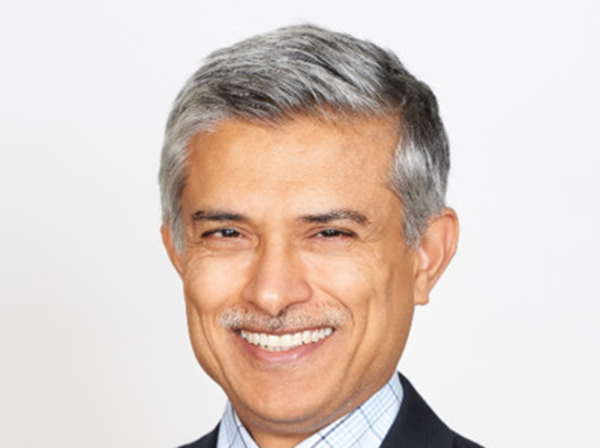
Click to learn more about author Deepak Dube.
Gartner reports have popularized the term “augmented intelligence” in the past couple of years. In this article, I will explain what it entails.
Let’s begin with how Gartner defines it: “Augmented intelligence is a design pattern for a human-centered partnership model of people and artificial intelligence (AI) working together to enhance cognitive performance, including learning, decision making and new experiences.”
First of all, what strikes me about the definition is that it’s a collaborative endeavor – dispelling any speculation about AI taking over human cognitive abilities or decision-making – and reinforces the notion of AI playing an assistive role in our lives. At its core, augmented intelligence is about empowerment: amplifying human intelligence with further insights, mined from data, in an automated, actionable, and timely manner, which otherwise would have taken much longer to discover by manual processes (or, worse yet, would have remained under wraps, well past the deadline for decision-making).
We all strive for betterment, and because of that innate drive, we end up collecting data. We all have it – plenty of it – and buried in that data are the insights to many questions that we seek answers for. After all, the data reflects our past decisions and outcomes as a result of those. This data is indeed about what worked and what did not – if only someone could explain in terms of simple rules and boundaries that we humans understand so well. The fact is, we are sitting on a gold mine. The question is, how do you mine that gold (the insights) in a timely and actionable manner, before it’s too late?
The discovery of these human-understandable rules and boundaries is more like reverse-engineering our code of methods, operations, and the systemic environment, be it in business or research. By the way, that’s not the only challenge. More often than not, in real-life use cases, the volume and dimensionality of the data presents another daunting challenge: There are so many features (dimensions) and many thorny questions, such as which features (factors or predictors) play a role and in what ways, what’s the interplay among these many features, and so on.
That is where augmented intelligence comes to our rescue, striking both challenges simultaneously by applying sophisticated techniques from AI and machine learning. Augmented intelligence extracts the intelligence – the insights about a systemic environment – from the entire data set, which helps improve decision-making. In education, for example, augmented intelligence might analyze a learning platform’s data dump and find that students on mobile platforms tend to have a poor experience compared to those on desktops in lengthy courses that have open-book exams.
Now that we have insights – presented to us in terms of simple, human-understandable rules and boundaries – we question the objective veracity of these insights. That’s OK. We are, by nature, inquisitive. It’s important for platforms offering augmented intelligence to instill that confidence by offering an objective measure of “goodness of insights (or reasons),” aka a confidence score. The platform/tool must associate a confidence score with each insight; you only select those insights that have a confidence score above your acceptable threshold. You verify these results against the data, by applying those human-understandable rules to filter the data and carefully study whether the results were correct, thereby building trust in the machine. That’s the beginning of true man-to-machine collaboration, or “trust.”
In conclusion, augmented intelligence plays the assistive role of a guide, enhancing your intelligence, helping you navigate through the maze of complex data, offering you comprehensive almost-instant insights and objective scores, and paving the path for your next steps. Onward to betterment.