quality measurement practices and QC techniques to ensure that only very high-quality data enters the analytics engine. At the center of any good DQM program is the set of “test cases” to measure data, which can tackle the poorest digital data.
Data Governance and Data Quality for Big Data: The Final Words
Consider the following industry statistics:
- A joint study by TMMData and the Digital Analytics Association reports that “nearly 40% of data professionals (37.5 percent) spend more than 20 hours per week accessing, blending, and preparing data rather than performing actual analysis.”
- The Global Data Management benchmark report by Experian Data Quality states that on an average, C-level executives report that “33 percent of their organizations’ data is inaccurate.”
What do the above indicate? Most business data are difficult to access and integrate. While self-service analytics platforms are on the rise, it is imperative that solid Data Governance and Data Quality programs together can alter the current state of affairs and instill confidence in data-centric activities like analytics and BI. What is Data Quality Management? shares an BMC author’s view of DQM.
Newer technologies, one of which is certainly big data, have increased the data security and privacy concerns of big, medium, and small service providers dealing with these technologies.
Image used under license from Shutterstock.com